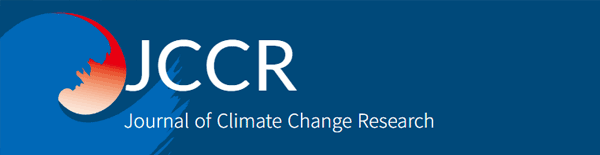
산포능력을 고려한 기후변화 생물지표종의 미래 분포 변화 예측
Abstract
This study assessed the climate change effect on and vulnerability of the three Climate-sensitive Biological Indicator Species (CBIS) Abies koreana E.H.Wilson, Aucuba japonica Thunb, and Camellia japonica L.. For this, True Skill Statistic (TSS)-weighted ensemble models were constructed using present/absent data of species, obtained from previous studies, and six environmental variables (three temperature variables and three precipitation variables). The future potential habitats of the species in 2050 (2041~2060) were predicted using the TSS-weighted ensemble models and the ensemble of 20 future climate projections produced by five Global Circulation Models (GCMs) based on four Representative Concentration Pathways (RCP) scenarios. The future potential habitats and climatic habitat suitability for three species were predicted considering reproduction age and seed dispersal function. The potential habitats of Abies koreana were on Mt. Halla in Jeju Island and the central and southern subalpine regions of the Korean Peninsula under the current climate. Those of Aucuba Japonica were the southern coastal and insular areas, and Camellia Japonica was present on the southern inland and central insular areas. The future habitats of all three species would expand under climate change. However, while the habitat suitability for Abies Koreana increased at all of its potential habitats, those of Acuba japonica and Camellia japonica decreased. Compared with the predicted areas without consideration of dispersal capacity, the future distribution areas of all three species decreased when considering dispersal processes, dispersal distance, and seed production processes. This study shows that dispersal processes are critical to understand the climate change effects on potential habitats of CBIS, which is fundamental knowledge for conservation planning.
Keywords:
Climate-sensitive Biological Indicator Species (CBIS), Climate change, Species distribution model (SDM), Seed dispersal1. 서 론
2018년에 IPCC에서 발표한 지구온난화 1.5도 특별보고서에서는 2006~2015년의 10년 평균 지구표면온도가 1850~1900년의 평균온도보다 0.87°C 상승하였고, 이에 따라 아한대림, 고산생태계 등 다양한 생태계가 훼손 또는 파괴 되었다고 평가하였다 (Intergovernmental Panel on Climate Change 2018). 특히 기후변화와 인간에 의한 생태계 파괴는 급격하고 지속적인 생물다양성 감소를 초래해 왔다 (Butchart et al. 2010). 제4차 지구생물다양성 전망 보고서 (Global Biodiversity Outlook 4)에서는 인류가 생물다양성 보전을 위해 노력하지 않는다면 생물다양성의 지속적인 감소로 인해 식량 공급 등 다양한 생태계서비스에 심각한 위협이 있을 것으로 전망하였다 (Secretariat of the Convention on Biological Diversity 2014).
21세기 이전 기후변화의 속도는 대부분의 생물 이동속도를 큰 폭으로 추월하지 않았으며, 일부 이동하지 못한 종은 유존종의 형태로 고산 및 제한된 서식지에 서식할 수 있었다 (Scherrer and Körner 2010; Dobrowski 2011; Gavin et al. 2014; Patsiou et al. 2014; Gentili et al. 2015). 그러나 21세기에 나타나고 있는 기후변화는 이전과 달리 변화의 폭과 속도가 크고 빠른 것으로 보고되었다 (Intergovernmental Panel on Climate Change and Edenhofer 2014). 또한 21세기의 인구 증가와 생태계 훼손은 기존에 경험할 수 없는 다양한 서식지의 파편화를 야기하였고 이로 인해 생물의 이동속도를 감소시키는 결과를 초래했다 (Pitelka et al. 1997; Etterson and Shaw 2001; Engler and Guisan 2009; Miller and McGill 2018). 이러한 다양한 조건의 변화로 인해 과거와 달리 기후변화에 적응하지 못하는 취약종들이 증가할 것으로 예측되고 있다 (Thomas et al. 2004).
서식지는 단순한 생물의 서식공간이 아니라 다양한 생태계 인자들과 생물이 상호작용하고 있는 종합적인 시스템으로 서식지의 건강성은 생물을 포함한 생태계 전체의 건강성을 평가할 수 있는 중요한 지표다. 이러한 중요성 때문에 유네스코의 인간과 생물권프로그램 (Man and the Biosphere program, MAB)에서는 서식지 보호의 중요성을 강조하고, 이를 위하여 멸종위기종 및 종다양성 보전을 위하여 중요한 서식지에 대하여 핵심지역, 완충지역, 전이지역으로 나누어 관리할 것을 권고하고 있다 (di Castri 1976). 또한 개발 등 생태계 훼손에 의해 파편화된 서식지의 경우 멸종위기종 등 주요 생물종의 서식지 보호를 위하여 서식지 복원 및 생태통로 설치 등과 같은 다양한 노력들이 이루어지고 있다 (Pascual-Hortal and Saura 2006). 그러나 서식지 보전을 위한 최적의 대응방안 마련을 위해서 먼저 주요종에 대한 적합서식지 분석 및 이를 바탕으로 미래 분포 변화 예측이 이루어져야 하며, 국내외에서 다수의 연구들이 진행되었다 (Svenning et al. 2008; Yun et al. 2014; Koo et al. 2015; Park et al. 2015, 2016b; Kim et al. 2017b; Koo et al. 2017a, b; Park et al. 2017; Koo et al. 2017c; Bosso et al. 2018; Koo et al. 2018; Avila and Charles 2018).
국내에서 수행된 대부분의 적합서식지 분석과 현재 분포 추정 및 이를 토대로 한 미래 분포 예측은 종의 분포 자료와 환경요인들간의 관계를 통해 적합서식지를 예측하고 종의 분포를 결정하는 종분포모형 (Species distribution model, SDM)을 활용하여 수행되었다 (Guisan and Zimmermann 2000; Guisan and Thuiller 2005; Elith and Leathwick 2009). 종분포모형은 상대적으로 모형 구현에 진입 장벽이 크지 않고 종의 서식지 환경 및 변화에 관한 분석이 용이하기 때문에 전세계적으로 서식지 보전에 관한 다양한 연구에 활용되고 있다 (Wiens et al. 2009; Song and Kim 2012; Cho et al. 2015; Do et al. 2017; Wang et al. 2018; Ikegami and Jenkins 2018; Raghavan et al. 2019; Runquist et al. 2019; Namgung et al. 2019). 국내에서는 아고산 지역에 서식하는 한대성 식물을 대상으로 서식지 적합도 추정 및 분포 변화 예측을 통한 취약성 분석 연구들 (Koo et al. 2015, 2017a; Park et al. 2015; Lim et al. 2018)과 온대 및 난대성 식물을 대상으로 기후변화에 따른 서식지 확장 및 변화 예측에 관한 연구들이 진행되었다 (Yun et al. 2011c, 2014; Park et al. 2016a; Koo et al. 2017b; Park et al. 2017; Koo et al. 2017c, 2018; Lim et al. 2018). 이러한 국내외에서 이루어진 대부분의 연구들은 기후 및 지형과 같은 자연조건만을 고려하고, 미래 분포 예측에 매우 중요한 인자인 산포와 같은 생물적 인자를 고려하지 않았다는 한계가 있다 (Engler and Guisan 2009; Park et al. 2017). 생물적 인자를 고려하지 않은 경우, 현재의 적합서식지 평가 및 분포 추정에는 유용하나 미래의 분포 예측은 매우 비현실적인 것으로 보고되었다 (Dormann et al. 2012). 이러한 문제점을 해결하기 위하여 국외에서는 식물의 산포를 설명하는 다양한 산포모형 (Gómez and Espadaler 1998; Bullock and Clarke 2000; Vellend et al. 2003)을 종분포모형에 결합하여 생물의 미래 분포를 예측하는 연구를 수행해왔다 (Bateman et al. 2013; Miller and McGill 2018).
환경부는 기후변화에 관한 생물의 영향을 연구하기 위한 기후변화 생물지표 (Climate-sensitive Biologocal Indicator Species, CBIS) 100종을 지정하였으며 (Lee et al. 2010), 이 종들을 활용한 다양한 기후변화 관련 연구가 수행되었다 (Yun et al. 2014; Koo et al. 2015; Park et al. 2015, 2016b; Kim et al. 2017b; Koo et al. 2017a, b; Park et al. 2017; Koo et al. 2017c, 2018). 본 연구에서는 이러한 기후변화 생물지표종에서 각기 다른 서식환경을 보이는 3종 (구상나무, 식나무, 동백나무)을 선택하여 현재 기후에서의 기후적합서식지를 추정하고 이를 바탕으로 현재 분포 및 기후변화에 따른 미래 분포 변화를 예측하고자 하였다. 또한 미래 예측에서 산포 능력을 고려하여 보다 현실적인 분포 변화를 예측하고, 이를 근거로 기후변화에 대한 종별 취약성을 평가하였다.
2. 연구방법 및 자료
2.1 연구종 및 연구지역
기후변화 생물지표종은 기후변화에 민감하게 영향을 받는 종으로 한반도 생태계에 대한 기후변화 영향 및 평가 연구에 활용되어 왔다 (Yun et al. 2014; Koo et al. 2015; Park et al. 2015, 2016b; Kim et al. 2017b; Koo et al. 2017a, b; Park et al. 2017; Koo et al. 2017c, 2018). 본 연구의 대상종은 아고산 생태계의 대표적인 기후변화 민감종인 구상나무 (Abies koreana E.H.Wilson), 난대림의 기후변화 민감종인 식나무 (Aucuba japonica Thunb.)와 동백나무 (Camellia japonica L.) 3종이다 (Table 1). 구상나무는 소나무과 한대성 상록침엽수로 한반도의 아고산에만 서식하는 고유종이다. 구상나무는 고산식물로써 제주도와 지리산 등 아고산지역의 서식지에서 기후변화에 따른 고사 현상이 보고되고 있으며, 고온과 건조스트레스에 민감한 것으로 보고되었다 (Koo et al. 2001, 2017a; Lim et al. 2006; Song et al. 2010, 2014; Kim and Lee 2013). 식나무는 식나무과에 속하는 난대성 상록활엽수로 주로 남부 해안 지역의 숲 속에서 서식한다 (Lee 1980). 동백나무는 차나무과 난대성 상록활엽수로 남해안부터 중부 해안까지 해안가 산록에 자생한다 (Lee 1980). 이 두 수종은 난대성 상록활엽수로 기후온난화에 따라 북쪽으로 그 분포를 확장하거나 분포지가 북쪽으로 이동할 것으로 예측되고 있다 (Park et al. 2016a).
한반도는 북위 33~43도, 동경 124~132도에 위치하며 3면이 바다로 둘러 쌓여있는 반도로 동고서저의 지형 경관을 보인다. 전체 국토의 약 70%가 산지이며 서해와 남해 등에 약 3600여개의 무인도와 유인도가 있는 것으로 보고되었다 (National Geographic Information Institute 2014). 이러한 지형 경관은 도서 지역과 내륙 산지에 다양한 동식물이 서식할 수 있는 서식지를 제공해 주었다 (Kong 2005; Kim et al. 2017a). 특히, 수직적으로 저지대부터 고산 및 아고산 지역까지 다양한 서식지 환경의 분포는 난대성 생물종에서 한대성 생물종까지 다양한 생물종의 분포를 가능하게 했다 (Kong 2007). 이와 더불어 기후는 연평균 10~15°C, 봄철의 건조 스트레스, 여름철의 많은 강수량 등 다양한 생물종이 서식할 수 있는 기후환경을 제공하고 있다 (Korea Meteorological Administration 2012).
2.2 환경변수들
생물기후변수 (Bioclimatic variables)는 기온과 강수를 계절적 시기에 따라 조합하여 식물의 서식 환경을 추정할 수 있는 지수로써 총 19개의 변수가 있으며, 생물의 서식에 중요한 환경요소로써 생태적지위모형 (Ecological niche model) 연구에서 중요한 인자로 고려되어 왔다 (Araújo and Guisan 2006; O’Donnell and Ig-nizio 2012). 기후변화에 따른 생태계 영향 평가 연구에 생물기후변수가 다양하게 활용되고 있으며, 19개의 모든 변수를 사용하는 것보다 상관분석, 주성분분석 등을 통해 대표성이 있는 변수를 선정하여 사용해왔다 (Koo et al. 2015; Park et al. 2017). 본 연구에서는 변수간의 Pearson’s correlation을 계산하여 상관관계가 높은 변수 (r > 0.7)를 분석에서 배제함으로써 상관관계가 높은 변수들의 중복된 사용을 피하였다. 각 수종들에 대한 기후변화 관련 생리·생태 연구의 미흡으로 상관관계가 높은 두 변수 중 각 생물종 서식지의 수직적, 수평적 기후환경과 계절적 기후환경의 특성을 반영할 수 있는 종속변수를 선별하였다 (Bio1, 2, 3, 12, 13, 14). Bio1과 Bio12는 연평균 기온과 강수량으로 우리나라 및 전세계적의 생물기후대를 설명하는데 사용되고 있는 대표적인 변수이며, Bio2와 Bio3는 기온의 연교차와 일교차의 공간적 분포를 잘 설명하는 변수다. 특히 Bio2의 경우 연교차가 북부와 고산 및 아고산에 비해 적은 남부 해안가에 사는 난대성 상록활엽수의 기후환경을 잘 설명한다. 또한, Bio14는 일반적으로 대부분의 우리나라 식물들이 영향을 받는 기후스트레스인 봄철 건조스트레스를 설명하며, Bio13는 집중호우와 태풍 등에 의한 스트레스를 설명하는 변수다 (Koo et al. 2015, 2018; Park et al. 2016b) (Table 2).
본 연구에서는 Worldclim에서 제공하는 30 Arc-second (ca 1 km2) 해상도의 1960년~1990년를 대표하는 Worldclim version 1.4의 기후 자료를 사용하였다. 전세계적으로 약 61개의 Global Circulation Model (GCM)이 개발되어 있으며, 각 모형은 4개의 Representative Concentration Pathways (RCP) 시나리오에 따라 미래 기후변화를 예측하고 있다 (Taylor et al. 2012). 4개의 RCP 시나리오는 RCP 2.6, 4.5, 6.0, 8.5로, 이 시나리오의 숫자는 인류가 배출할 것으로 예상되는 온실가스의 양에 따라 대기중에 축적될 것으로 예상되는 온실가스 농도를 의미한다 (Riahi et al. 2011). 그러므로 RCP 2.6에서 8.5로 갈수록 대기중 온실가스 농도가 증가하고, 이에 따라 기후변화의 정도. 기존의 기후변화 관련 식물 분포 변화 연구에서는 기후변화의 미래예측에서 다양한 모형에 따라 발생할 수 있는 불확도를 줄이기 위하여 여러 개의 GCM 예측 결과를 앙상블 하여 사용하고 있다 (Wang et al. 2012). 특히, 우리나라의 경우 자체 개발한 GCM이 없는 상황에서 한 개의 GCM 예측 결과를 사용하는 것보다는 다양한 GCM의 예측 결과를 앙상블 하여 사용하는 것이 불확도를 줄이는데 효과적일 것이다. 그래서 본 연구에서는 미래 기후변화 예측을 위하여 현재 국내에서 가장 많이 활용되며, 지리적으로 인접하여 우리나라 기후변화 예측력이 상대적으로 높을 것으로 판단되는 5개의 GCM을 선정하였다. 이 5개의 모형에 RCP 2.6, 4.5, 6.0, 8.5 시나리오를 각각 적용하여 20개의 2050년 (2041~2060) 기후변화 예측 결과를 모의하고, 이를 앙상블 하여 미래 기후변화 자료로 사용하였다 (Watanabe et al. 2011; The HadGEM2 Development Team: G. M. Martin et al. 2011; Yukimoto et al. 2012; Schmidt et al. 2014) (Table 3).
2.3 모형개발 및 검증
종분포모형의 개발에 필요한 연구종의 출현/비출현자료는 구경아 (2000), 이우철과 임양재 (2002), 국립수목원의 한반도 관속식물 분포도 (2004; 2005; 2007; 2008; 2009; 2010a; 2010b; 2011), 환경부의 제3차 전국자연환경조사 (2006; 2007; 2008; 2009; 2010; 2011)에 수록된 분포자료를 활용하였다. 식물조사 지점이 동일하거나 인접한 지점을 통합하여 각 종별 634개에서 757개의 식물분포자료를 사용하였다. 각 지점에서 연구대상종이 분포하지 않는 것으로 기록된 지점을 비출현자료로 활용하였다.
종분포모형의 개발을 위해 연구종의 출현/비출현 지점의 bio1~3과 bio12~14 환경변수를 추출하고 추출된값들을 70대 30의 비율로 훈련자료 (Training dataset)와 시험자료 (Test dataset)로 무작위 분할하였다. 자료 분할에서 나타나는 분할의 편향성을 최소화하기 위해 위 분할 과정을 각 종별 50회 반복 시행하였다. 50번 분할된 훈련자료에 개별 알고리즘에서 신뢰도가 높은 것으로 알려진 4개의 알고리즘 (Random Forest (RF), Generalized Boosting Model (GBM), Generalized Additive Model (GAM), Multiple Adaptive Regression Splines (MARS))을 적용하여 개별 종분포모형을 구현하였고 시험자료를 사용하여 각 모형을 검증하였다 (Kwon et al. 2012; Park et al. 2016b). 개별 모형에서 오는 불확도를 최소화하기 위해 모형의 검증에서 TSS (True Statistic Skill) 값이 0.7보다 높은 종분포모형을 선정하고 각 모형에 TSS 값으로 가중치 (TSS Weighted Ensemble Model, TWE)를 부여하여 앙상블 하였으며 해당 모형은 R의 Biomod2 패키지를 활용하였다 (Thuiller et al. 2009) (Equation 1).
(Eq.1) |
i는 개별 종분포모형, Pi는 개별 i 종분포모형에 의해 계산된 분포적합도, TSSi는 종분포모형 i의 TSS 값이며 PTWE는 기후변화 생물지표의 TSS 가중치 앙상블예측
종분포모형은 종의 출현 확률로 서식지의 적합도를 표현하기 때문에 종의 분포를 설명하기 위해서는 출현과 비출현의 경계를 결정하는 임계점 (threshold)이 되는 출현 확률을 계산하는 것이 필수적이다. 본 연구의 종분포모형에서 임계점 설정은 최근 연구에서 많이 사용되고 있는 TSS 값이 최대가 되는 지점을 적용하였다 (Allouche et al. 2006). 모형의 정확도는 TSS 값과 AUC (Area Under the Curve) 값을 사용하여 평가하였다 (Landis and Koch 1977; Pearson 2007).
종분포모형은 미래 환경변화에 따른 분포 변화 예측에 있어서 종의 산포 능력을 무제한으로 가정한다. 그러나, 종은 각기 다른 산포 유형을 보이며 유형에 따라 확장 범위가 다르기 때문에 산포 능력을 무제한으로 가정하는 것은 비현실적이다 (Thomas et al. 2004; Thuiller et al. 2005). 이러한 한계점을 극복하기 위해 Cellular Automata를 활용한 R의 MigClim패키지를 사용하여 종의 산포 가능성을 모의하였다 (Engler and Guisan 2009). 종의 산포 능력은 역지수함수를 사용하여 거리에 따른 확률을 산출하였다 (Equation 2). 역지수함수의 기울기는 동물에 의해 산포 가능한 거리의 최대치인 5 km (기울기: -0.001)를 기본으로 하였으며, 이보다 먼 10 km를 산포할 수 있는 기울기 -0.0005를 추가하여 바람 등에 의한 장거리 산포도 고려하였다 (Vellend et al. 2003). 또한 산포 과정에서 각 식물은 성장하여 씨앗을 생산할 수 있는 기간이 필요하며, 이러한 기간은 산포의 지연 요인으로 작용한다 (Engler and Guisan 2009). 씨앗의 성장 기간은 서식지의 환경에 따라 각기 달라지며, 정확한 모의를 위해서는 각 서식지별 연구 자료가 필요하나 연구종에 대한 이러한 연구의 부재로 미래 예측에 있어서 5년, 10년, 15년, 20년의 다양한 기간을 씨앗의 성장기간으로 가정하였다. 모의는 현재 분포 지역에서 시작하여 1990년부터 2050년까지 60년간 60번의 산포 모의를 통해 미래 분포 변화를 예측하였다.
(Eq.2) |
Diθ는 θ에 따른 거리별 산포 확률, θ는 역지수함수의 기울기로 -0.001과 -0.0005를 각각 적용
3. 연구결과
3.1 기후적합서식지 및 현재 분포
현재 기후 (1960~1990년)에서 각 연구종에 대하여 서식지 기후적합도가 예측되었으며, TSS값이 최대가 되는 임계점을 기준으로 현재의 기후적합서식지를 예측하고 이를 바탕으로 현재의 분포를 추정하였다 (Fig. 1). 각 종별 서식지의 기후적합도는 출현 확률로 계산되어 5개의 그룹으로 분류되어 제시되고 있으며, 현재의 기후적합서식지와 이를 바탕으로 예측된 현재 분포는 지도상에 흰색을 제외한 지역으로 제시되어 있다 (Fig. 1). 현재 분포 예측에 대한 각 모형의 예측력을 TSS와 AUC 값을 계산하여 평가한 결과, 모든 모형의 예측력이 매우 높은 것으로 분석되었다 (Table 4). TSS 값을 기준으로 구상나무 종분포모형은 0.98, 식나무는 0.91, 동백나무는 0.82를 보였다. TSS 값으로 평가한 모형 예측력에서는 동백나무가 구상나무와 식나무에 비하여 다소 낮은 예측력을 보였으나, AUC 값을 기준으로 보면 3개종 모두 0.95를 넘는 매우 우수한 예측력을 보였다.
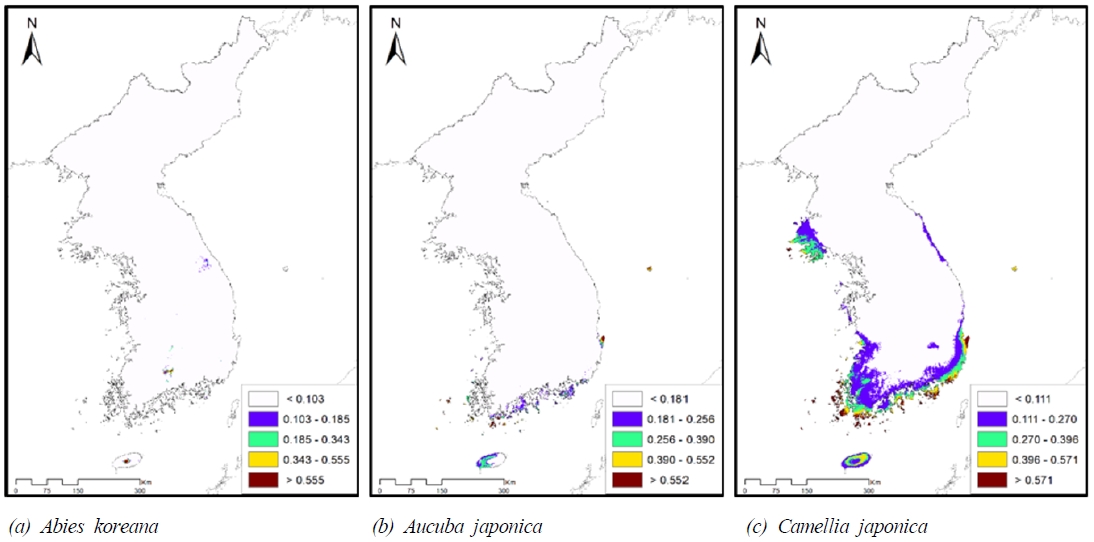
Habitat suitability and potential habitats of study species under current climatic conditions. White color on the map indicates the absent of species and other colors presence. Other colors also present habitat suitability calculated by the probability of species presence under current climatic conditions.

Evaluation statistics of ensemble models. TSSs, AUCs, Sensitivities and Specificities were calculated from the ensemble projection of each species using the full dataset.
모형예측결과 현재 기후에서 기후적합서식지를 근거로 예측된 구상나무의 분포지역은 898 km2, 식나무는 3,996 km2, 동백나무 37,823 km2으로 추정되었다. 구상나무의 경우, 현재 구상나무가 분포하는 지역 외에도 설악산 인근과 강원도 산악지역까지 기후적합서식지인 것으로 예측되어, 예측된 분포지역이 실제 분포지역보다 다소 넓은 것으로 나타났다. 식나무의 경우 현재 분포 지역과 모형 예측에 의해 추정된 분포지역이 대부분 일치하며 남부 해안 및 도서지역에 주로 분포하는 것으로 나타났다. 동백나무는 난대성 상록활엽수 중에서 중부 해안지역까지 자생하는 것으로 알려진 종으로 모형의 결과에서도 동일하게 남부 내륙과 중부 해안지역까지 분포하는 것으로 예측되었다 (Fig 1). 각 수종별 분포지역 내 서식지 기후적합도를 살펴보면, 구상나무는 대부분 지역에서 낮은 적합도를 보인 반면, 식나무는 일부 해안지역의 적합도가 높게 나타났고, 동백나무는 남부 해안과 중부 해안의 도서지역에서 높은 적합도를 보였다.
3.2 기후변화에 따른 분포 변화 예측: 무제한 산포 가정
기후변화 시나리오에 따라 예측된 20개의 2050년 기후를 앙상블 하고 이를 적용하여 미래 기후적합서식지를 추정하였다 (Fig. 2). 미래 기후적합서식지만을 고려하여 분포 변화를 예측한 결과, 구상나무는 9,763 km2, 식나무는 7,659 km2, 동백나무는 80,922 km2로 모두 분포지가 확대될 것으로 예측되었다. 구상나무의 경우 기존에 존재하던 제주도, 지리산, 설악산 등 모든 지역에서 분포가 확대되는 것으로 나타났으며, 식나무는 서해안 중부지역, 동백나무는 북부 해안지역, 중부 해안지역에서 중부 내륙지역까지 분포가 확대될 것으로 예측되었다 (Fig 2). 적합도 변화를 살펴보면, 구상나무는 모든 지역에서 적합도가 상승하였으며, 식나무의 경우 분포 면적이 확대되는 것과 반대로 모든 지역에서 적합도는 하락하는 것으로 예측되었다. 동백나무의 경우도, 미래 기후에서 분포는 증가하지만 현재 기후에서 높은 적합도를 보이던 지역의 적합도가 하락하는 것으로 예측되었다. 예를 들어 제주도 중산간 지역은 현재 기후에서는 0.270~0.396과 0.396~0.571범위의 적합도를 보였지만 미래 기후에서는 0.111~0.270으로 떨어졌다. 또한 진도, 거제도를 포함한 남해안과 신안군 인근의 서해 도서에서는 0.571 이상의 적합도에서 0.270~0.396 또는 0.111~0.270으로 하락하는 것으로 나타났다.
3.3 기후변화에 따른 분포 변화 예측: 제한된 산포 가정
기후적합서식지 예측에 산포 능력 (산포 거리와 첫번째 씨앗의 생산 기간)을 결합하여 종별 2050년 분포를 예측하였다 (Fig. 3). 모형에 의해 예측된 현재 분포 지역은 산포 모형의 시작 지점으로 사용되었으며, 미래 기후적합서식지는 산포 가능지역으로 활용되었다. Fig. 3에서 현재 분포 (Current distribution)는 현재 기후에서 기후적합서식지를 설명하며, 서식지 유실 (Habitat loss)는 기후변화에 따른 서식지 유실을 의미한다. 산포를 고려한 미래 분포 (Future distribution with dispersal)는 산포 능력과 기후변화를 모두 고려한 예측으로, 산포 능력의 제한 하에서 각 종의 기후변화에 따른 미래 분포 변화를 보여준다.
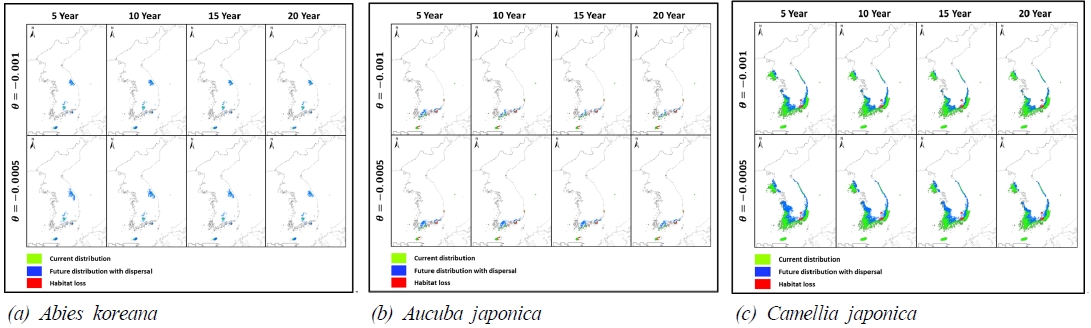
Ensemble forecast of study species in 2050 under dispersal limitations. The left axis is the coefficient value for the negative exponential equation (θ: -0.005, -0.001), and the top axis is the first reproduction year (5, 10, 15, 20 years). Current distribution (green color on the map) explains that the distribution of study species will stay in the future climate condition. Future distribution with dispersal (blue color on the map) explains the areas where their distributions will expand under future climate condition and dispersal limitations. Habitat loss (red color on the map) explains the areas where they lose its climatic suitable habitats under climate change.
구상나무의 2050년 분포는 산포 능력의 제한을 고려하여도 확장되는 것으로 예측되었다. 다만 철원 및 경기 북부 내륙까지는 확장되지 못하였고 산포 가능 거리에 따라 강원도 내륙 산악지역의 확장이 크게 감소하는 것으로 예측되었다 (Fig 3 (a)). 식나무는 산포 능력을 고려할 경우 미래 기후에서 기후적합서식지로 예측된 중부 해안지역과 남부 내륙지역까지 확장하지 못하는 것으로 예측되었다 (Fig 3 (b)). 동백나무는 산포 능력을 고려하면 미래 기후에서 기후적합서식지로 예측된 북부 해안 및 중부 내륙까지 확장하지 못하는 것으로 예측되었으며, 중부 해안 지역과 남부 내륙 지역도 산포 능력에 따라 확장이 제한되는 것으로 나타났다 (Fig 3 (c)).
산포 능력을 고려한 미래 분포 면적은 산포 모형의 기울기와 재생산 기간이 짧아지는 것에 따라 늘어나는 경향성을 보였다 (Table 5). 구상나무 분포 면적은 산포 모형의 기울기에 따라 최소 5,650 km2에서 최대 8,595 km2를 보였다. 이는 산포 능력을 무제한으로 가정하고 예측한 미래 분포 면적에 비해 58%와 88%만이 실제 분포 가능 면적으로 예측됨으로서 분포 확장이 제한됨을 보여준다. 이는 산포 능력을 고려한다면 구상나무의 미래 분포 면적이 12%에서 42%까지 감소할 수 있다는 것을 의미한다. 식나무의 분포 면적은 산포 모형의 기울기에 따라 최소 4,473 km2에서 최대 6,956 km2로 산포 능력을 무제한으로 가정한 예측 결과에 비해 10%에서 42%의 분포 면적이 감소하는 것으로 예측되었다. 동백나무의 경우, 산포 모형에 따라 분포 면적은 51,434 km2와 69,683 km2로 예측되었고, 산포 능력을 무제한으로 가정한 예측 결과에 비해 14%에서 37%의 분포 면적이 감소하는 것으로 예측되었다. 산포 능력을 고려한 경우 구상나무의 분포 면적 감소가 다른 2종에 비해 비교적 큰 것으로 예측되었으며, 동백나무가 가장 낮은 감소율을 보였다.

The future distribution of study species according to dispersal capacity and the ratio of climatic suitable habi-tats assumed unlimited dispersal vs. those restricted by dispersal processes. The dispersal capacity is a func-tion of dispersal distance, coefficients for the negative exponential equation, and the first reproduction year
4. 고찰
앙상블 기법은 개별 모형 및 자료에서 야기될 수 있는 불확실성을 줄일 수 있는 방법으로 전세계적으로 기후변화에 따른 종의 분포 변화 연구에 많이 사용되어 왔다 (Diniz-Filho et al. 2009; Buisson et al. 2010; Jones et al. 2013; Zhang et al. 2015; Jones and Cheung 2015). 이에 따라 본 연구에서도 개별 종분포모형에서 나타나는 불확실성을 낮추고 모형 예측력을 높이기 위하여 개별 종분포모형을 앙상블하여 사용하였고, 그 결과 TSS 값 0.96, 0.91, 0.82와 AUC 값 0.99, 0.98, 0.97의 높은 정확도를 확보할 수 있었다. 미래 기후 자료의 경우에도, 연구 지역인 한반도의 기후 특성에 맞게 개발된 GCM이 없는 상태에서 한반도의 기후를 비교적 잘 반영할 것으로 판단되는 5개의 GCM 기후 자료를 선별하여 앙상블함으로서 기후 모형에 의해 발생할 수 있는 불확실성을 최소화하였다 (Wang et al. 2012). 특히, 본 연구는 동일한 공간 스케일과 환경변수를 사용하여 각기 다른 생태적 특징을 갖는 기후변화 생물지표종에 대한 기후변화 영향 및 취약성을 평가하여, 한반도 생태계의 다양한 기후변화 영향을 평가하는 기초자료를 제공할 것으로 판단된다.
종분포모형은 종의 출현과 환경요인과의 상관관계를 통해 종의 출현 가능성을 추정하기 때문에 다양한 생태적 기작을 고려하지 못하는 한계점을 가지고 있다 (Wiens et al. 2009; Koo et al. 2015). 이를 보완하기 위하여 최근 기후변화에 따른 종분포 변화 연구에서는 산포와 같은 생태적 과정을 반영하고 있으며, 본 연구에서도 산포 과정을 적용하여 각 연구종의 미래 분포 변화를 예측하였다 (Engler and Guisan 2009). 산포 과정을 적용한 결과 각 연구종의 기후변화에 대한 취약성이 증가하는 것으로 나타났으며, 이를 통해 본 연구는 산포 과정 등 생태적 과정이 미래 종분포 예측에 중요한 인자임을 잘 보여주었다. 또한, 연구종의 산포 과정에 대한 연구가 미비하여, 좀더 정확한 미래 분포 예측 및 기후변화 영향 평가를 위해 산포 기작을 밝히는 연구의 수행이 필요함을 보여주었다.
본 연구에서 구상나무의 미래 분포 면적이 확장하는 것으로 예측되었으나 대부분의 기존 연구에서는 구상나무의 분포 감소가 예측되었다 (Choi et al. 2011; Park et al. 2015; Koo et al. 2017a). 또한 현장 조사에서도 구상나무 생장의 쇠퇴가 보고되었다 (Koo et al. 2001; Choi et al. 2011; Song et al. 2014). 이는 본 연구의 예측이 한반도를 공간 범위로 기후 인자만 고려한 반면, 기존의 연구들은 한라산과 같이 지역단위의 공간적 범위에서 미기후, 미소 지형, 종간 경쟁 등 다양한 인자들의 영향을 고려하였기 때문으로 판단된다. 또한 기후변화 시나리오에서는 미래 강수량이 증가하는 것으로 예측하고 있으며, 이러한 강수량의 증가가 기온상승에서 오는 부정적인 영향을 상쇄하여 구상나무 분포 확대 예측을 가져온 것으로 판단된다. 기존의 구상나무 생장과 기후 인자와의 관계를 밝히는 연구에서도 구상나무 생장은 건조스트레스에 매우 민감한 것으로 보고되었다 (Koo et al. 2001). 그러나 구상나무의 적응 능력이나 경쟁 및 상리 공생 등 다양한 연관종과의 상호작용 등을 고려하지 않고 기후조건만을 가지고 구상나무의 미래 분포를 설명하는 것은 한계점을 가진다. 그러므로 좀더 정확한 구상나무 미래 분포 예측을 위하여 다양한 생태적 연구들이 수행되어야 하며 이러한 연구를 바탕으로 한 모형 개발이 수행되어야 할 것이다.
식나무와 동백나무를 포함한 남부 지역에 분포하는 난대성 수종은 기후변화에 따른 취약성이 높지 않는 것으로 여겨지고 있으며 (Klötzli and Walther 1999; Koo 2000; Lee and Choi 2010; Yun et al. 2011a), 본 연구의 결과도 분포의 확장을 예측하였다. 그러나 산포 능력을 고려했을 때 그 확장 면적은 크게 감소하는 것으로 나타났으며, 특히 식나무의 경우 서식지가 해안지역에 분포하는 것을 고려했을 때, 해수면 상승과 같은 기후변화에 따라 나타나는 2차적인 영향으로 서식지 유실이 일어날 것으로 추정된다 (Kim and Cho 2016). 또한, 해안지역은 거주지, 도로, 관광지 등으로 인간에 의한 토지의 전용이 활발한 지역으로 인간에 의한 서식지 교란 및 파괴에 이한 서식지 유실이 예측된다 (Choi 2014). 그러므로 모형의 예측 정확도를 높이기 위하여 인간에 의한 토지이용 변화 및 기후변화에 따라 나타나는 다양한 현상을 고려한 모형의 개발이 필요하며, 이러한 인자들을 고려할 경우 기후변화에 대한 식나무의 취약성이 더욱 높아질 것으로 판단된다. 동백나무의 경우에도 좀더 정확한 예측을 위하여 기존 중부지역에 서식하는 온대성 식물과의 종간 경쟁 등 다양한 생태적 요인을 고려하는 예측이 필요하며 이를 위한 모니터링 및 기초 생태 연구 결과가 필요할 것으로 보인다.
5. 결론
본 연구에서는 종분포모형과 산포모형을 사용하여 기후변화 생물지표 3종 (구상나무, 식나무, 동백나무)의 기후변화에 따른 분포 변화를 예측하였다. 현재 기후에서 구상나무의 기후서식적합지를 바탕으로 추정된 분포 지역은 제주도와 남부 및 중부 고산 지역으로 예측되었으며, 식나무는 남부해안 및 도서지역, 동백나무는 남부 내륙과 중부 해안지역으로 예측되었다. 미래 기후에서 모든 연구대상종의 분포 지역이 확대되는 것으로 예측되었다. 서식적합도를 고려했을 때, 미래 기후에서 구상나무의 기후서식적합도가 모든 지역에서 높게 나타났으나, 동백나무와 식나무는 서식지 적합도가 전체적으로 감소하는 것으로 나타나 질적으로 우수한 서식지의 감소가 예측되었다. 산포 능력을 고려하여 미래분포변화를 예측한 결과, 연구종 모두에서 산포 능력을 고려하지 않은 경우에 비해 분포 지역 확대 정도가 최소 10%에서 최대 42%까지 감소하는 것으로 예측되었다. 이는 기후조건이 적합해진다해도 산포와 같은 생태 조건을 고려한다면 실제 서식지 확장 폭이 제한될 수 있음을 보여주는 것으로 산포 과정 등 생태적 기작과 과정에 대한 연구의 필요성과 이러한 연구 결과들을 통합한 서식지 모형의 개발이 필요함을 보여준다.
Acknowledgments
본 연구는 환경부의 재원으로 한국환경산업기술원의 기후변화대응환경기술사업 (2018001310004)과 2017년도 정부 (과학기술정보통신부)의 재원으로 한국연구재단의 지원을 받아 수행된 기초연구사업 (NRF-2017R1A2B4007428)에 의해 이루어졌습니다.
References
-
Allouche O, Tsoar A, Kadmon R (2006) Assessing the accuracy of species distribution models: prevalence, kappa and the true skill statistic (TSS). Journal of Applied Ecology 43: 1223-1232. x
[https://doi.org/10.1111/j.1365-2664.2006.01214.x]
-
Araújo MB, Guisan A (2006) Five (or so) challenges for species distribution modelling. Journal of Biogeography 33: 1677-1688.
[https://doi.org/10.1111/j.1365-2699.2006.01584.x]
-
Avila GA, Charles JG (2018) Modelling the potential geographic distribution of Trissolcus japonicus: a biological control agent of the brown marmorated stink bug, Halyomorpha halys. BioControl 63: 505-518.
[https://doi.org/10.1007/s10526-018-9866-8]
-
Bateman BL, Murphy HT, Reside AE, et al (2013) Appropriateness of full-, partial-and no-dispersal scenarios in climate change impact modelling. Diversity and Distributions 19: 1224-1234.
[https://doi.org/10.1111/ddi.12107]
-
Bosso L, Smeraldo S, Rapuzzi P, et al (2018) Nature protection areas of Europe are insufficient to preserve the threatened beetle Rosalia alpina (Coleoptera: Cerambycidae): evidence from species distribution models and conservation gap analysis. Ecological Entomology 43: 192-203.
[https://doi.org/10.1111/een.12485]
-
Buisson L, Thuiller W, Casajus N, et al (2010) Uncertainty in ensemble forecasting of species distribution. Global Change Biology 16: 1145-1157.
[https://doi.org/10.1111/j.1365-2486.2009.02000.x]
-
Bullock JM, Clarke RT (2000) Long distance seed dispersal by wind: measuring and modelling the tail of the curve. Oecologia 124: 506-521.
[https://doi.org/10.1007/PL00008876]
-
Butchart SHM, Walpole M, Collen B, et al (2010) Global Biodiversity: Indicators of Recent Declines. Science 328: 1164-1168.
[https://doi.org/10.1126/science.1187512]
-
Cho H-J, Kim D-H, Shin M-S, et al (2015) Predicting the Goshawk’s habitat area using Species Distribution Modeling: Case Study area Chungcheongbuk-do, South Korea. Korean Journal of Environment and Ecology 29: 333-343.
[https://doi.org/10.13047/KJEE.2015.29.3.333]
-
Choi S, Lee W-K, Kwak D-A, et al (2011) Predicting forest cover changes in future climate using hydrological and thermal indices in South Korea. Climate Research 49: 229-245.
[https://doi.org/10.3354/cr01026]
-
Choi YR (2014) Modernization, Development and Underdevelopment: Reclamation of Korean tidal flats, 1950s-2000s. Ocean & Coastal Management 102: 426-436.
[https://doi.org/10.1016/j.ocecoaman.2014.09.023]
-
di Castri F (1976) International, interdisciplinary research in ecology: Some problems of organization and execution. The case of the man and the biosphere (MAB) programme. Hum Ecol 4: 235-246.
[https://doi.org/10.1007/BF01534288]
-
Diniz-Filho JAF, Bini LM, Rangel TF, et al (2009) Partitioning and mapping uncertainties in ensembles of forecasts of species turnover under climate change. Ecography 32: 897-906.
[https://doi.org/10.1111/j.1600-0587.2009.06196.x]
-
Do MS, Lee J-W, Jang H-J, et al (2017) Spatial Distribution Patterns and Prediction of Hotspot Area for Endangered Herpetofauna Species in Korea. Korean Journal of Environment and Ecology 31: 381-396.
[https://doi.org/10.13047/KJEE.2017.31.4.381]
-
Dobrowski SZ (2011) A climatic basis for microrefugia: the influence of terrain on climate. Global Change Biology 17: 1022-1035.
[https://doi.org/10.1111/j.1365-2486.2010.02263.x]
-
Dormann CF, Schymanski SJ, Cabral J, et al (2012) Correlation and process in species distribution models: bridging a dichotomy. Journal of Biogeography 39: 2119-2131.
[https://doi.org/10.1111/j.1365-2699.2011.02659.x]
-
Elith J, Leathwick JR (2009) Species Distribution Models: Ecological Explanation and Prediction Across Space and Time. Annual Review of Ecology, Evolution, and Systematics 40: 677-697.
[https://doi.org/10.1146/annurev.ecolsys.110308.120159]
-
Engler R, Guisan A (2009) MigClim: Predicting plant distribution and dispersal in a changing climate. Diversity and Distributions 15: 590-601.
[https://doi.org/10.1111/j.1472-4642.2009.00566.x]
-
Etterson JR, Shaw RG (2001) Constraint to Adaptive Evolution in Response to Global Warming. Science 294: 151-154.
[https://doi.org/10.1126/science.1063656]
-
Gavin DG, Fitzpatrick MC, Gugger PF, et al (2014) Climate refugia: joint inference from fossil records, species distribution models and phylogeography. New Phytologist 204: 37-54.
[https://doi.org/10.1111/nph.12929]
-
Gentili R, Baroni C, Caccianiga M, et al (2015) Potential warm-stage microrefugia for alpine plants: Feedback between geomorphological and biological processes. Ecological Complexity 21: 87-99.
[https://doi.org/10.1016/j.ecocom.2014.11.006]
-
Gómez C, Espadaler X (1998) Myrmecochorous dispersal distances: a world survey. Journal of Biogeography 25: 573-580.
[https://doi.org/10.1046/j.1365-2699.1998.2530573.x]
-
Guisan A, Thuiller W (2005) Predicting species distribution: offering more than simple habitat models. Ecology Letters 8: 993-1009.
[https://doi.org/10.1111/j.1461-0248.2005.00792.x]
-
Guisan A, Zimmermann NE (2000) Predictive habitat distribution models in ecology. Ecological Modelling 135: 147-186.
[https://doi.org/10.1016/S0304-3800(00)00354-9]
-
Ikegami M, Jenkins TAR (2018) Estimate global risks of a forest disease under current and future climates using species distribution model and simple thermal model - Pine Wilt disease as a model case. Forest Ecology and Management 409: 343-352.
[https://doi.org/10.1016/j.foreco.2017.11.005]
- Intergovernmental Panel on Climate Change (2018) Global warming of 1.5°C.
-
Intergovernmental Panel on Climate Change, Edenhofer O (eds) (2014) Climate change 2014: mitigation of climate change: Working Group III contribution to the Fifth Assessment Report of the Intergovernmental Panel on Climate Change. Cambridge University Press, New York, NY.
[https://doi.org/10.1017/CBO9781107415416]
-
Jones MC, Cheung WWL (2015) Multi-model ensemble projections of climate change effects on global marine biodiversity. ICES J Mar Sci 72: 741-752.
[https://doi.org/10.1093/icesjms/fsu172]
-
Jones MC, Dye SR, Fernandes JA, et al (2013) Predicting the Impact of Climate Change on Threatened Species in UK Waters. PLOS ONE 8:e54216.
[https://doi.org/10.1371/journal.pone.0054216]
- Kim H, Kim D, Jeon C, et al (2017a) Island-Biogeographical Characteristics of Naturalized Plant in Jeollanamdo Islands. Environmental impact assessment 26: 273-290.
-
Kim N-S, Lee H-C (2013) A Study on Changes and Distributions of Korean Fir in Sub-Alpine Zone. Journal of the Korea Society of Environmental Restoration Technology 16: 49-57.
[https://doi.org/10.13087/kosert.2013.16.5.049]
-
Kim Y, Cho K (2016) Sea level rise around Korea: Analysis of tide gauge station data with the ensemble empirical mode decomposition method. Journal of Hydro-environment Research 11: 138-145.
[https://doi.org/10.1016/j.jher.2014.12.002]
-
Kim Y-Y, Leem H, Han S, et al (2017b) Conservation Measures and Distribution of Vulnerable Species for Climate Change in Gayasan National Park. Korean Journal of Plant Resources 30: 167-175.
[https://doi.org/10.7732/kjpr.2017.30.2.167]
-
Klötzli F, Walther G-R (1999) Recent vegetation shifts in Switzerland. In: Klötzli F, Walther G-R (eds) Conference on Recent Shifts in Vegetation Boundaries of Deciduous Forests, Especially Due to General Global Warming. Birkhäuser Basel, p.15-29.
[https://doi.org/10.1007/978-3-0348-8722-9_2]
- Kong W-S (2005) Selection of Vulnerable Indicator Plants by Global Warming. Asia-Pacific Journal of Atmospheric Sciences 41: 263-273.
- Kong W-S (2007) Biogeography of Korean Plants. Geobook, Seoul.
- Koo K-A (2000) Distribution of Evergreen Broad-leaved Trees and Climatic Factors. KyungHee University
-
Koo KA, Kong W-S, Nibbelink NP, et al (2015) Potential Effects of Climate Change on the Distribution of Cold-Tolerant Evergreen Broadleaved Woody Plants in the Korean Peninsula. PLOS ONE 10:e0134043.
[https://doi.org/10.1371/journal.pone.0134043]
-
Koo KA, Kong W-S, Park SU, et al (2017a) Sensitivity of Korean fir (Abies koreana Wils.), a threatened climate relict species, to increasing temperature at an island subalpine area. Ecological Modelling 353: 5-16.
[https://doi.org/10.1016/j.ecolmodel.2017.01.018]
-
Koo KA, Park SU, Hong S, et al (2018) Future distributions of warm-adapted evergreen trees, Neolitsea sericea and Camellia japonica under climate change: ensemble forecasts and predictive uncertainty. Ecol Res 33: 313-325.
[https://doi.org/10.1007/s11284-017-1535-3]
-
Koo KA, Park SU, Kong W-S, et al (2017b) Potential climate change effects on tree distributions in the Korean Peninsula: Understanding model & climate uncertainties. Ecological Modelling 353: 17-27.
[https://doi.org/10.1016/j.ecolmodel.2016.10.007]
-
Koo KA, Park SU, Seo C (2017c) Effects of Climate Change on the Climatic Niches of Warm-Adapted Evergreen Plants: Expansion or Contraction? Forests 8: 500.
[https://doi.org/10.3390/f8120500]
- Koo K-A, Park W-K, Kong W-S (2001) Dendrochronological Analysis of Abies koreana W. at Mt. Halla, Korea: Effects of Climate Change on the Growths. The Korean Journal of Ecology 24: 281-288.
- Korea Meteorological Administration (2012) The climate atlas of Korea. Korea Meteorological Administration, Seoul.
- Korea National Arboretum (2004) Distribution maps of vascular plants of Korean Peninsula I. South coast province. Korea National Arboretum, Pocheon.
- Korea National Arboretum (2005) Distribution maps of vascular plants of Korean Peninsula II. South Province (Jeolla-do & jirisan). Korea National Arboretum, Pocheon.
- Korea National Arboretum (2006) Distribution maps of vascular plants of Korean Peninsula III. Central & South Province (Chungcheong-do). Korea National Arboretum, Pocheon.
- Korea National Arboretum (2007) Distribution maps of vascular plants of Korean Peninsula IV. Central & South Province (Gyeongsangbuk-do). Korea National Arboretum, Pocheon.
- Korea National Arboretum (2008) Distribution maps of vascular plants of Korean Peninsula V. Central Province (Geonggi-do). Korea National Arboretum, Pocheon.
- Korea National Arboretum (2009) Distribution maps of vascular plants of Korean Peninsula VI. Central Province (Gangwon-do). Korea National Arboretum, Pocheon.
- Korea National Arboretum (2010a) Distribution maps of vascular plants of Korean Peninsula VII. South Province (Gyeongsangnam-do) and Ulleung-do Province. Korea National Arboretum, Pocheon.
- Korea National Arboretum (2010b) Distribution maps of vascular plants of Korean Peninsula VIII. Jeju-do Province. Korea National Arboretum, Pocheon.
- Korea National Arboretum (2011) Distribution maps of vascular plants of Korean Peninsula IX. West & South coast Province. Korea National Arboretum, Pocheon.
-
Kwon HS, Seo CW, Park CH (2012) Development of Species Distribution Models and Evaluation of Species Richness in Jirisan region. Journal of Korean Society for Geospatial Information System 20: 11-18.
[https://doi.org/10.7319/kogsis.2012.20.3.011]
-
Landis JR, Koch GG (1977) The Measurement of Observer Agreement for Categorical Data. Biometrics 33: 159-174.
[https://doi.org/10.2307/2529310]
-
Lee BY, Nam GH, Yun JH, et al (2010) EAPDC Symposium : Biological indicators to monitor responses against climate change in Korea. Korean Journal of Plant Taxonomy 40: 202-207.
[https://doi.org/10.11110/kjpt.2010.40.4.202]
-
Lee JH, Choi BH (2010) Distribution and Northernmost Limit on the Korean Peninsula of Three Evergreen Trees. Korean Journal of Plant Taxonomy 40: 267-273.
[https://doi.org/10.11110/kjpt.2010.40.4.267]
- Lee TB (1980) Flora of Korea. Hyangmunsa, Seoul.
- Lee WC, Yim Y Plant geography with special reference to Korea. Kangwon National University press, Chuncheon
-
Lim C-H, Yoo S, Choi Y, et al (2018) Assessing Climate Change Impact on Forest Habitat Suitability and Diversity in the Korean Peninsula. Forests 9: 259.
[https://doi.org/10.3390/f9050259]
- Lim JH (Korea FRI, Woo SY (University of S, Kwon MJ (Seoul NU, et al (2006) Photosynthetic Capacity and Water Use Efficiency under Different Temperature Regimes on Healthy and Declining Korean Fir in Mt. Halla. Journal of Korean Forestry Society.
-
Miller KM, McGill BJ (2018) Land use and life history limit migration capacity of eastern tree species. Global Ecology and Biogeography 27: 57-67.
[https://doi.org/10.1111/geb.12671]
- Ministry of Environment (2006) The 3rd National Ecosystem Survey 2006 Report. Ministry of Environment, Seoul.
- Ministry of Environment (2007) The 3rd National Ecosystem Survey 2007 Report. Ministry of Environment, Seoul.
- Ministry of Environment (2008) The 3rd National Ecosystem Survey 2008 Report. Ministry of Environment, Seoul.
- Ministry of Environment (2009) The 3rd National Ecosystem Survey 2009 Report. Ministry of Environment, Seoul.
- Ministry of Environment (2010) The 3rd National Ecosystem Survey 2010 Report. Ministry of Environment, Seoul.
- Ministry of Environment (2011) The 3rd National Ecosystem Survey 2011 Report. Ministry of Environment, Seoul.
-
Namgung H, Kim M-J, Baek S, et al (2019) Predicting potential current distribution of Lycorma delicatula (Hemiptera: Fulgoridae) using MaxEnt model in South Korea. bioRxiv 557421.
[https://doi.org/10.1101/557421]
- National Geographic Information Institute (2014) The National atlas of Korea 1st Edition. HumanCultureArirang, Suwon.
-
O’Donnell MS, Ignizio DA (2012) Bioclimatic predictors for supporting ecological applications in the conterminous United States, 691st edn. U.S. Geological Survey Data Series.
[https://doi.org/10.3133/ds691]
-
Park H-C, Lee J-H, Lee G-G, Um G-J (2015) Environmental features of the distribution areas and climate sensitivity assesment of Korean Fir and Khinghan Fir. Journal of Environmental Impact Assessment 24: 260-277.
[https://doi.org/10.14249/eia.2015.24.3.260]
- Park SU, Koo KA, Kong W-S (2016a) Potential Impact of Climate Change on Distribution of Warm Temperate Evergreen Broad-leaved Trees in the Korean Peninsula. Journal of the Korean Geographical Society 51: 201-217.
-
Park SU, Koo KA, Seo C, Hong S (2017) Climate-related range shifts of Ardisia japonica in the Korean Peninsula: a role of dispersal capacity. Journal of Ecology and Environment 41:38.
[https://doi.org/10.1186/s41610-017-0055-y]
-
Park SU, Koo KA, Seo C, Kong W-S (2016b) Potential Impact of Climate Change on Distribution of Hedera rhombea in the Korean Peninsula. Journal of Climate Change Research 7: 325.
[https://doi.org/10.15531/ksccr.2016.7.3.325]
-
Pascual-Hortal L, Saura S (2006) Comparison and development of new graph-based landscape connectivity indices: towards the priorization of habitat patches and corridors for conservation. Landscape Ecol 21: 959-967.
[https://doi.org/10.1007/s10980-006-0013-z]
-
Patsiou TS, Conti E, Zimmermann NE, et al (2014) Topo-climatic microrefugia explain the persistence of a rare endemic plant in the Alps during the last 21 millennia. Global Change Biology 20: 2286-2300.
[https://doi.org/10.1111/gcb.12515]
- Pearson RG (2007) Species’ Distribution Modeling for Conservation Educators and Practitioners. Synthesis American Museum of Natural History 54-89.
- Pitelka LF, Gardner RH, Ash J, et al (1997) Plant migration and climate change. American Scientist 85: 464-473.
-
Raghavan RK, Barker SC, Cobos ME, et al (2019) Potential Spatial Distribution of the Newly Introduced Long-horned Tick, Haemaphysalis longicornis in North America. Scientific Reports 9: 498.
[https://doi.org/10.1038/s41598-018-37205-2]
-
Riahi, K., Rao, S., Krey, V., Cho, C., Chirkov, V., Fischer, G., ... & Rafaj, P. (2011). RCP 8.5—A scenario of comparatively high greenhouse gas emissions. Climatic Change, 109 (1-2), 33.
[https://doi.org/10.1007/s10584-011-0149-y]
-
Runquist RDB, Lake T, Tiffin P, Moeller DA (2019) Species distribution models throughout the invasion history of Palmer amaranth predict regions at risk of future invasion and reveal challenges with modeling rapidly shifting geographic ranges. Scientific Reports 9:2426.
[https://doi.org/10.1038/s41598-018-38054-9]
-
Taylor KE, Stouffer RJ, Meehl GA. 2012. An overview of CMIP5 and the experiment design. Bull Am Meteorol Soc 93 (4): 485.
[https://doi.org/10.1175/BAMS-D-11-00094.1]
-
Scherrer D, Körner C (2010) Infra-red thermometry of alpine landscapes challenges climatic warming projections. Global Change Biology 16: 2602-2613.
[https://doi.org/10.1111/j.1365-2486.2009.02122.x]
-
Schmidt GA, Kelley M, Nazarenko L, et al (2014) Configuration and assessment of the GISS ModelE2 contributions to the CMIP5 archive. Journal of Advances in Modeling Earth Systems 6: 141-184.
[https://doi.org/10.1002/2013MS000265]
- Secretariat of the Convention on Biological Diversity (2014) Global Biodiversity Outlook 4
- Shin M-S, Seo C, Park S-U, et al (2018) Prediction of Potential Habitat of Japanese evergreen oak (Quercus acuta Thunb.) Considering Dispersal Ability Under Climate Change. Journal of Environmental Impact Assessment 27: 291-306.
-
Song K-M, Kang Y-J, Hyeon H-J (2014) Vegetation Structure at the Slope Direction and Characteristic of Seedlings of Abies koreana in Hallasan Mountain. Journal of Environmental Science International 23: 39-46.
[https://doi.org/10.5322/JESI.2014.23.1.39]
-
Song K-M, Kim C-S, Koh J-G, et al (2010) Vegetation Structure and Distributional Characteristics of Abies koreana Forests in Mt. Halla. Journal of Environmental Science International 19: 415-425.
[https://doi.org/10.5322/JES.2010.19.4.415]
-
Song W-K, Kim E-Y (2012) A Comparison of Machine Learning Species Distribution Methods for Habitat Analysis of the Korea Water Deer (Hydropotes inermis argyropus). Korean Journal of Remote Sensing 28: 171-180.
[https://doi.org/10.7780/kjrs.2012.28.1.171]
-
Svenning J-C, Normand S, Kageyama M (2008) Glacial refugia of temperate trees in Europe: insights from species distribution modelling. Journal of Ecology 96: 1117-1127.
[https://doi.org/10.1111/j.1365-2745.2008.01422.x]
-
The HadGEM2 Development Team: G. M. Martin, Bellouin N, Collins WJ, et al (2011) The HadGEM2 family of Met Office Unified Model climate configurations. Geoscientific Model Development 4: 723-757.
[https://doi.org/10.5194/gmd-4-723-2011]
-
Thomas CD, Cameron A, Green RE, et al (2004) Extinction risk from climate change. Nature 427: 145-148.
[https://doi.org/10.1038/nature02121]
-
Thuiller W, Lafourcade B, Engler R, Araújo MB (2009) BIOMOD - a platform for ensemble forecasting of species distributions. Ecography 32: 369-373.
[https://doi.org/10.1111/j.1600-0587.2008.05742.x]
-
Thuiller W, Lavorel S, Araújo MB, et al (2005) Climate change threats to plant diversity in Europe. PNAS 102: 8245-8250.
[https://doi.org/10.1073/pnas.0409902102]
-
Vellend M, Myers JA, Gardescu S, Marks PL (2003) Dispersal of Trillium Seeds by Deer: Implications for Long-Distance Migration of Forest Herbs. Ecology 84: 1067-1072.
[https://doi.org/10.1890/0012-9658(2003)084[1067:DOTSBD]2.0.CO;2]
-
Wang R, Li Q, He S, et al (2018) Modeling and mapping the current and future distribution of Pseudomonas syringae pv. actinidiae under climate change in China. PLOS ONE 13:e0192153.
[https://doi.org/10.1371/journal.pone.0192153]
-
Wang T, Campbell EM, O’Neill GA, Aitken SN (2012) Projecting future distributions of ecosystem climate niches: Uncertainties and management applications. Forest Ecology and Management 279: 128-140.
[https://doi.org/10.1016/j.foreco.2012.05.034]
-
Watanabe S, Hajima T, Sudo K, et al (2011) MIROC-ESM: model description and basic results of CMIP5-20c3m experiments. Geoscientific Model Development Discussions 4: 1063-1128.
[https://doi.org/10.5194/gmdd-4-1063-2011]
-
Wiens JA, Stralberg D, Jongsomjit D, et al (2009) Niches, models, and climate change: Assessing the assumptions and uncertainties. PNAS 106: 19729-19736.
[https://doi.org/10.1073/pnas.0901639106]
-
Yukimoto S, Adachi Y, Hosaka M, et al (2012) A New Global Climate Model of the Meteorological Research Institute: MRI-CGCM3 —Model Description and Basic Performance—. Journal of the Meteorological Society of Japan Ser II 90A:23–64.
[https://doi.org/10.2151/jmsj.2012-A02]
- Yun J-H, Kim J-H, Oh KH, Lee BY (2011a) Distributional Change and Climate Condition of Warm-temperate Evergreen Broad-leaved Trees in Korea. Korean journal of environment and ecology 25: 47-56.
-
Yun J-H, Nakao K, Kim J-H, et al (2014) Habitat prediction and impact assessment of Neolitsea sericea (Blume) Koidz. under Climate Change in Korea. Journal of Environmental Impact Assessment 23: 101-111.
[https://doi.org/10.14249/eia.2014.23.2.101]
- Yun J-H, Nakao K, Park C-H, et al (2011b) Change Prediction for Potential Habitats of Warm-temperate Evergreen Broad-leaved Trees in Korea by Climate Change. Korean Journal of Environment and Ecology 25: 590-600.
- Yun J-H, Nakao K, Park C-H, Lee B-Y (2011c) Potential Habitats and Change Prediction of Machilus thunbergii Siebold & Zucc. in Korea by Climate Change. Korean journal of environment and ecology 25: 903-910.
-
Zhang L, Liu S, Sun P, et al (2015) Consensus Forecasting of Species Distributions: The Effects of Niche Model Performance and Niche Properties. PLOS ONE 10:e0120056.
[https://doi.org/10.1371/journal.pone.0120056]