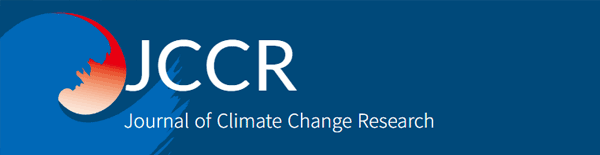
종 분포 모형을 이용한 곰솔 잠재서식지 분포 예측 결과의 정확도 평가 연구 : 앙상블 방법론의 검증을 중심으로
Abstract
Species distribution models (SDMs) are widely used for biodiversity assessment, habitat management, and climate change impact assessment due to their ability to quantitatively evaluate species distribution. However, due to model uncertainty, the use of SDMs in public policy management has been limited. In order to overcome the limitations, many studies have been conducted mainly focusing on an ensemble approach, which compensates for the uncertainty of a single model. Even though ensemble methodology has been proven to improve accuracy compared to single models, this was based on inner validation. As inner validation has established flaws, with using the data in the form of ‘point‘, the need to assess outer validation with independent data in a polygon formations has been raised. In this study, we evaluated the accuracy of a Committee Averaging (CV) ensemble methodology using outer validation. In order to minimize uncertainty beyond the methodology setting, we used Pinus thunbergii, which has spatial specificity. As the outer validation method showed more accurate evaluation results, we used outer validation indices–sensitivity, specificity and accuracy–for comparison analysis between ensemble and single model results. Single models tend to overestimate compared to ensemble models, with a high value of sensitivity and a low value of specificity, whereas ensemble models tended to decrease the spatial uncertainty of single models, with generally high values of sensitivity, specificity and accuracy. Accordingly, the ensemble model methodology proved to improve accuracy by reducing the uncertainty of single models. Furthermore, through comparison analysis between outer and inner validation results, we additionally interpreted differences and limitations among inner validation, and have finally confirmed the need for further consideration in interpreting the results of the inner validation for both methodologies. Hence, outer validation using independent data should also be used.
Keywords:
Species Distribution Model (SDM), Ensemble, Uncertainty, Validation1. 서 론
종 분포모형 (Species Distribution Model, SDM)은 종의 출현/비출현 정보와 환경변수 간의 관계를 분석하여 종의 출현 가능성을 도출하는 모형으로, 생물 종의 분포에 대한 정량적 예측이 가능하다는 특성에 따라 생물 종들의 기후변화를 포함한 서식 환경변화의 영향을 평가하는데 광범위하게 사용되고 있다 (Austin, 2002). 이러한 유용성에도 불구하고, 단일 종 분포 모형 내 서로 다른 알고리즘으로 상이한 예측 결과 및 정보가 도출됨에 따라 모형의 활용이 제한되어 왔다 (Araújo and New, 2007). 이러한 불확실성을 보완하기 위해 최근에는 합의 모형에 기반한 앙상블 방법론이 사용되고 있다 (Elith et al., 2006). 앙상블 방법론은 단일 모형의 현재 분포예측, 종 풍부도의 패턴 등의 불확실성을 개선하며 (Kwon, 2014), 이에 따라 다양한 정책 의사결정에 활용되고 있다 (Araújo et al., 2005b; Thuiller et al., 2009; Meller et al., 2014; Thuiller, 2003).
하지만, 최근 앙상블 방법론의 정확도 검증에 대한 한계가 제시됨에 따라 (Buisson et al., 2010; Marmion et al., 2009) 앙상블 방법론 평가에 대한 추가 분석의 필요성이 제기되고 있다 (Crimmins et al., 2013; Hirzel et al., 2006). 종 분포 모형 평가에 보편적으로 사용되는 내부 검증방법은, 모형 구동에 사용되는 종의 출현 자료를 단순 분할하여 도출한 예측 정밀도로 정확도를 평가한다는 점에서 한계를 가지며 (Buisson et al., 2010; Marmion et al., 2009; Dobrowski et al., 2011), 또한 검증에 사용되는 자료의 형태가 종 출현지점 (포인트)임에 따라 해당 공간 범위에서의 환경적 특성의 대표성을 반영하지 못할 수 있다는 문제점도 존재한다 (Corcoran et al., 2015). 이를 극복하기 위하여, 상이한 시간대의 독립 자료를 활용하거나, 종의 분포 범위를 반영할 수 있는 폴리곤 (polygon) 형태의 자료를 활용하는 다양한 외부 검증 방법론 및 접근 방향에 대한 연구가 진행되고 있다 (Hirzel et al., 2006). 이에 따라 앙상블 방법론의 정확도 평가에도 이러한 요소가 반영된 외부 검증방법의 적용이 필요하다.
본 연구에서는 BIOMOD2 구동을 통해 단일 및 앙상블 모형의 결과를 도출하고, 폴리곤 형태의 독립적 자료를 활용한 외부 검증을 새로이 적용함으로써 각 방법론 별 정확도를 비교·평가하고자 하였다. 방법론 설정 외의 추가적인 불확실성을 최소화하기 위해 보다 높은 모형 예측도를 도출하는 것으로 연구된 (Kadmon et al. 2003; Segurado and Araújo, 2004; Hernandez et al., 2006; Tsoar et al., 2007; Franklin et al., 2009) 국지적 분포의 국내 종, 곰솔 (Pinus thunberii)을 이용하였다. 또한, 기존의 내부 검증 결과와의 정확도 결과 비교를 통해 내부 검증 결과의 한계를 추가적으로 확인하고 그 원인을 고찰해보고자 한다.
2. 재료 및 방법
2.1 연구 대상종
곰솔 (Pinus thunbergii)은 500m 이하의 산지 및 해안에 특징적으로 분포하며 (Mirov, 1967), 한국에서는 분포 면적이 매우 좁아 출현 분포가 공간적으로 특이성을 갖는다 (Yoo et al., 2013). 곰솔은 우리나라 남부 해안가 및 남부산림권역에서 생육가능한 주요 상록침엽수종 (Yoo et al., 2013)으로, 내한성이 약하기 때문에 중부 내륙 지방과 오지에서는 생육이 불가능하지만 (Chun et al., 2014), 일부 내륙지역에 조림된 부분도 많다 (Yoo et al., 2013). 곰솔의 분포는 기온의 영향을 많이 받으며, 특히 겨울철의 저온이 그 분포에 제한을 주는 것으로 알려져 있어 (Yoo et al., 2013), 기후변화에 의해 나타나는 기온 상승은 곰솔의 분포에 영향을 미칠 것으로 예측된다 (Chun et al., 2014). 이에 따라 곰솔에 대한 보다 정확한 잠재 서식 분포 파악 및 이를 통한 양적·질적 평가와 향후 관리방안·대책 수립이 요구되고 있다 (Yoo et al., 2013). 곰솔의 출현 위치 자료 취득을 위해 제3차 전국자연환경조사 (2006-2012)자료를 활용하였으며, 총 128개의 곰솔 출현 위치 자료를 확보하였다.
2.2 연구 방법
본 연구 방법은 크게 3단계로 구성된다 (Fig. 1). 먼저 선행연구 검토를 통해 대상 종의 출현 분포 및 서식에 영향을 주는 환경 변수를 구축하고, 활용하고자 하는 앙상블 방법론을 선정하였다. 이후, BIOMOD2내 단일·앙상블 모형을 통해 곰솔의 잠재서식 분포를 예측하였으며, 예측에 활용되지 않은 폴리곤 형태의 독립 자료를 활용한 외부 검증 방법을 새롭게 적용하여 각 방법론에 대한 정확도를 비교 평가하였다. 또한, 각 방법론 별 기존의 내부 검증 결과와 새롭게 적용한 외부 검증 결과와의 비교를 통해 결과 차이에 대한 추가적인 분석을 진행하였다.
곰솔의 서식에 영향을 주는 환경 변수 자료 구축을 위해 여러 선행연구를 참고하여 30-Arc second (1 km2) 해상도의 기후·지형·토양 변수를 선정하였다 (Kim and Bong, 1983; Kwon et al., 2012; Kim et al., 2015; Seok et al., 2014; Lee et al., 2006; Chun and Lee, 2013; Hong et al., 2006). 변수들의 다중공선성 (multicollinearity)을 제거하기 위해 변수들 간 상관관계 분석을 진행하였다 (Fortin and Dale, 2014). 분석 결과, 상관관계가 0.7보다 높은 변수들은 제외하였으며 (Park et al., 2016), 일부 변수 중 문헌 고찰을 통해 곰솔의 분포에 있어 중요하다고 판단되는 변수는 포함 하여 환경변수를 최종 구축하였다 (Table 1).
현재의 기후자료 구축을 위해 Worldclim-Global climate Data (www.worldclim.org)에서 제공하는 1970-2000년의 생물기후적 변수 (Bioclimatic Variables, Bioclim) 자료를 활용하였다. 생물기후적 변수들은 생물의 분포 및 성장에 영향을 주는 기후요소들로 구성된 19개의 변수로, 이를 활용하여 생물종의 분포와 기후요소와의 관계를 찾는 다양한 연구가 진행되고 있다 (Park et al., 2016). 본 연구에서는 19개의 변수 중, 곰솔의 서식에 영향을 주며, 식물의 생장과 서식지 적합도를 설명함과 동시에 다른 변수들을 대표할 수 있는 기온변수 (Bio2,3,11)와 강수변수 (Bio12,13,14)를 사용하였다 (Koo et al., 2015). 또한, 곰솔의 수고생장과 기상인자에서 상대습도가 중요한 인자로 판명됨에 따라 (Son and Chung, 1994), 상대습도 변수에 대한 대체변수로 CGIAR-CSI (www.cgiar-csi.org)에서 제공하는 Global-PET (Global Potential Evapo-Transpiration)를 사용하였다 (Trabucco and Zomer, 2009). 지형자료의 경우, 환경공간정보서비스 (http://egis.me.go.kr)에서 제공하는 고도, 향, 경사 자료를 이용하였다. 이 중 고도 변수는 Bio2와 0.7보다 높은 상관성을 보였으나, 고도와 같은 지형 인자는 미기후환경에 영향을 주어 식물의 생육과 직접적인 관련이 있는 만큼 (Lee and Lim, 2002), 그 중요성이 높은 것으로 판단되어 최종 변수로 포함시켰다. 토양자료는 ISRIC (data.isric.org)에서 제공하는 토양 pH와 유기탄소함량 변수를 사용하였으며, 유기탄소함량의 경우 유기물 함량에 대한 대체변수로 사용되었다.
본 연구에서 사용한 BIOMOD2 모형 내부에는 통계 기반의 Generalized Linear Models (GLM), Generalized Additive Models (GAM), Multivariate Adaptive Regression Splines (MARS)와 기계학습 기반의 Classification Tree Analysis (CTA), Flexibel Discriminant Analysis (FDA), Artificial Neural Networks (ANN), Generalized Boosted Models (GBM), Random forest (RF), Surface Range Envelope (SRE), Maxent (Maximum entropy algorithm)로 총 10가지의 모델이 존재하며, 이 모델을 한 번에 구동할 수 있다. MaxEnt와 SRE 모델을 제외한 나머지 8개의 모델은 모두 종의 출현-비출현 형태의 이항형 자료가 필요한 모델로, 출현자료만을 사용하는 모델보다 정확도가 높다 (Elith et al., 2006). 이에 따라 본 연구에서는 8가지 모델 (GLM, GAM, MARS, CTA, FDA, ANN, GBM, RF)을 이용하여 종 분포 모델링을 수행하였다. 하지만, 전국자연환경조사 자료는 출현자료만을 포함한 자료이므로, 출현-비출현 형태의 이항형 입력 자료를 구축하기 위해선 의사 비출현 자료 (pseudo-absence data)를 생성하여 적용해야한다 (Kwon, 2014). 선행 연구 검토에 따라 (Barbet Massin et al., 2012), 출현 자료와 동일한 개수의 임의 비출현 자료를 임위 표본화 하는 방법으로 10회 반복하여 모형을 구현하였다.
본 연구에서 활용한 Committee Averaging (CV) 앙상블 방법론은, 높은 정확도로 선정된 단일 종 분포 모형의 연속 확률분포 결과를 출현/비출현의 형태로 변환한 후 중첩하여 단일 평균 결과로 도출한다 (Meller et al., 2014; Hao et al., 2019). 각 단일 모형 결과를 이항 분포 형태로 변환하기 위해서는 종의 출현 여부를 결정짓는 임계점을 이용한다. 임계점 설정 값에 따라 각 모델 별 잠재 서식 가능 분포에서의 차이가 유발되므로 (Grenouillet et al., 2011), 각 모형의 정확도 측면에서도 적절한 임계점 설정은 매우 중요하다. 많은 연구에서는 모형에 의해 예측된 종의 출현이 실제 관찰과 얼마나 일치하는지를 보여주는 출현 정확도 (Sensitivity)와 모형에 의해 예측된 종의 비출현이 실제 관찰되지 않은 곳과 얼마나 일치하는지를 보여주는 비출현 정확도 (Specificity)의 합이 최대이거나 차이가 최소가 되는 지점, 즉 TSS (True skill statistics)값이 최대가 되는 지점을 임계점으로 설정하여 연구를 진행하고 있다 (Liu et al., 2013). 본 연구에서 사용한 Committee Averaging 방법론 또한 위의 임계점을 적용하였으며, 단일 모형 결과 중 TSS 값이 0.8보다 높은 모형을 선별하여 앙상블 결과를 도출하였다 (Gallien et al., 2012).
CV 방법론은 앙상블 결과를 다양한 단일 모형의 평균 출현확률이 아닌, 단일 모형 결과 간의 종 출현 일치율 (a percentage of agreement)로 도출했다는 점에서 의미가 있다 (Gallien et al., 2012). 또한, 서로 다른 의미 및 범위의 변이를 포함하고 있는 연속적 확률 분포 결과물을 일괄적으로 통일 및 통합할 수 있다는 장점이 있다. 더 나아가 여러 임의 비출현 자료를 사용할 때 생길 수 있는 편향을 최소화할 수 있어 (Gallien et al., 2012), 임의 비출현 자료를 생성해야하는 다양한 종 분포 모형을 활용 시 용이하다.
본 연구에서는 예측에 활용되지 않은 폴리곤 형태의 독립 자료를 활용하는 외부 검증 (outer validation) 방법을 새롭게 적용하여 단일 및 앙상블 방법론 결과 간 정확도 비교를 진행하였다. 외부 검증의 경우, 제2, 3차 전국 자연환경 조사 (1997-2003, 2006-2012) 결과를 토대로 최근 항공 영상이 반영되어 작성된 현존식생도 (국토환경정보센터, www.neins.go.kr)내 폴리곤 형태의 곰솔 분포를 독립자료로 활용하였다 (Appendix 2 (b)). 이로써 기존 포인트 형태의 곰솔의 공간적인 분포 범위를 보다 잘 반영할 수 있는 데이터 형태 (폴리곤)를 활용한 외부 검증을 수행하였다. 외부 검증을 통한 정확도 평가는 혼동 행렬 (Confusion Matrix)1) 을 통해 진행되었으며, 각 방법론 별 출현 정확도 (Sensitivity), 비출현 정확도 (Specificity), 정확도 (Accuracy) 지수2)를 각각 계산하여 비교 분석을 진행하였다 각 지수를 계산하기 위해선 각 모형 결과를 출현/비출현의 이항 분포 형태로 변환해야 하며, 임계점의 설정에 따라 각 결과가 상이하게 도출됨에 따라 본 연구에서는 종 출현 확률의 중앙값 500 (0-1000)을 임계점으로 고정하여 일괄적으로 설정하였다 (Song and Kim, 2012). 각 지수 별 정확도 평가 기준의 경우, 정확도 지수는 0.8이상을 높다고 해석하였으며 (Ryu et al., 2017), 출현 정확도와 비출현 정확도 지수는 0.5 이하일 때 모형의 분류 성능이 없는 것으로 해석됨에 따라, 0.5를 기준 값으로 설정하여 정확도를 평가하였다 (Song, 2018).
기존의 내부 검증 방법 (inner validation)의 경우, 종 분포 모형 개발에 사용되는 포인트 형태의 출현 자료를 훈련자료 (training data)와 시험자료 (test data)로 80대 20으로 나누어 5회 반복 실시하는 것으로 진행되었다. 또한 앙상블 방법론의 경우, 단일 모형 예측 정확도 검증에 사용한 교차검증 자료를 동일하게 사용하여 모형 결과별 공정한 비교 분석을 진행하였다 (Thuiller, 2016). 내부 검증의 모형 예측 정확도 분석에는 대표적으로 ROC (Receiver operation characteristic analysis)의 AUC (Area under the curve)값과 TSS (True skill statistics) 값이 있다 (Landis and Koch, 1977; Pearson, 2010). 이 중 TSS값이 출현과 비출현 자료에 대한 정확도를 모두 포함하고, 출현과 비출현에 대한 비율에 영향을 받지 않음과 동시에, AUC와 달리 대상종의 분포 면적 및 형태에 영항을 받지 않는 장점을 가지고 있어 종 분포모형의 검증에 많이 사용되고 있다 (Allouche et al., 2006). 이에 본 연구에서는 내부 검증의 모형 예측 정확도 분석에 TSS 값을 평가에 활용하였다. TSS 계수 값은 0.4-0.6 까지는 일치정도가 보통을 나타내며, 0.6-0.7은 높음, 0.7이상은 거의 일치함을 나타낸다 (Franklin, 2009). 본 연구에서는 TSS 0.6을 기준 값으로 모형 결과의 정확도를 평가하였다.
3. 결과 및 고찰
3.1 곰솔의 잠재서식지 예측
8개의 개별 단일 모형 방법론 및 CV 앙상블 모형 방법론 구동을 통해, 각 방법론 별 곰솔의 잠재서식지를 예측하였다. 단일 모형의 경우, 각 개별 모형 별 50개의 결과 세트를 평균 내어 잠재서식지를 도출하였으며, 앙상블의 경우 도출된 총 50개의 결과 세트 중 TSS값이 0.8보다 큰 단일 모형이 적어도 한 개 존재하여 앙상블이 진행될 수 있는 25개의 세트 (Ensemble 1 ~ Ensemble 25)를 최종 도출하여 (Appendix 1) 각 잠재 서식지를 도출하였다.
잠재서식지 추정 결과, 단일 모형과 앙상블 모형 모두 생태권역 중 해안도서권역에 집중적으로 나타났으며 (Fig. 2 (a), Fig. 3), 이는 곰솔 수종의 특성상 분포 지역이 제한적인 것을 적절히 반영한 결과로 해석된다 (Fig. 2 (b)). 다만, 대부분의 단일 모형의 경우 곰솔의 적지 분포로 분류되는 남동산야권역·남서산야권역 (Fig. 2 (b); Chun et al., 2014; Kim et al., 2016) 외 추가적으로 산림권역 및 중부산야권역으로까지 분포가 과대하게 예측되어 도출된 반면, 앙상블 모형은 대부분 남동산야권역·남서산야권역 범위까지 분포가 예측되어 도출되었다 (Fig. 3).
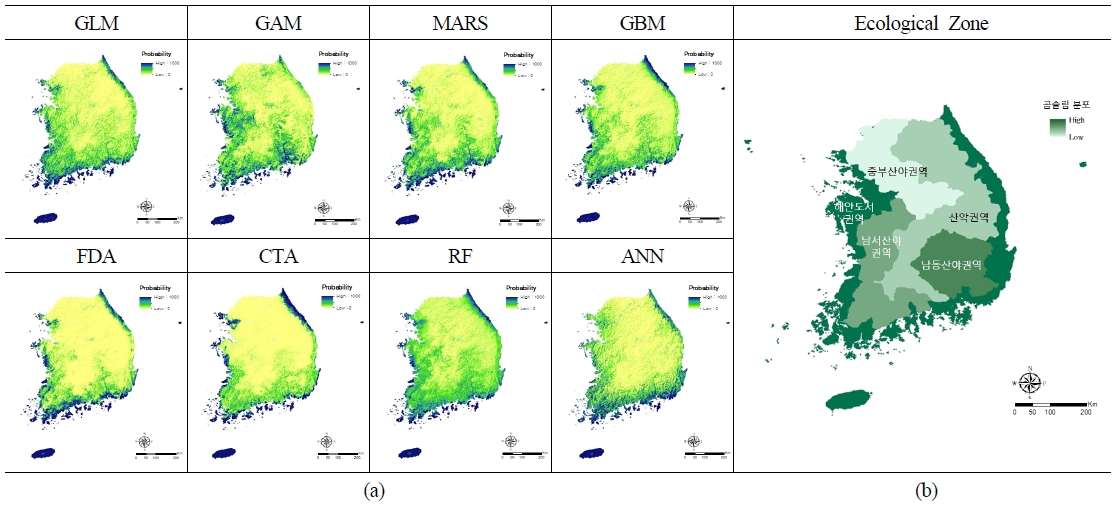
(a) Potential Habitat Distribution results of Single Model (b) Distribution of Pinus Thunbergii by Ecological Zone (Kim et al., 2016).
이러한 단일 및 앙상블 모형 방법론 별 잠재서식지 분포간의 불일치는, 단일 모형 방법론의 과대추정과 같은 불확실성 때문인 것으로 판단되며, 이에 대한 수치적 비교를 위해 정확도 평가가 수행되었다.
3.2 단일 및 앙상블 방법론 정확도 비교 분석
단일 모형과 앙상블 모형 결과 간의 정확한 비교를 위해 외부 검증 결과로 도출된 총 3가지 정확도 지수- 출현 정확도, 비출현 정확도와 정확도 지수 - 를 모두 분석하였다.
출현 정확도 지수의 경우, 앙상블 모형이 평균 0.64로 기준 값 0.5보다는 높지만, GAM (0.55)과 CTA (0.62) 모형을 제외한 모든 단일 모형보다 낮게 나타났다 (Fig. 4 (a)). 이 결과는, 종 출현 확률 값이 단일 모형 결과의 중첩 정도로 나타나 불연속 값으로 도출되는 앙상블 모형과 달리, 단일 모형의 확률 값은 연속적으로 나열된 값임과 동시에 그 수치 값이 다양하고 개수 또한 많아 잠재 서식 가능 분포 면적이 상대적으로 넓게 도출되는 특성이 반영 된 것으로 보인다 (Fig. 2 (a) and Fig. 3). 단일 모형 중에서는 FDA 모형이 0.7로 가장 높은 예측 정확도를 나타냈다.
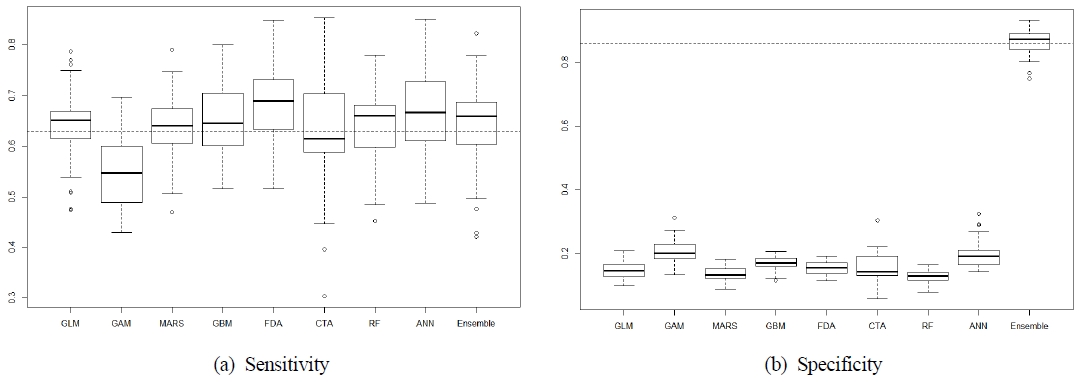
(a) Sensitive of single·ensemble models (Average value of ensemble, 0.64) (b) Specificity of single·ensemble models (Average value of ensemble, 0.87).
반면, 비출현 정확도 지수의 경우 앙상블 모형이 평균 0.87로 기준 값 0.5보다 높게 나타났으며, 모든 단일 모형보다 높게 분석되었다 (Fig. 4 (b)). 모형의 분류 성능의 기준 0.5보다 낮은 정확도를 나타낸 모든 단일 모형의 결과는, 앞서 출현 정확도에서 보였던 경향과 유사하게 각 단일 모형별로 모형 구동에 영향을 미치는 공간 변수의 차이에 따라 잠재 서식 분포가 과도하게 도출되는 경향 (Thuiller et al., 2004; Arau’jo et al., 2005a; Pearson et al., 2006; Elith and Graham, 2009; Miller, 2014)이 반영된 것으로 보인다. 반면 앙상블 방법론이 평균적으로 모든 단일 모형보다 평균 4배 이상의 비출현 정확도 값을 나타내어, 각 단일 모형의 과도 추정 불확실성을 가시적으로 저감시킨 것으로 해석할 수 있다 (Appendix 2 (a) and Appendix 3).
최종 정확도 분석 결과, 앙상블 모형이 평균 0.86으로 RF (0.87) 모형을 제외한 모든 단일 모형보다 높게 나타났다 (Fig. 5). 이 결과는, 앞서 설정된 기준값을 통해 변환된 이항 분포 형태의 잠재서식지 예측 결과와 외부 검증에 사용된 독립 자료 간의 폴리곤 기반 비교에 따른 출현·비출현 정확도를 모두 고려했을 때, 앙상블 모형이 대부분의 단일 모형 (8개 모형 중 7개)보다 높은 정확도 결과를 도출한 것으로 해석되며, 이는 앙상블 방법론이 각 단일 종 분포 모형의 평균 오차를 저감하여 정확도를 향상시킨다는 선행 연구 결과와 유사한 결과이다 (Crossman and Bass, 2008; Marmion et al., 2009; Araújo and New, 2007).
3.3 내·외부 검증 결과 비교 분석
단일 종 분포 모형의 내부 검증 분석 결과, 도출된 8개 모형의 평균 TSS값이 모두 기준 값 0.6 이상을 나타냈다 (Fig. 6). 외부 검증 결과 또한 8개 모형의 평균 정확도 (Accuracy) 모두 기준 값 0.8이상을 나타내었으며, 이에 따라 단일 모형의 경우 포인트 형태로 검증이 이루어진 내부 검증과 폴리곤 형태로 검증이 이루어진 외부 검증 결과가 모두 유사하게 높은 정확도를 나타냈다 (Fig. 6).
앙상블 모형 구축을 위해, 도출된 총 50개의 결과 세트 중 TSS값이 0.8보다 큰 단일 모형이 적어도 한 개 존재하여 앙상블이 진행될 수 있는 25개의 세트 (Ensemble 1 ~ Ensemble 25)를 최종 도출했다 (Appendix 4). 내부 검증 결과, Ensemble 4~14에서는 TSS값이 0이었으며, 나머지 일부 결과에서도 기준 값 0.6보다 낮은 정확도가 나타났다 (Table 2). 이 결과는 앞서 높은 정확도 (> 0.8)의 단일 모형 결과물을 선정하여 앙상블 한 결과의 정확도로는 적합하지 않다. 반면 외부 검증의 경우, 거의 대부분의 앙상블 결과 (25개 중 23개)에서 기준 값 0.8이상의 정확도 (Accuracy) 지수 값이 도출되어, 앙상블 결과가 대체적으로 높은 정확도를 나타낸 것으로 최종 분석되었다 (Table 2).
이와 같은 내·외부 검증 결과 간의 차이는 CV 앙상블 방법론의 원리 및 대상 종인 곰솔의 출현 분포 특성 및 내·외부 검증 각각에 사용된 자료 형태의 차이에 기인한 것으로 해석할 수 있다. CV 방법론을 통해 도출된 종의 잠재적 서식 분포 결과는 앞서 선정된 단일 모형 결과의 이항 분포 형태 (출현/비출현)의 중첩이므로, 확률 수치 값의 개수가 단일 모형 결과에 비해 적으며 불연속적이다. 즉, 8개의 각 단일 모형 결과에서의 확률 수치 값의 평균 개수가 941개 (최소: 812, 최대: 1001)인 것을 고려하면, 앙상블에서 도출될 수 있는 (ex> Appendix 1, Ensemble 10) 최대 8개의 확률 수치 값은 확연히 적은 값이다. 또한, 대상 종으로 사용한 곰솔은 그 생태적 특성에 따라 분포가 남부 해안 쪽으로 치우쳐 있어 비출현의 공간적 영역 면적이 출현 면적보다 넓다 (Appendix 2 (b)). 이와 같은 출현 분포 특성이 앙상블 결과의 종 출현 확률 수치 값 개수를 더 줄인 것으로 보이며, 결과적으로 앙상블 결과의 내부 검증에 다양한 확률 수치 값이 사용되지 않게 된 것으로 보인다. 더불어, 상대적으로 적은 범위에서의 값만을 포함한 검증을 진행하는 포인트 형태의 내부 검증 방법론이 함께 작용함에 따라 적절한 출현 정확도·비출현 정확도 및 임계점이 도출되지 않았다. 이에 따라 외부 검증 결과와의 차이가 크게 나타난 것으로 해석된다.
이와 같은 연구 결과에 따라, 국지적으로 분포하는 종을 대상으로 CV 앙상블 모형을 구동할 때에는 내부 검증 결과 해석에 유의해야하며, 독립적임과 동시에 환경 대표성을 보다 잘 반영할 수 있는 폴리곤 형태의 자료를 활용한 외부 검증이 추가적으로 수행되어야 한다.
4. 결론
본 연구에서는 단일 및 앙상블 종 분포 모형 간 정확도 평가를 위해 예측에 활용되지 않은 폴리곤 형태의 독립 자료를 활용하는 외부 검증 (outer validation) 방법 내 정확도 (Accuracy)·출현 정확도 (Sensitivity)·비출현 정확도 (Specificity) 지수를 새로이 적용하여 비교 분석을 수행하였으며, 더 나아가 기존의 내부 검증 결과와의 비교를 통해 내부 검증 결과의 한계 및 원인을 고찰하였다.
외부 검증 결과, 출현 정확도에서는 단일 모형이 앙상블 모형보다 대체적으로 (8개의 모형 중 6개) 높은 값을 보인 반면, 비출현 정확도에서는 모든 모형이 앙상블 모형보다 확연히 낮은 값을 보였다. 출현, 비출현 정확도를 모두 반영한 정확도 지수에서 또한 앙상블 모형이 단일 모형보다 높은 값을 나타냄으로서 (RF 제외), 앙상블 방법론이 단일 방법론 모형의 과대 추정 불확실성을 줄이고 보다 높은 정확도를 나타냄을 확인하였다. 내부 검증 방법론 적용 결과를 분석해본 결과 환경적 대표성을 적절히 반영하지 못하는 포인트 형태의 검증 자료를 사용함에 따라, 공간적 특이성을 나타내며 출현/비출현의 분포가 불균형하게 나타나는 대상 종 및 CV 앙상블 방법론 결과에 적용하는 데 한계가 있음을 확인하였다.
본 연구 결과는 앙상블 모형이 단일 모형의 불확실성을 저감하여 정확도를 향상시킨 것을 외부 검증 방법을 통해 확인 및 평가한 것에 의의가 있다. 또한, 각 방법론 별 검증 데이터의 형태가 다른 내·외부 검증 결과 간 차이 비교 분석을 통해 내부 검증의 한계 및 이에 따른 유의점을 제시함으로써, 종 분포 모형 결과의 정확도 평가 방법론 및 사용되는 대상 종·앙상블 방법론에 따른 적절 검증 방법론 적용 필요성을 제시했다. 향후 연구에서는 추가적인 정확도 지표 개발 및 이를 반영하여 외부 검증 방법론을 고도화하고자하며, 더 나아가 각 모델 별 잠재 서식지 결과가 내·외부 검증 모두에 기본이 되는 자료인 만큼, 입력 값인 생물 좀 분포 자료의 수를 늘려 그 정확도 또한 높이고자 한다.
Acknowledgments
본 결과물은 환경부의 재원으로 한국환경산업기술원의 “화학사고대응환경기술개발사업 (No. 2016001970001)” 및 “훼손 유형별 생태복원사업 모델 개발 및 평가 체계 구축 사후관리 기술개발 (No. 2018000210006)”의 지원을 받아 연구되었습니다.
Notes
References
-
Allouche O, Tsoar A, Kadmon R. 2006. Assessing the accuracy of species distribution models: Prevalence, kappa and the true skill statistic (TSS). J Appl Ecol 43 (6): 1223-1232.
[https://doi.org/10.1111/j.1365-2664.2006.01214.x]
-
Arau’jo MB, Robert JW, Richard JL, Markus E. 2005a. Reducing uncertainty in projections of extinction risk from climate change. Global Ecol. Biogeogr. 14: 529-538.
[https://doi.org/10.1111/j.1466-822X.2005.00182.x]
-
Araújo MB, New M. 2007. Ensemble forecasting of species distributions. Trends Ecol. Evol 22: 42-47.
[https://doi.org/10.1016/j.tree.2006.09.010]
-
Araújo MB, Whittaker R.J, Ladle RJ, Erhard M. 2005b. Reducing uncertainty in projections of extinction risk from climate change. Global Ecology and Biogeography 14: 529-538.
[https://doi.org/10.1111/j.1466-822X.2005.00182.x]
-
Austin M. 2002. Spatial prediction of species distribution: An interface between ecological theory and statistical modelling. Ecological Modelling 157 (2): 101-118.
[https://doi.org/10.1016/S0304-3800(02)00205-3]
-
Barbet-Massin M, Jiguet F, Albert CH, Thuiller W. 2012. Selecting pseudo‐absences for species distribution models: how, where and how many?. Methods in Ecology and Evolution 3 (2): 327-338.
[https://doi.org/10.1111/j.2041-210X.2011.00172.x]
-
Buisson L, Thuiller W, Casajus N, Lek S, Grenouillet G. 2010. Uncertainty in ensemble forecasting of species distribution. Global Change Biology 16 (4): 1145–1157
[https://doi.org/10.1111/j.1365-2486.2009.02000.x]
-
Chun JH, Lee CB. 2013. Assessing the Effects of Climate Change on the Geographic Distribution of Pinus densiflora in Korea using Ecological Niche Model in Korean with English abstract. Korean Journal of Agricultural and Forest Meteorology 15 (4): 219-233.
[https://doi.org/10.5532/KJAFM.2013.15.4.219]
- Chun JH, Shin MY, Kwon TS, Lim JH, Lee YK, Park GE, Kim TW, Seong JH. 2014. Predicting the Changes of Productive Areas for Major Tree Species under Climate Change in Korea. Korea Forest Research Institute.
-
Corcoran J, Knight J, Pelletier K, Rampi L, Wang Y. 2015. The effects of point or polygon based training data on RandomForest classification accuracy of wetlands. Remote Sensing, 7 (4): 4002-4025.
[https://doi.org/10.3390/rs70404002]
-
Crimmins SM., Dobrowski SZ, Mynsberge AR. 2013. Evaluating ensemble forecasts of plant species distributions under climate change. Ecological modelling 266: 126-130.
[https://doi.org/10.1016/j.ecolmodel.2013.07.006]
-
Crossman ND, Bass DA. 2008. Application of common predictive habitat techniques for post border weed risk management. Diversity and Distributions 14: 213-224.
[https://doi.org/10.1111/j.1472-4642.2007.00436.x]
-
Dobrowski SZ, Thorne JH, Greenberg JA, Safford HD, Mynsberge AR., Crimmins SM, Swanson AK. 2011. Modeling plant ranges over 75 years of climate change in California, USA: temporal transferability and species traits. Ecological Monographs 81 (2): 241-257.
[https://doi.org/10.1890/10-1325.1]
-
Elith J, Graham C.H, Anderson R.P, Dudik M, Ferrier S, Guisan A .et al. 2006. Novel methods improve prediction of species’ distributions from occurrence data. Ecography 29: 129-151.
[https://doi.org/10.1111/j.2006.0906-7590.04596.x]
-
Elith, J, Graham CH. 2009. Do they? How do they? WHY do they differ? on finding reasons for differing performances of species distribution models. Ecography, 32 (December 2008): 66-77.
[https://doi.org/10.1111/j.1600-0587.2008.05505.x]
- Fortin M., Dale MR. 2014. Spatial Analysis a guide for ecologist, Cambridge University Press.
-
Franklin J, 2009. Mapping species distributions spatial inference and prediction, 1st ed New York, Cambridge University Press.
[https://doi.org/10.1017/CBO9780511810602]
-
Franklin J, Wejnert KE, Hathaway SA, Rochester CJ, Fisher RN. 2009. Effect of species rarity on the accuracy of species distribution models for reptiles and amphibians in southern California. Divers. Distrib 15: 167-177.
[https://doi.org/10.1111/j.1472-4642.2008.00536.x]
-
Gallien L, Douzet R, Pratte S, Zimmermann NE, Thuiller W. 2012. Invasive species distribution models-how violating the equilibrium assumption can create new insights. Global Ecology and Biogeography 21 (11): 1126-1136.
[https://doi.org/10.1111/j.1466-8238.2012.00768.x]
-
Grenouillet G, Buisson L, Casajus N, Lek S. 2011. Ensemble modelling of species distribution: the effects of geographical and environmental ranges. Ecography 34 (1): 9-17.
[https://doi.org/10.1111/j.1600-0587.2010.06152.x]
-
Hao T, Elith J, Guillera‐Arroita G, Lahoz‐Monfor, JJ. 2019. A review of evidence about use and performance of species distribution modelling ensembles like BIOMOD. Diversity and Distributions 25 (5): 839-852.
[https://doi.org/10.1111/ddi.12892]
-
Hernandez PA, Graham CH, Master LL, Albert DL. 2006. The effect of sample size and species characteristics on performance of different species distribution modeling methods. Ecography 29: 773-785.
[https://doi.org/10.1111/j.0906-7590.2006.04700.x]
-
Hirzel AH, Le Lay G, Helfer V, Randin C, Guisan A. 2006. Evaluating the ability of habitat suitability models to predict species presences. Ecological modelling 199 (2): 142-152.
[https://doi.org/10.1016/j.ecolmodel.2006.05.017]
- Hong SK, Park JW, Yang HS. 2006. Ecological Characteristics of Black Pine Forest as Ecotourism Resource - Jeungdo, Shinan-gun, Jeonnam. Journal of the Island Culture 28: 223.244.
-
Kadmon R, Farbor O, Danin A. 2003. A systematic analysis of factors affecting the performance of climatic envelope models. Ecol. Appl 13: 853-867.
[https://doi.org/10.1890/1051-0761(2003)013[0853:ASAOFA]2.0.CO;2]
- Kim OS, Lee JS, Kim JB, Lim JH, Lee CS. 2016. Preservation and Management System of Pine·Pine Forests in Korea. NIFOS. 1: 73-94
-
Kim TG, Cho YH, Oh JG. 2015. Prediction Model of Pine Forests’ Distribution Change according to Climate Change in Korean with English abstract. Korean Journal of Ecology and Environment 48 (4): 229-237.
[https://doi.org/10.11614/KSL.2015.48.4.229]
- Kim, JU, Kil BS. 1983. A study on the distribution of Pinus thunbergii in the Korean Peninsula in Korean with English abstract. The Korean Journal of Ecology 6 (1): 45-54.
-
Koo KA, Kong W, Nibbelink NP, Hopkinson CS, Lee JH. 2015. Potential effects of climate change on the distribution of cold tolerant evergreen broadleaved woody plants in the Korean peninsula. PloS one 10 (8): e0134043.
[https://doi.org/10.1371/journal.pone.0134043]
-
Kwon HS, Ryu JE, Seo CW, Kim JY, Tho JH, Suh MH, Park CH. 2012. Climatic and Environmental Effects on Distribution of Narrow Range Plants in Korean with English abstract. Journal of the Korea Society of Environmental Restoration Technology 15 (6): 17-27.
[https://doi.org/10.13087/kosert.2012.15.6.017]
- Kwon HS. 2014. Applying Ensemble Model for Identifying Uncertainty in the Species Distribution Models in Korean with English abstract. Journal of the Korean Society for Geospatial Information System 22 (4): 47-52.
-
Landis JR, Koch GG. 1977. The measurement of observer agreement for categorical data. Biometrics, p.159-174.
[https://doi.org/10.2307/2529310]
- Lee CS, Lee WK, Yoon JH, Song CC. 2006. Distribution Pattern of Pinus densiflora and Quercus Spp. Stand in Korea Using Spatial Statistics and GIS in Korean with English abstract. Journal of Korean Forestry Society 95 (6): 663-671.
- Lee WC, Lim YJ. 2002. Plant Geography. Kangwon National University.
-
Liu C, White M, Newell G. 2013. Selecting thresholds for the prediction of species occurrence with presence only data. Journal of biogeography 40 (4): 778-789.
[https://doi.org/10.1111/jbi.12058]
-
Marmion M, Luoto M, Heikkinen RK, Thuiller W. 2009. The performance of state-of-the-art modelling techniques depends on geographical distribution of species. Ecol. Model. 220: 3512-3520.
[https://doi.org/10.1016/j.ecolmodel.2008.10.019]
-
Meller L, Cabeza M, Pironon S, Barbet-Massin M, Maiorano L, Georges D, Thuiller W. 2014. Ensemble distribution models in conservation prioritization: from consensus predictions to consensus reserve networks. Diversity and distributions 20 (3): 309-321.
[https://doi.org/10.1111/ddi.12162]
-
Miller JA. 2014. Virtual species distribution models: Using simulated data to evaluate aspects of model performance. Progress in Physical Geography, 38 (1): 117-128.
[https://doi.org/10.1177/0309133314521448]
- Mirov. NT. 1967. The genus pinus. The Ronald Press Company, p.602.
-
Park SU, Koo KA, Seo CW, Kong WS. 2016. Potential Impact of Climate Change on Distribution of Hedera rhombea in the Korean Peninsula in Korean with English abstract. Journal of Climate Change Research 7 (3): 325-334.
[https://doi.org/10.15531/ksccr.2016.7.3.325]
-
Pearson RG, Thuiller W, Arau’jo MB, Martinez-Meyer EB, Lluls M, Colin M., Lera S, Pedro D, Terence P, Lees DC. 2006. Model-based uncertainty in species range prediction. Journal of Biogeography, 33: 1704-1711.
[https://doi.org/10.1111/j.1365-2699.2006.01460.x]
- Pearson RG. 2010. Species’ distribution modeling for conservation educators and practitioners. Lessons in Conservation 3: 54-89.
- Ryu JE, Hwang JH, Lee JH, Chung HI, Lee KI, Choi YY, Zhu YY, Sung MJ, Jang RI, Sung HC, Jeon SW, Kang JY. 2017. Analysis of Changes in Forest According to Urban Expansion Pattern and Morphological Features in Korean with English abstract. Korean Journal of Remote Sensing 33 (5): 835-854.
-
Segurado P, Araújo MB. 2004. An evaluation of methods for modelling species distributions. J. Biogeogr 31: 1555-1568.
[https://doi.org/10.1111/j.1365-2699.2004.01076.x]
- Seok YS, Song KH, Chon JH. 2014. The Application of the Systems Thinking Approach in Suggesting the Restoration Direction of the Ecological Landscape Protected Area - Focused on the Relationship between the Damage Causes of Hasidong·Anin Coastal Dune and Keystone Species- in Korean with English abstract. Journal of East Asian Landscape Studies 8 (2): 43-55.
- Son YM, Chung YG. 1994. The Effects of the Topographical, Soil and Metetological Factors on the Tree Height Growth in the Pinus thunbergii Stands in Korean with English abstract. Journal of Korean Forestry Society 83 (3): 380-390.
-
Song SW. 2018. Assess the Accuracy of Diagnostic Tools. Korean Journal of Family Practice 8 (1): 1-2.
[https://doi.org/10.21215/kjfp.2018.8.1.1]
-
Song WK, Kim EY. 2012. A Comparison of Machine Learning Species Distribution Methods for Habitat Analysis of the Korea Water Deer (Hydropotes inermis argyropus) in Korean with English abstract. Korean Journal of Remote Sensing 28 (1): 171-180.
[https://doi.org/10.7780/kjrs.2012.28.1.171]
- Thuiller W, Georges D, Engler R, Breiner F, Georges M. D, Thuiller CW. 2016. Package ‘biomod2’. Species distribution modeling within an ensemble forecasting framework https://CRAN.R-project.org/package=biomod2, .
-
Thuiller W, Lafourcade B, Engler R., Araujo MB. 2009. BIOMOD - a platform for ensemble forecasting of species distributions. Ecography 32 (3): 369-373.
[https://doi.org/10.1111/j.1600-0587.2008.05742.x]
- Thuiller W, Miguel B, Arau’jo MB, Richard G, Pearson, Robert JW, Lluis B, Sandra L. 2004. Biodiversity conservation: uncertainty in predictions of extinction risk. Nature 427: 145-148.
-
Thuiller W. 2003. BIOMOD - optimizing predictions of species distributions and projecting potential future shifts under global change. Global Change Biology 9 (10): 1353-1362.
[https://doi.org/10.1046/j.1365-2486.2003.00666.x]
- Trabucco A, Zomer RJ. 2009. Global aridity index (global-aridity) and global potential evapo-transpiration (global-PET) geospatial database. CGIAR Consortium for Spatial Information.
-
Tsoar A, Allouche O et al. 2007. A comparative evaluation of presence only methods for modelling species distribution. Divers. Distrib. 13: 397-405.
[https://doi.org/10.1111/j.1472-4642.2007.00346.x]
- Yoo BO, Lee KS et al. 2013. Pinus thunbergii Resources and Forest Management in the South Forest Area. Korea Forest Research Institute.