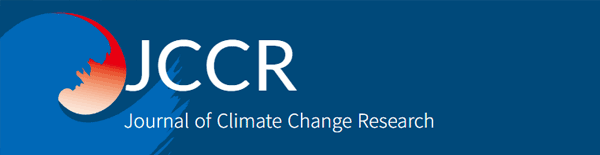
Econometric Estimation of the Climate Change Policy Effect in the U.S. Transportation Sector
Abstract
Over the past centuries, industrialization in developed and developing countries has had a negative impact on global warming, releasing CO2 emissions into the Earth’s atmosphere. In recent years, the transportation sector, which emits one-third of total CO2 emissions in the United States, has adapted by implementing a climate change action plan to reduce CO2 emissions. Having an environmental policy might be an essential factor in mitigating the man-made global warming threats to protect public health and the coexistent needs of current and future generations; however, to my best knowledge, no research has been conducted in such a context with appropriate statistical validation process to evaluate the effects of climate change policy on CO2 emission reduction in recent years in the U.S. transportation. The empirical findings using an entity fixed-effects model with valid statistical tests show the positive effects of climate change policy on CO2 emission reduction in a state. With all the 49 states joining the climate change action plans, the U.S. transportation sector is expected to reduce its CO2 emissions by 20.2 MMT per year, and for the next 10 years, the cumulated CO2 emission reduction is projected to reach 202.3 MMT, which is almost equivalent to the CO2 emissions from the transportation sector produced in 2012 by California, the largest CO2 emission state in the nation.
Keywords:
Climate Change Policy, CO2, Entity Fixed-Effects Model, Heteroskedasticity and Autocorrelation- Consistent (HAC) Standard Errors, Transportation1. INTRODUCTION
The effects of global warming have been observed around the world in the form of more frequent wildfires, longer droughts, and stronger tropical storms, which can have negative impacts on the Earth’s societal, ecological, and environmental systems (The National Aeronautics and Space Administration, 2014). Carbondioxide (CO2) emissions contribute to global warming through the greenhousegas (GHG) effect by holding heat energy from the sun in the Earth’s atmosphere, producing increases in global average temperatures (The U.S. Environmental Protection Agency, 2015). The Intergovernmental Panel on Climate Change (2014) reported that CO2 accounted for 57% of total global GHG emissions in 2004 and was the largest single source of emissions. They also reported that CO2 emissions produced by the transportation sector accounted for as much as 13% of total global GHG emissions from the combustion of fossil fuel1).
Among the five economic sectors in the U.S. (electricity, transportation, industry, commercial and residential, and other), CO2 emissions from the transportation sector ranked second highest, explaining 32% of total U.S. GHG emissions in 2012 (The U.S. Environmental Protection Agency, 2013). Although CO2 emissions from the transportation sector have increased, they reached a peak in 2007 (Fig. 1). Regarding the reduction after 2007, Choi et al. (2014) and Choi and Roberts (2015) suggested a couple of possible factors: 1) a government policy change (stricter federal regulations for GHG emissions) towards CO2 emission reduction, and 2) a decline in fuel consumption caused by higher oil prices led to increases fuel economy, production from alternative sources, and fewer miles travelled by light duty vehicles2).
For the last two decades, increasing interest in reducing CO2 emissions has generated numerous studies of the major CO2 emitting sectors (e.g., power, industry, transportation, etc.) mainly using the Laspeyres or the Divisia methods to attribute the growth in CO2 emissions to the potential driving forces. However, those methods are limited by their inability to consider the effects of qualitative factors, such as a change in environmental policy, while leaving to speculate the effects of an environmental policy change on CO2 emissions. In contrast, other researchers have incorporated an environmental policy factor while studying CO2 emission changes in passenger transport, freight transport, transport land use, and highway accidents when focusing on forecasting CO2 emissions, the effects of an environmental policy change, or revealing the relationship between CO2 emissions and their potential driving factors (Diakoulaki et al., 2007; Al-Ghandoor et al., 2009; Choi et al., 2010; Chung et al., 2013; Barido and Marshall, 2014; Kim et al., 2016; Park et al., 2016; Zhang et al., 2016).
Even though quantitative and qualitative analyses of CO2 emissions have been performed, to the best of my knowledge no research has been conducted with statistical validation process since 2007 to evaluate the effects of climate change policy on CO2 emissions produced by the U.S. transportation sector. Thus, the objectives of this study are to: I) identify whether climate change policies can reduce CO2 emissions from the U.S. transportation sector with appropriate statistical validation tools; ii) quantify the effects of U.S. climate change policy on CO2 emissions in the 49 contiguous U.S. states and for the nation 3); and iii) determine whether the effects of U.S. climate change policy are consistent states. The findings of this study will be helpful to state and federal policy planners in evaluating the effects of climate change policy within their boundaries and nation wide and will provide a base on which to improve and accelerate the trend of CO2 emission reduction through the implementation of stricter environmental regulations all over the world.
2. BACKGROUND
2.1 Study Area
The World Bank (2015) defines the U.S. as a high-income country among the Organization for Economic Cooperation and Development members, with gross national income per capita and total population of $53,470 and 319 million in 2014, respectively. In contrast, the U.S. transportation sector in 2011 emitted the largest CO2 emissions of 1,629 million metrictons (MMT) in the world, which was even higher than the summation of the European Union and China, the CO2 emissions of which were 1,535 MMT (=897+638) (The World Bank, 2015).
The CO2 emissions from the U.S. transportation sector during the study period of 2007∼2012 vary by state, but show three kinds of clusters, as presented in Table 1. The first one is the major CO2 emission group, which includes California, Texas, and Florida, the CO2 emissions of which contributed almost one-third of the total U.S. transportation CO2 emissions, and if the next 6l argest states (Florida, NewYork, Illinois, Pennsylvania, Ohio, Georgia, and NewJersey) are added, then they would account for almost 50% of the total U.S. transportation CO2 emissions. Second, the total CO2 emissions of the other next largest CO2 emission states, from Virginia to Kansas in Table 1, showing less than 3% but above 1% CO2 emissions in the total CO2 emissions, account for the other 40% of the total CO2 emissions; for this reason, they were classified with the middle CO2 emission group. Third, forming the minor CO2 emission group, the rest of the states emitted less than 1% of the CO2 emissions, occupying the bottom 10% of CO2 emissions. Fig. 2 provides a geographic description of each group by state.

CO2 emissions by state, percentage and cumulative percentage of the total CO2 emissions from the U.S. transportation sector in 2012, and group classification
2.2 Air Pollution Regulations including a Climate Change Action Plan
The first federal act regarding air pollution was initiated with the Air Pollution Control Act of 1955 to protect public health from disease caused by air pollution. Congress enacted the Clean Air Act in 1970 to establish national air quality standards and made major revisions in 1977 and 1990. Since the 2000s, with the Energy Policy Act in 2005, Energy Independence and Security Act in 2007, and presidential proclamations for CO2 emission reduction in recent years, the USEPA has established stricter air pollution regulations.
For the state-level climate change policy, Table 2 shows when the GHG inventory and/or climate change action plan was completed in each state. Of the 49 U.S. states, 31 (29) have completed a climate change action plan (GHG inventory) within their boundaries. A climate change action plan contains specific policy recommendations to address and reduce GHG emissions in various sectors, including transportation. Transportation-related emission reduction strategies are as follows: Low carbon fuel standard, trip reduction programs, heavy-duty vehicle anti-idling measures, clean vehicle purchase incentives, vehicle emissions standards, pay as you drive insurance, or others (Morrow et al., 2010; Choi and Roberts, 2014).
3. DATA AND METHODOLOGY
Various independent variables4) in the literature were taken into account to explain the factors of CO2 emission reduction in the transportation sector; therefore, the seven in dependent variables, including the implementation of a climate change policy, were all initially examined by a stepwise regression even though the effects of such apolicy are of primary interest in this study. The stepwise regression automatically selected the most important in dependent variables for modeling the mean dependent variable based on a t-test, and the brief procedures used are explained as follows: 1) all possible onevariable models of a linear form were fitted; 2) the best twovariable model of the form was selected through there maining (k—1), where k is the number of independent variables; and 3) the researchers checked for the third independent variable and this process continued until further independent variables did not appear to contribute to a significant p-value with the variables already in the model (Mendenhall and Sincich, 2011).
After the procedures, the four independent variables (fuel consumption, regulation of CO2 emissions, VMT, and GDP in the transportation sector, ordered by the lower p-value and statistically significant at the 10% level) remained. However, due to the high possibility of multicollinearity between the four variables, the variance inflation factor (VIF) was tested for each variable. Excluding the regulation of CO2 emissions, the three variables were highly correlated, showing a VIF greater than 10 (Mendenhall and Sincich, 2011); thus, only the variable of fuel consumption, which is directly connected to CO2 emissions, remained and the others were dropped. Finally, the two independent variables (fuel consumption and regulation of CO2) were selected. The former variable was obtained from the online data base of the U.S. Energy Information Administration (2015) and was measured in billion Btu. The latter variable was produced through a qualitative variable technique based on information regarding a climate change action planfrom Table 2. For the dependent variable, CO2 emissions were derived from the USEPA and measured in MMT (The U.S. Environmental Protection Agency, 2014). All the data ranged from 2007 to 2012 by state.
An entity fixed-effects panel regression model was utilized to estimate the effects of climate change policy on CO2 emission reduction in the U.S. transportation sector. The empirical econometric model for the pooled data and each group is as follows (Pindyck and Rubinfeld, 1997; Stock and Watson, 2011):
(1) |
where, β0, β1, β2, y3, …, yn+1 are unknown coefficients; X1it is the implementation of a climate change policy by state i in year t; X2it is the transportation sector fuel consumption by state i in year t; D2i is a qualitative variable that equals 1 when i = 2 and 0 otherwise; D3i is a qualitative variable that equals 1 when i = 3 and 0 otherwise, and so on; uit and is an error term.
n—1 qualitative variables were used to avoid the dummy variable trap by omitting the first qualitative variable, D1i. The rest of the qualitative variables take care of the effects of all the omitted variables that are not the same from each state to another but are constant over time. To summarize, the processes of the model performed in this study are as follows. First, the author extracted the appropriate regressors for the model by the stepwise regression and multicollinearity test. Second, the author tested whether the entity fixed-effects regression model is the best among other possible panel models, such as the time fixed effects, one-way random effects, and two-way random effects, by using F statistics and comparing the overall model fit and the estimated coefficients’ statistical significance. Third, a couple of statistical tests for verifying the serial correlation and heteroskedasticity were processed by the Durbin-Watson test and the Breusch-Pagan test, and the problems found were fixed by heteroskedasticity and autocorrelationconsistent (HAC) standard errors. Fourth, the author regressed the panel model and then analyzed the regression results based on the estimated coefficients’ p-values at the 10%, 5%, and 1% significance levels.
4. EMPIRICAL RESULTS
The empirical findings regarding the effects of climate change policy on CO2 emission reduction in the U.S. transportation sector from 2007 to 2012 are summarized in two partspooled data and each group-in Table 3 and Table 4. In both tables, the F statistic’s null hypothesis, which is no fixed effects, was rejected at the 1% level of significance. A couple of OLS regression assumptions were tested and the test results are shown with the existence of positive serial correlation and heteroskedasticity since the Durbin-Watson tests were less than 2 and the null hypothesis of homoskedasticity in the Breusch-Pagan tests were rejected at the 5% significant level, respectively.
To address these problems, HAC standard errors were used in the entity fixed-effects models. The HAC standard errors functioned to make the regression errors remain randomly correlated within a grouping and uncorrelated across groups (Stock and Watson, 2011). Additionally, to consider the potential omitted variable bias arising from omitting both technological advancements in fuel efficiency and the U.S. economic recession, the two control variables5) were utilized in the regression models. The concept of a control variable replaced the first least squares assumption of exogeneity with conditional mean in dependence6) to prevent the estimated causal effect of interest from suffering from endogeneity (Wooldridge, 2012). As Stock and Watson (2011) explained in 2011, this study distinguished between regressors for which we have an interest in estimating a causal effect and a control variable. By establishing the alternative assumption, “the OLS estimator of the effect of interest was unbiased, but the OLS coefficients on control variables were in general biased and did not have a causal interpretation” (Stock and Watson, 2011, p. 231).
In Table 3, an overall effect of a climate change action plan on CO2 emission reduction in the U.S. transportation sector across the 49 U.S. states was estimated and its coefficient shows a negative value of —0.413. If a state’s policy plan of CO2 emission reduction took effect during the period of 2007∼2012, then it on average has reduced the CO2 emissions per year by 0.413 MMT. Since the 31 states have completed such a CO2 emission reduction policy plan through a climate change action plan, their total reduced CO2 emissions reached 12.803 MMT in 2012, which was bigger than the entire transportation CO2 emissions of West Virginia in 2012, 11.75 MMT. As the author expected, the fuel consumption in the transportation sector has had a positive impact on CO2 emissions, technically 1 billion Btu in fuel consumption by transport in a state per year on average, increasing the CO2 emissions by 0.000077 MMT.
Table 4 provides the effects of the climate change policy on CO2 emission reduction in the three groups. Overall, the stability of average and standard deviation of CO2 emission by each group would not seem to be deteriorated when considering the difference of the levels of CO2 emission. The major group shows the most significant CO2 emission reduction through the climate change action plan, followed by the middle and minor groups. The policy plan of CO2 emission reduction in a state in the major group on average has almost three times as powerful a CO2 emission reduction effect than that of the middle group, while a state in the minor group has on average only one-tenth of the CO2 emission reduction effect compared with the major group. Since a climate change action plan exerts different effects within the groups, if the time and financial resources available are limited in the nation, then it is recommend to focus on letting the federal government first support the states in the major and middle groups7) rather than the minor group.
5. CONCLUSIONS
The human activities for industrialization have been accelerating and raising the global temperature for centuries, due to the increasing CO2 emissions released into the atmosphere by most of the economic sectors in a nation. As a result, we are unexpectedly experiencing severe natural disasters in many areas worldwide more frequently than in the past and thereby more people are being exposed to danger to their life and property.
A positive environmental policy change will be an essential factor in protecting public health and the coexistent needs of current and future generations; as Benjamin Franklin (Sussman, 2006) emphasized in 1963 at the University of North Dakota, the environment is an important public policy concern. In the U.S., such a change to relax the current high-level global warming and reverse it to a moderate level of around 1990 has been evident in various social and economic sectors; among them, the transportation sector, which emits one-third of the total CO2 emissions in the U.S., has been quickly adapting by implementing a climate change action plan to reduce its CO2 emissions.
This study revealed how effectively a climate change policy can function in the U.S. transportation sector by estimating the quantified climate change policy impacts not only for the national level, but also for the major, middle, and minor CO2 emission groups. The empirical findings using an entity fixedeffects model with various valid statistical tests shows an evidently positive effect of the existence of a climate change policy in a state on decreasing its CO2 emissions, even in the case of the minor CO2 emission group. If all of the 49 states can implement climate change action plans, the U.S. transportation sector can reduce its CO2 emissions by 20.2 MMT per year, and for the next 10 years, the cumulated CO2 emission reduction will reach 202.3 MMT, which is almost equivalent to the CO2 emissions produced by the transportation sector in 2012 in California, the largest CO2 emitting state in the nation. All of this finally suggests the importance of implementing a climate change policy to reduce CO2 emissions in the transportation sector.
6. STUDY LIMITATION
The author tried to find out scientific proofs regarding implementing effective climate change policies in the transportation sector as using an econometric tool which was well known in the econometrics. We probably think that decrease of fuel consumption will step out of CO2 emissions in the air more and more, but regardless of intuitive awareness, evident proofs need to be revealed. In this study, the author macroscopically found out the CO2 reduction effect of climate change policies, but in the next study microscopic effects of each climate change policy needs to be reviewed for the detailed policy effect analysis.
Acknowledgments
This study was supported by the Korea Research Institute for Human Settlements basic research. The contents are sole responsibility of the author. The author would like to thank colleagues in the institute and anonymous reviewers for their constructive comments.
References
-
Al-Ghandoor, A, Jaber, JO, Samhouri, M, Al-Hinti, I, (2009), Analysis of aggregate electricity intensity change of the Jordanian industrial sector using decomposition technique, International Journal of Energy Research, 33, p255-266.
[https://doi.org/10.1002/er.1466]
- Barido, DP, Marshall, JD, (2014), Relationship between urbanization and CO2 emissions depends on income level and policy, Policy Analysis, 48, p3632-3649.
- Choi, M, Kim, J, Lee, HJ, Jang, YK, (2010), Estimation of greenhouse gas emission from off-road transportation, Journal of Climate Change Research, 1, p211-217.
- Choi, J, Roberts, DC, (2015), does the change of carbon dioxide emissions change affect transportation productivity? A case study of the U.S. transportation sector from 2002 to 2011, Open Journal of Social Science, 3, p96-106.
- Choi, J, Roberts, DC, Lee, E, (2014), Forecast of CO2 emissions from the U.S. transportation sector: Estimation from a double exponential smoothing model, Journal of Transportation Research Forum, 53, p63-81.
- Chung, Y, Cho, H, Choi, K, (2013), Impacts of freeway accidents on CO2 emissions: A case study for Orange County, California, US. Transportation Research Part D, 24, p120-126.
-
Diakoulaki, D, Mavrotas, G, Orkopoulos, D, Papayannakis, L, (2006), A bottom-up decomposition analysis of energyrelated CO2 emissions in Greece, Energy, 31, p2638-2651.
[https://doi.org/10.1016/j.energy.2005.11.024]
- Intergovernmental Panel on Climate Change, (2014), Climate Change 2014: Impacts, adaptation, and vulnerability, Retrieved, January 5, 2015, from http://www.ipcc.ch/report/ar5/wg2/.
- Kim, M, Yoon, YJ, Han, J, Lee, HS, Jeon, EC, (2016), Analysis of GHG reduction potential on road transportation sector using the LEAP model, Journal of Climate Change Research, 7, p85-93.
- Mendenhall, W, Sincich, T, (2011), A second course in statistics: Regression analysis, Pearson, London.
- Morrow, WR, Gallagher, KS, Collantes, G, Lee, H, (2010), Analysis of policies to reduce oil consumption and greenhouse-gas emissions from the US transportation sector, Energy Policy, 38, p1305-1320.
- Pindyck, RS, Rubinfeld, DL, (1997), Econometric models and economic forecasts, McGraw-Hill, New York.
- Park, YS, Lim, SH, Egilmez, G, Szmerekovsky, J, (2016), Environmental efficiency assessment of US transport sector: A slack-based data environment analysis approach, Transportation Research Part D: Transport and Environment.
- Stock, JH, Watson, MW, (2011), Introduction to econometrics, Addison-Wesley, Boston.
- Sussman, G, (2006), The Environment as an important public policy Issue, Retrieved, January 9, 2015, from http://ww2.odu.edu/ao/instadv/quest/Environment.html.
- The National Aeronautics and Space Administration, (2014), The current and future consequences of global change, Retrieved, January 5, 2015, from http://climate.nasa.gov/effects/.
- The United States Energy Information Administration, (2013), Alternative Fuel Vehicle Data, Retrieved, January 7, 2015, from http://www.eia.gov/renewable/afv/users.cfm#tabs_charts-2.
- The United States Environmental Protection Agency, (2013), Global greenhouse gas emissions data, Retrieved, January 5, 2015, from http://www.epa.gov/climatechange/ghgemissions/global.html.
- The United States Environmental Protection Agency, (2014), Greenhouse gas inventory data explorer, Retrieved, January 3, 2015, from http://www.epa.gov/climatechange/ghgemissions/inventoryexplorer/#transportation/allgas/source/all.
- The United States Environmental Protection Agency, (2015), Climate change & air quality, Retrieved, January 5, 2015, from http://www.epa.gov/airquality/airtrends/2011/report/climatechange.pdf.
- The World Bank, (2015), CO2 emissions from transport, Retrieved, January 6, 2015, from http://data.worldbank.org/indicator/EN.CO2.TRAN.MT/countries?display=graph.
- Wooldridge, JM, (2012), Introductory econometrics: A modern approach, Cengage Learning, Boston.
-
Zhang, H, Chen, W, Huang, W, (2016), TIMES modelling of transport sector in China and USA: Comparisons from a decarbonization perspective, Apllied Energy, 162, p1505-1514.
[https://doi.org/10.1016/j.apenergy.2015.08.124]