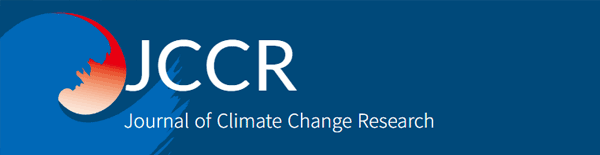
쇠퇴지역 도시회복력 평가지표 도출을 위한 기초 연구 : 정책 네트워크 분석 및 Fuzzy 다기준 의사결정법의 활용
Abstract
With the increase in disasters and damage caused by climate change, the global population and infrastructure are gradually being concentrated in urban areas as a result of rapid urbanization, and concerns for disasters in urban areas are increasing. Vulnerability to and risks of disasters are particularly serious issues in declining areas with poor physical and social environment. The improvement of urban resilience is necessary to prevent disasters in these areas, and an index for evaluating the current resilience level in these areas is necessary. To determine the suitability of evaluation index application for disaster resilience in declining areas, this study generated a list of 24 evaluation indices by reviewing published studies on this topic and examined the applicability of these indices in a survey conducted with 30 experts. The independence of each index was assessed through policy network analysis. In addition, the relevance and applicability of the indices were determined, and their effectiveness was assessed using the fuzzy multiple-criteria decision making method. Lastly, the priorities of the evaluation indices were suggested by combining the two analysis methods mentioned above. The results of this study will likely contribute to the development of an evaluation index for effective evaluation of urban resilience in declining areas.
Keywords:
Declining Areas, Disaster, Resilience, Assessment Indicator, Expert Survey1. 서론
기후변화에 따른 재난재해의 발생 및 피해는 더욱 증가할 것으로 전망되는 가운데, UNISDR (UN International Strategy for Disaster Reduction; 유엔 재해경감전략기구)을 비롯해 많은 국제기구와 전문가들은 가뭄, 홍수, 열섬, 극한 폭우 및 자연재난 등 글로벌 환경변화에 대한 도시지역의 취약성은 지속적으로 증가할 것으로 전망한다(Elmqvist et al., 2019). 급격한 도시화로 도시 내 인구 집중, 하천 주변 토지이용의 고밀화 및 각종 시설물 증가 등 인프라가 집중됨에 따라 재난재해에 대하여 도시의 취약성 및 위험성은 더욱 심각해지고 있다(Han, 2015; Kim et. al., 2020, Shin et. al., 2021). 특히 도시지역에서 재난이 발생할 경우, 도시를 구성하는 다양한 요소가 상호 유기적으로 밀접하게 관련되어 있기 때문에 건축 · 시설물의 파괴는 물론 라이프라인 시설의 기능 정지 등으로 인해 도시기능의 총체적 마비와 막대한 경제적 손실을 초래할 수 있다(Oh, 2020). 특히, 물리적 · 사회적 환경이 열악한 쇠퇴지역은 노후 · 불량주택이 밀집해 있어 사회재난의 발생 우려가 높고, 저지대 · 비탈길 사면, 관거배관망 등 물리적 기반시설의 관리가 열악하고 재난재해 피해 및 대응에 대해 취약하여(Kim et. al., 2016), 사전예방 측면에서 재난재해에 대응하기 위한 체계적 관리가 더욱 요구된다(Kim et. al., 2020).
이러한 요구에 맞춰 재난발생 가능성을 전제로 피해의 최소화 또는 재난발생 후 일상으로 신속하게 돌아가는 리질리언스(Resilience; 회복력) 확보에 초점을 두는 방향으로 도시 방재정책의 패러다임이 변화하고 있다(Jeon and Byun, 2017). 라틴어 ‘resilio’에서 유래한 resilience(회복력)는 어원상 ‘회복 혹은 극복할 수 있는 능력’을 의미하며(Kim et. al., 2016; Kwon and Cha, 2016), 도시의 회복력은 사전예방 측면에서 재난재해의 대응 뿐만 아니라 재난재해로부터의 위협 감소와 영향 완화 등 도시의 지속가능성에 있어서 중요한 개념으로 발전하고 있다(Jung et. al., 2016; Lee et. al., 2019). 재해경감을 위하여 2015년 UNISDR이 채택한 센다이프레임워크(Sendai Framework for Disaster Risk Reduction, SFDRR)에서는 국가 및 지역차원에서 회복력 구축을 강조한 바 있으며(Choi et. al., 2017), 많은 도시에서 회복력 확보를 도시의 발전방향으로 설정하고(ICLEI, 2018) 커뮤니티 차원의 회복력을 강조하고 있다(IFRC, 2014).
하지만 커뮤니티 차원, 특히 재난재해에 취약한 쇠퇴지역이 얼마나 취약하고 위험성이 높은지에 대한 실증적인 근거의 부족으로 사전적 재난대응을 위한 정책 수립 및 시행에 어려움이 있으며(Kim et. al., 2020), 지역의 활성화 및 환경개선, 방재 등을 위한 사업을 추진하는데 있어 계획수립의 사전 · 사후평가 체계가 미흡하여 사업추진의 타당성 및 효과, 검증 등을 판단하는데 있어 한계가 있다. 특히 도시재생뉴딜 사업 등을 통해 쇠퇴지역 개선을 위한 정부정책이 활발하게 진행되고는 있으나, 대부분 커뮤니티 활성화, 사회복지기반 시설 및 경제활성화 등에 초점을 맞추고 있어, 기후변화 및 재난재해 발생에 따른 주민들의 건강과 안전한 삶을 확보하는데에는 한계가 있어 회복력 평가를 근거로 한 정책개선도 요구된다.
이에 본 연구는 쇠퇴지역 및 도시재생지역의 도시회복력을 평가할 수 있는 평가체계를 마련하기 위하여 도시회복력 평가와 관련된 선행연구 검토를 통해 평가지표 후보목록을 제시하고, 전문가 설문조사를 통해 우선순위를 도출하고자 하였다. 전문가 설문조사는 정책 네트워크 분석 및 Fuzzy 다기준의사결정법을 활용하여 분석하였으며, 분석결과의 종합을 통해 도출된 우선순위를 바탕으로 도시회복력 평가체계를 마련하는데 기초자료로 활용하고자 하였다.
2. 이론적 고찰
2.1. 회복력 평가지표 관련 고찰
일반적으로 회복력은 ‘회복력’ 이외에도 ‘탄력성’, ‘회복탄력성’, ‘리질리언스’, ‘탄력회복성’ 등 언급되고 있으며(Lee et. al., 2019), 적용되는 범위와 개념적 정의, 다루고 있는 시공간적 범위도 매우 다양하게 나뉘고 있다. 회복력은 기본적으로 ‘어려움으로부터 회복하는 능력(the ability to recover from adversity)을 의미하지만(Shanchez et al., 2018), 도시 및 방재분야로 넘어오면서 더욱 다양하고 복잡하게 변화하고 있다. 도시에서의 회복력은 ’단순히 원래 상태로 복귀만을 의미하는 것이 아니라, 위기 이전의 상태의 안정성(Stability)과 변화된 환경에 대한 적응력(Adaptability)을 확보하면서 어느 시점에서는 그로 인한 새로운 수준의 전환능력(Transformability)까지를 포괄하는 개념‘으로 규정되기도 하며(Walker et. al., 2004; Folke et. al., 2010; Kim et. al., 2017) 시설물 중심의 전통적인 구조적(structural) 대책 뿐만 아니라 도시환경의 다양한 물리적 영역, 위기관리체계, 사회경제적 역량 등 비구조적(non-structural) 대책이 병행되는 종합적 접근으로 정의되기도 한다(Shin and Kim, 2020).
도시공간에서의 회복력을 평가하기 위한 평가지표는 크게 도시의 구성요소(부문별)와 평가단위, 회복력의 특성 등으로 구분되고 있다. 국내 · 외 회복력 평가지표 관련 16개의 선행연구를 바탕으로 회복력 평가를 살펴보면, 회복력의 특성(가외성, 내구성, 신속성, 자원부존성)은 합의되고 있으나(Kim et. al., 2017) 회복력 평가의 구성요소와 평가단위는 상이하게 나타나고 있다(Table 1. 참조). 도시의 구성요소로서 자연환경 / 물리적 기반시설 및 건축물 / 경제 · 금융 / 인간 · 건강 / 지식 / 사회 / 문화 / 제도 / 거버넌스 · 정책 등 지표항목은 지표의 목적과 수준, 공간 단위 등에 따라 다양하게 나타나고 있다. 특히, 대부분의 평가지표는 지역과 도시, 커뮤니티 단위에서 평가하고 있으며, 특정 사이트(건축물, 개별 필지 또는 블록 단위)를 대상으로 회복력을 평가하는 연구는 아직까지 없는 것으로 나타났다.
2.2. 정책 네트워크 분석 관련 고찰
네트워크 분석(사회연결망 분석)은 선과 점으로 구성된 그래프를 도출하는 분석기법을 의미한다. 기본적으로 네트워크 분석은 Node(노드) 간의 상호작용을 시각적 · 수치적으로 확인 가능하다는 장점을 갖고 있다(Ludvig et al., 2007; Newell et al., 2018). 네트워크 분석은 노드 간의 관계에 규명하는 것에 관심이 있으며, 전반적인 체계를 파악하는 데 매우 유리하다(Choi et. al., 2011; Lee and Lim 2014). 따라서, 사회 · 인문 · 과학 등 분야를 막론하고 다양한 상황에 적용가능성이 높은 분석방법으로 활용되고 있다(Seo, 2019). 네트워크 분석은 하나의 지표 혹은 하나의 조직을 개별 개체로 분석하기 보다는 이들 사이에 상호작용, 관계로부터 나타나는 출현적 속성(Emergent property)을 강조한다는 점에서 변수 중심의 점근법과는 구별된다. 네트워크 시스템 혹은 관계망 안에 있는 노드의 관계를 강조함으로서 거시적 관점에서 시스템을 바라볼 수 있고 구성요소의 구조를 그려낼 수 있다(Kang, 2002; Ko, 2007).
네트워크의 특성을 파악하는 방법으로는 노드 수준분석, 하위집단 분석 등 다양한 분석이 이루어지고 있으나, 가장 많이 활용되는 기법은 중심성 분석이다(Park et. al., 2018). 정책연구는 복잡한 의사결정 과정을 이해하여야 하며, 정책 간의 관계도 복잡하게 얽혀있다는 어려움에 당면하게 된다(Kim, 2008). 분권화되고 다원화된 현대의 복잡한 정책현실을 기술하고, 영향력 있는 정책을 파악하거나 정책 지원에 우선순위를 파악할 수 있도록 하는 가장 좋은 설명 방법은 네트워크 분석으로 볼 수 있다(Park et. al., 2018). 그 중에서도 중심성은 보통 네트워크 구조에서 각 노드가 갖는 중요도라는 의미를 갖는데, 정책 연구에서 중심성은 거버넌스의 구체적이고 전체적인 구조를 파악하거나 정책 영향력을 분석하는 도구로 해석될 수 있어 기존의 개별 정책에 집중하는 연구와는 다른 새로운 시각을 제시할 수 있다. 또한, 쉽게 계량화 할 수 있는 유용한 수단이라는 강력한 장점을 갖고 있다(Kim, 2008; Seo, 2019).
다양한 연구 분야에 수많은 네트워크가 존재하는 만큼 분석에 활용되는 중심성 지표 역시 성격에 따라 다양하게 존재한다(Zafarani et. al., 2014). 연결 정도를 파악하는 활용되는 연결중심성(Degree Centrality), 노드를 잇는 최단 경로에 많이 포함된 노드일수록 중심에 있는 것으로 파악하는 근접중심성(Closness Centrality), 중요한 지표와 연결된 노드일수록 중요하다는 개념이 반영된 고유벡터중심성(Eigenvector Centrality) 등이 있다. 고유벡터 중심성과 이를 심화하여 외부로 향하는 Link(링크)의 가중치를 제한하는 Page rank와 같은 분석 방법이 가장 널리 활용되고 있다(Zafarani. et. al., 2014).
2.3. Fuzzy 다기준 의사결정법 관련 고찰
Fuzzy 이론을 기반으로 심화된 Fuzzy 다기준 의사결정법은 애매한 언어적 표현을 수치화하는 데 주로 활용되는 분석기법이다(Zedah, 1965). Fuzzy 이론은 실제 문제에서 발생하는 모호성과 불확실성 개념을 효과적으로 처리할 수 있는 이론으로 간주되어, 의학 · 공학 등 다양한 분야에서 응용되고 있으며(Lee and Lim, 2014), Fuzzy 다기준 의사결정법은 설문조사를 기반으로 의사결정 문제를 해결하는 방법으로 활용되고 있다. 평가 지표를 구축하거나 정책 우선순위를 결정하기 위해서는 사업에 관련한 이해당사자 혹은 관련 전문가가 세부 항목의 중요도를 기반하여 평가하고, 측정된 의견을 취합하는 과정이 필요하다. Fuzzy 다기준 의사결정법은 개별 지표 혹은 정책에 대한 각 개인의 선호를 수렴하고 대표성을 가지는 하나의 수치로 표현하는 여러 방법 중 설문조사에 의해 수집된 의견을 다루는 방법으로(Russell et. al., 2001), 기존의 방법론의 한계를 극복하기 위해 응답을 하나의 수로 표현하기보다 퍼지수를 부여하여 구간으로 표현한다(Dubois and Prade, 1978). 퍼지수의 형태는 연구자가 필요에 따라 다양하게 구성할 수 있지만, 간편하게 사용할 수 있는 장점이 있는 삼각퍼지수(Triangular Fuzzy Numbers)가 주로 활용되고 있다. 중요도를 구간으로 표현하고, 함수로 나타내는 과정을 거치며 신뢰수준이 반영된 중요도를 도출하게 되면, 결과값은 같은 방법으로 계산된 값들과 비교분석이 가능하다는 장점을 갖는다(Shon et. al., 2001).
Fuzzy 다기준 의사결정법의 적용절차는 크게 5단계로 구분할 수 있으며, 절차는 다음과 같다(Kwak et. al., 2009; Lee and Lim, 2014; Kwak and Lee, 2015).
첫째로 k개의 평가속성을 설정해야 한다. 본 연구에서는, 내부 연구진 회의 및 전문가 Focus Group 회의를 통해 24개의 평가지표 선정하였다. 두 번째 단계는 설문 응답자가 각 평가지표에 대해 평가할 수 있는 언어값을 정의하고, 동시에 응답자가 각 평가에 대해 어느 정도 확신하는지 묻는 언어값을 정의한다. 세 번째는 퍼지수(Fuzzy number)를 설정하는 단계이며, 퍼지수란 응답자가 내린 의사결정을 특정 수치가 아니라 구간으로 표현하는 수단을 의미한다. 구체적으로 퍼지수 M = (a,b,c)는 신뢰구간의 최소값 a, 중간값 b, 최대값 c으로 이루어져 있다. 만약, 응답자가 ‘보통 수준으로 중요하다’라는 선택을 하고, 이에 대해 퍼지수 M을 (0.5, 0.75, 1)이라고 부여한다면, 최소 0.5에서 1까지 구간으로 수치를 부여할 수 있다. 본 연구에서는 다음 식 (1)과 같은 형태로 적절성/평가가능성, 신뢰수준에 대한 퍼지수를 설정하였다.
(1) |
(W : 적절성/평가가능성 평가 S: 신뢰수준, 확신의 정도)
5점 척도의 경우, 응답자가 선택할 수 있는 언어값은 5개이며, Table 2와 같이 1/4 간격으로 떨어져 있는 세 원소로 이루어진 퍼지수를 구성할 수 있으며, 순차적으로 대응된다. 퍼지수를 부여하게 되면, 비로소 개별 지표에 대한 퍼지신뢰지수(Fi)를 도출할 수 있다(식 (2)).
(2) |
네 번째, 다섯 번째는 총합산값과 중요도(weights)를 계산하는 단계로, 식 (3)의 과정을 통해 도출할 수 있다. 여기서 통상적으로 α = 0.5로 가정하는데, 이는 의사결정자가 낙관적이지도 않고 비관적이지도 않은 중립적인 상태에서 의사결정을 했음을 의미한다. 마지막으로 총 합산값이 1이 되도록 정규화(Normalize)하여 최종적으로 각 지표의 중요도를 구할 수 있다.
(3) |
(4) |
3. 연구의 방법
3.1. 전문가 설문조사
본 연구에서는 앞서 이론적 고찰에서 검토했던 국내외 회복력 평가지표 관련 16개 선행연구를 대상으로, 지표의 속성에 따라 분류된 총 24개의 평가지표를 대상으로 전문가 설문조사를 통해 지표 적용의 타당성을 검토하고자 하였다. 설문조사는 학계 및 관련기관에 종사하는 국토도시 및 기후변화, 재난재해, 도시재생 분야 전문가 30인(학계 5인, 연구기관 24인, 민간 1인)을 대상으로 2020년 12월 7일~18일에 실시하였으며, 현재 도시재생사업이 주요하게 추진되고 있는 약 15만 ㎡ 내외의 커뮤니티 규모에서의 평가 적용을 고려하였다.
전문가 설문조사의 내용은 크게 2가지로 ①정책 네트워크 분석을 활용하여 지표 상호간의 독립성을 평가하기 위하여 매트릭스를 구성하고 0-3점 척도로 평가하고자 하였으며, ② Fuzzy 다기준 의사결정법을 활용하여 개별 지표의 적절성과 평가가능성, 그리고 신뢰수준을 1-5점 척도로 평가하고자 하였다.
3.2. 정책 네트워크 분석 방법
정책의 평가지표 구성에 있어 중요한 것은 서로 중복되지 아니하는 지표를 구성하는 것이다. 중복 평가되는 지표가 존재할 경우, 비용 효율적이지 못하며 독립적인 지표로 구성함으로써 더 넓게 평가될 수 있도록 하는 것이 중요하다. 이에 본 연구에서는 전문가 설문조사를 통해 유형별(물리적/사회적) 상호영향의 크기를 조사하였고, 네트워크 분석을 위한 최종 매트릭스를 2단계로 구분하여 구축하였다. 첫째는 24개 전체 정책지표에 대한 24*24 매트릭스이고, 둘째는 물리적/사회적 지표를 구분하여 각각의 12*12 매트릭스를 구축해 분석에 활용하고자 하였다. 전체를 기준으로 평가할 경우 물리적 평가지표와 사회적 평가지표가 서로 무관하기 때문에 전체적으로 높은 점수가 책정되는 문제가 발생할 수 있기 때문이다.
본 연구에서는 네트워크 분석 방법론을 활용하고자 지표간의 중복성이 높을수록 긴밀한 관계에 있는 것으로 판단하여 설문조사 시 높은 점수가 책정하도록 설문을 구성하였다(Table 3 참고). 예를 들어, 지표 A와 B가 상호관계가 긴밀하여 중복평가가 우려될 경우 3점, 상호관계가 거의 없어 독립 평가가 가능하다면 0점이 되도록 하였다. 설문을 응답한 총 30인의 응답 결과를 바탕으로 24*24 최종 매트릭스를 구축하였으며, 이러한 경우 한 링크의 최소값은 0, 최대값은 90까지 가질 수 있다.
네트워크 분석은 Netminer, Giphy, Python 3을 활용하여 수행하였으며, 분석에서 도출되는 노드는 각 평가지표 자체를, 링크는 각 평가지표의 상호의존성을 의미한다. 과거 이루어졌던 정책연구 대부분이 사례분석을 중심으로 질적 연구에 머물렀지만(Ko, 2007; Shin, 2017), 본 연구는 네트워크 분석방법을 통하여 평가지표간의 관계를 규명함으로서 거시적인 구조를 파악하고 계량화하고자 하였다.
3.3. Fuzzy 다기준 의사결정법의 분석 방법
네트워크 분석 결과 각 평가지표의 독립성을 확인했다면, Fuzzy 다기준 의사결정법을 통해서는 각 평가지표의 개별 효과성을 파악하고자 하였다. 평가지표의 효과성은 적절성과 적용가능성을 바탕으로 평가하고자 하였으며, 적절성이란, 해당 평가지표가 쇠퇴지역 및 도시재생지역의 회복력을 평가하기에 적절한 정도를, 적용가능성은 실제로 데이터 수집 · 평가가 가능한 정도를 의미한다.
Fuzzy 분석은 Python 3을 활용해 수행되었으며, 설문조사로부터 파악되는 적절성과 적용가능성 값은 개별 지표의 중요도를 동등하게 파악하고 각 지표에 대한 응답은 (매우 낮음(1) ~ 보통(3) ~ 매우 높음(5))으로 5점 척도로 진행하였다(Table 4 참고). 각 응답의 신뢰수준은 Fuzzy 다기준 의사결정법 적용을 위한 설문문항으로, 적절성과 적용가능성 응답에 대한 신뢰정도를 평가하며, 최종적으로 효과성은 적절성 Fuzzy값과 적용가능성 Fuzzy값을 곱한 값을 활용하여 우선순위를 도출하고자 하였다.
3.4. 평가지표 우선순위의 선정 방법
네트워크 분석은 노드 간의 관계를 규명하고, 전반적인 체계를 파악하기는 합리적이지만(Chung et. al., 2020), 각 노드의 중요도를 각각 파악하는 방법으로는 적절하지 않다는 한계가 있다(Lee, 2020). 또한, Fuzzy 다기준 의사결정법을 활용한다면, 개별 항목의 적절성을 평가할 수 있지만, 하나의 항목이 다른 항목과 어떤 상호관계에 놓여있는지, 또는 계층적 구조에 대하여 파악할 수는 없는 한계가 있다(Han and Lee, 2018).
본 연구의 목적은 도시회복력 평가지표의 적절성을 평가하고 그 우선순위를 도출하는 것으로, 어떤 하나의 지표가 도시회복력을 평가하는 데 가장 큰 영향을 미치는지 파악하는 것 뿐만 아니라 다른 평가지표와의 중복도가 낮은 지표를 선정하는 것도 중요하다. 도시회복력 전반을 평가하면서 동시에 중복된 평가지표를 선정하지 않음으로서 평가의 효율성을 높이는 것이 필요하기 때문이다.
따라서, 본 연구에서는 일차적으로 네트워크 분석을 활용해 중심성이 높은 지표를 탈락시키고자 하였다. 일반적으로 네트워크 분석에서 중심성이 높은 지표는 다른 지표와 연계성이 높은 지표로 평가되는데, 본 연구에서 설계한 설문지에서는 연계성을 중복도로 치환해 해석할 수 있다. 즉, 중심성 높은 지표는 다른 지표와 중복 평가될 우려가 높은 지표로 네트워크 분석에서 낮은 값을 부여하고 있다. 두 번째로, Fuzzy 다기준 의사결정법을 활용해 개별 지표의 적절성(평가지표로 적절한 정도)과 적용가능성(정량 · 정성평가가 가능한 정도)을 평가하여 효율성이 좋은 지표에 높은 값을 부여하도록 하였다. 최종적으로 (네트워크분석 결과값*효과성 결과값)을 통해 지표의 우선순위를 선정하였고, 최종으로 도출된 값이 높을수록 다른 평가지표와 중복성이 낮고, 평가의 효율성이 높은 지표라는 특징을 갖게 된다.
4. 연구결과
4.1. 선행연구 검토를 통한 평가지표의 도출
본 연구에서는 국내외 회복력 평가지표 관련 16개 선행연구를 대상으로, 평가지표에 사용되는 지표를 속성별로 분류하여 대분류로 물리적 부문과 사회적 부문으로 구분하였다. 이를 다시 과거 이력 또는 지역의 특성과 관련된 항목은 안정성으로, 재난재해 측면에서 준비 및 대응과 관련된 항목을 적응성으로, 재난발생 후의 회복을 위한 잠재력 능력과 관련된 항목을 전환능력으로 중분류로 분류하고, 마지막으로 개별지표별로 유사한 항목끼리 묶어 세분류로 묶어 총 24개의 회복력 평가체계의 후보목록을 도출하였다(Table 5 참조). 물리적 부문에서는 자연 · 환경적 특성 및 기반시설 등의 속성을 갖는 평가지표로서 안정성 측면에서는 재난발생 피해와 면적, 빈도 등의 과거 이력 중심으로, 적응성은 현재 기반시설의 능력 및 수용력을 중심으로, 전환능력은 재난대응을 위한 시설의 잠재력을 중심으로 평가지표가 도출되었다. 사회적 부문은 행정 · 사회 · 경제 · 거버넌스 등을 포함하여 안정성 측면에서는 인구, 경제, 교육 등 과거와 현재의 사회여건 현황을 중심으로, 적응성은 재난대응을 위한 준비상태를 중심으로, 전환능력은 인적 · 재원의 활용 및 주민참여 가능성을 중심으로 항목을 도출하였다.
4.2. 정책 네트워크 분석
24개 지표항목에 대한 30인의 전문가 설문조사 결과 평가항목의 상호 관계 당 47(최소값) ~ 82(최대값)를 가지는 것으로 나타났으며, 분석한 정책 네트워크 분석 결과는 다음 Fig. 1과 같다.
본 연구에서는 정책 네트워크 분석으로 도출할 수 있는 다양한 중심성(Centrality) 값 가운데 연결중심성(Degree Centrality) 값을 활용하였다. 평가지표의 특성상 30인의 응답은 다양하게 나타났으나, 모든 노드가 연결되는 것으로 나타나 연결의 유무가 중심성 값에 큰 영향을 미치는 방법에 중점을 두는 Pagerank 또는 Eeigenvector 중심성 대신 네트워크의 노드들이 얼마나 많은 연결(링크)을 가지고 있는지 확인하는 연결중심성 값을 활용하였다. 특히, 노드 간 중복성의 누적 응답 횟수(연결 빈도)를 가중치로 가지는 가중 연결중심성(Weighted Degree Centrality) 값을 활용하고자 하였다.
정책 네트워크 분석에서 확인하고자 하는 바는 지표 간 독립성을 파악하는 것이며, 중복 평가의 우려가 있는 지표는 독립적인 평가지표보다 낮은 우선순위로 책정하는 것이 비용 효율적이고 합리적이기 때문에 도출한 가중 연결중심성 값의 역수(inverse)를 최종 독립성을 판단하는 값으로 산정하였다.
전체 평가지표를 활용하여 구축한 24*24 매트릭스 분석 결과, 다음 Table 6과 같이 나타났으며 상위 6개 순으로 [사회적 유대관계(사회적 지표)], [교육수준(사회적 지표)], [정책수립 주민참여(사회적 지표)], [법제도적 기준 부합성(물리적 지표)], [행동유도 프로그램(사회적 지표)], [사회경제(사회적 지표)]가 나타났다. 24개 전체 평가지표를 하나의 매트릭스로 정책 네트워크를 분석하는 경우, 상위 10개의 평가지표 중 8개가 사회적 평가지표로 나타났으며, 이는 사회적 지표와 물리적 지표가 상호 관련성이 낮으며, 사회적 지표가 보다 독립적인 지표로 구성되어 있음에 기인한 것으로 판단된다.
따라서, 평가지표의 균등을 고려하여 물리적 평가지표와 사회적 평가지표 각 12개씩으로 나누어 매트릭스를 다시 구성하여 다음 Table 7과 같이 도출하였다. 물리적 평가지표의 경우 [재난발생 피해], [대응기반시설의 적정성], [자연생태적 탄력성], [재난발생 빈도], [건축물과 구조물의 안정성] 순으로, 사회적 평가지표는 [인구구성], [맞춤형 응급지원체계], [사전대응체계], [사회서비스 제공수준], [인적재원의 가용성] 순으로 나타났다.
4.3. Fuzzy 다기준 의사결정법을 활용한 분석
각 지표별로 각각의 신뢰수준을 고려한 적절성과 적용가능성의 fuzzy 값을 산출하였으며, 이들 값의 곱으로 지표별 효과성을 도출하였다(Table 8 참조). 네트워크 분석과는 달리, 개별 지표의 효과성을 파악하고자 하였기에 물리적/사회적 지표를 구분하여 분석하지는 않았다. 지표별 효과성이 높은 순으로는 [인구구성(사회적 지표)], [재난발생 피해(물리적 지표)], [자연생태적 탄력성(물리적 지표)], [재난발생 빈도(물리적 지표)], [대응기반시설의 적절성(물리적 지표)], [취약지역 면적(물리적 지표)] 등으로 나타났으며, 상위 10개의 지표 가운데 물리적 지표가 7개 도출되는 등 전체적으로 물리적 지표의 효과성이 높은 것으로 평가되었다.
4.4. 우선순위 선정
24개 평가지표의 우선순위 도출을 위하여 정책 네트워크 분석결과와 Fuzzy 분석결과를 종합하면 다음 Table 9와 같다. 우선순위 도출을 위하여 정책 네트워크 분석 결과인 가중 연결중심성 값의 역수와 Fuzzy 분석 결과인 효과성 값을 곱한 뒤, 종합적으로 도출된 값의 가독성을 높이기 위해 100,000을 곱한 값으로 구성되어 있다. 종합에 활용된 네트워크 분석 결과는 물리적/사회적 네트워크로 구분하여 파악한 값은 적용하였으며, 이들을 하나의 우선순위로 표현할 수 없기 때문에 최종적으로 우선순위 또한 물리적/사회적으로 구분하여 제시하였다.

Result of total analysis (policy network anlysis and Fuzzy Multiple-Criteria Decision-Making Method)
물리적 평가지표의 경우 안정성 측면의 지표가 전반적으로 우선순위가 높으며, [재난발생 피해], [대응기반시설의 적절성], [자연생태적 탄력성] 순으로 나타났다. 사회적 평가지표의 경우 적응성 측면의 지표가 전반적으로 우선순위가 높았으며, [인구구성], [맞춤형 응급지원체계], [사전대응체계] 순으로 우선순위가 나타났다.
본 연구에서 제시한 도시회복력 평가지표 24개의 우선순위 선정은 향후 도시재생지역을 포함한 쇠퇴지역에서의 계획 및 설계, 평가 과정에서 지역의 도시회복력 수준을 평가하기 위한 평가체계 개발에 있어 최종 평가항목 도출에 있어 유용하게 활용될 수 있을 것으로 판단된다. 지역의 도시회복력 수준을 평가하는 평가항목 도출 및 평가체계 개발을 통하여 지역의 현재 도시회복력 수준을 평가하고 계획수립 및 사업시행 이후 도시회복력 수준의 변화를 예측, 사후모니터링 등에 활용할 수 있으며, 재난재해에 사전대응할 수 있는 지역의 회복력 향상을 위한 방법론 마련에 기여할 수 있을 것이다.
5. 결론 및 고찰
기후변화에 따른 재난재해의 발생 및 피해는 증가하는 가운데 급격한 도시화로 인구 및 기반시설의 집중에 따라 도시지역에서의 재난재해 우려는 커지고 있으며, 특히, 물리적ㆍ사회적으로 열악한 쇠퇴지역의 경우 재난재해로부터의 취약성 및 위험성은 더욱 증가하고 있다. 이에 지역에서 발생할 수 있는 재난재해의 사전 예방적 측면에서 지역의 회복력 향상이 요구되며, 회복력 향상을 위한 다양한 기법 및 기술 개발과 함께 현재의 회복력 수준을 평가할 수 있는 평가체계 개발이 필요한 실정이다.
평가지표를 구축하는 데 있어, 평가지표의 상호 간 독립성과 개별 지표의 적절성 및 평가가능성은 매우 중요한 항목이다. 이들 중 하나에만 집중하여 지표를 구축하는 것은 바람직하지 않으며, 세 가지 항목이 상호 존중되면서 효율적인 균형점을 찾는 것이 필요하지만, 이를 계량화하여 정량적으로 평가하는 연구는 그 필요성에 비해 아직 부족한 실정이다. 이에, 본 연구에서는 정책 네트워크 분석을 활용해 지표 상호 간의 독립성을 평가하고, Fuzzy 다기준 의사결정법을 활용하여 개별 지표의 적절성과 평가가능성을 계량화 하였으며, 최종적으로 두 분석법의 종합을 통해 평가지표 선정을 위한 우선순위를 도출하는데 활용하고자 하였다.
연구결과를 요약하면, 첫째, 회복력 평가지표 관련 16개 선행연구 검토를 통하여 후보지표로 물리적/사회적 부문으로 구분된 총 24개의 회복력 평가지표 항목을 도출하였다. 물리적/사회적 부문별로 각각 안정성-적응성-전환능력의 항목으로 각 항목별 4개의 지표로 분류하여 정리하였다.
둘째, 지표 간 독립성을 파악하기 위하여 전문가 설문조사 결과를 바탕으로 정책 네트워크 분석을 실시하였으며, 네트워크의 노드들의 연결 유무를 기준으로 도출된 가중 연결중심성의 역수 값을 산출하였다. 24개 전체 평가지표로 구성된 24*24 매트릭스 분석 결과 사회적 지표의 독립성이 상대적으로 월등히 높았는데, 지표 분류의 특성에 기인한 것으로 판단되어 물리적/사회적 부문별 각 12개 평가지표로 구성된 12*12 매트릭스로 다시 분석하였다. 분석 결과, 물리적 지표에서는 [재난발생 피해], [대응기반시설의 적정성], [자연생태적 탄력성], [재난발생 빈도], [건축물과 구조물의 안정성] 순으로, 사회적 지표에서는 [인구구성], [맞춤형 응급지원체계], [사전대응체계], [사회서비스 제공수준], [인적재원의 가용성] 순으로 분석되었다.
셋째, 지표별 효과성 분석을 위하여 전문가 설문조사 결과를 바탕으로 fuzzy 다기준 의사결정법을 활용하였으며, 적절성과 적용가능성의 fuzzy 값의 곱을 통해 효과성을 도출하였다. 지표별 효과성이 높은 순으로는 [인구구성(사회적 지표)], [재난발생 피해(물리적 지표)], [자연생태적 탄력성(물리적 지표)], [재난발생 빈도(물리적 지표)], [대응기반시설의 적절성(물리적 지표)], [취약지역 면적(물리적 지표)] 등으로 나타났으며, 전체적으로 물리적 지표의 효과성이 높은 것으로 분석되었다.
마지막으로, 지표의 우선순위 도출을 위하여 정책 네트워크 분석을 통한 가중 연결중심성 값의 역수와 Fuzzy 분석 결과를 통해 도출한 효과성 값의 종합을 통해 분석하였다. 네트워크 분석 결과는 물리적/사회적 네트워크로 구분하여 파악하였기에 최종적으로 우선순위 또한 물리적/사회적 부문으로 구분하여 분석하였다. 물리적 지표의 경우 안정성 측면의 지표가 전반적으로 우선순위가 높게 나타났으며, [재난발생 피해], [대응기반시설의 적절성], [자연생태적 탄력성] 순으로 나타났다. 사회적 지표의 경우 적응성 측면의 지표가 전반적으로 우선순위가 높았으며, [인구구성], [맞춤형 응급지원체계], [사전대응체계] 순으로 우선순위가 높게 나타났다.
본 연구는 재난재해 회복력 평가지표를 개발하기 위하여 지표의 후보목록을 도출하였고, 각 지표별 상호 독립성과 효과성을 전문가 설문조사를 통해 계량화하여 우선순위를 제시함으로써 쇠퇴지역 및 도시재생지역에서의 도시회복력을 효과적으로 평가하기 위한 지표 개발에 기여할 수 있을 것으로 판단된다. 또한, 평가지표 개발에 있어 정량화된 방법론을 마련하는데 활용될 수 있기를 기대한다.
한편, 본 연구는 평가지표 간 상호 독립성 판단을 위하여 네트워크 분석을 통한 가중 연결중심성 결과를 활용하였으나, 네트워크의 연결만을 고려하였기 때문에 다양한 중심성 지수를 통한 해석이 추가적으로 필요할 것으로 판단된다. 또한, 효과성 분석에 있어 적절성과 적용가능성 종합을 통해 분석하였는데, 쇠퇴지역에서 실제로 데이터 수집 및 평가가 가능한가에 대해 판단하는 적용가능성의 경우 국가 또는 광역 단위에서는 평가가 가능하지만 커뮤니티 규모인 쇠퇴지역에서는 평가하기가 어려운 지표인 [재난발생 빈도(물리적 지표)] 등이 상대적으로 높게 평가되어 전체 효과성 및 우선순위까지 영향을 미치는 등 실제 평가지표로 적용하는데 있어 어려운 측면이 있으므로 이에 대한 추가 검토가 필요하다.
마지막으로, 본 연구에서 적용한 방법론은 설문조사 대상 선정에 있어 이해관계자를 포함할 수 있으며, 이를 통해 지역의 특성에 부합할 수 있는 실질적인 지표 체계의 마련과 지표에 적용할 수 있는 측정인자 도출을 위한 후속 연구가 필요하다. 특히, 측정인자에서 단순한 지역 또는 피해 현황(예를 들어, 지역인구수 또는 피해인구수 등)이 반영될 수 있으므로, 각 지표들을 대표하면서도 현실적으로 적용가능한 측정인자의 도출 및 방법론 개발 등이 필요할 것으로 판단된다.
Acknowledgments
본 논문은 국토교통부 ‘쇠퇴지역 재생역량 강화를 위한 기술개발사업’의 연구비지원(21TSRD-B151228-03)에 의해 한국환경연구원이 수행한 ‘쇠퇴지역의 도시공간 위험성 분석 및 도시회복력 향상을 위한 기술개발 연구[2021-009(R)]’의 일부 내용을 발췌하여 논문화한 것입니다.
References
- Argonne National Laboratory. 2020. Community Resilience Indicator Analysis: County-Level Analysis of Commonly Used Indicators from Peer-Reviewed Research. Homeland Security.
- Choi JH, Kim HS and Lim GG. 2011. Keyword Network Analysis for Technology Forecasting. Journal of Intelligence and Information Systems. 17(4). pp.227-240.
- Choi YJ, Hong SH, Lee SJ and Sohn HG. 2017. Analysis of Domestic Policy Trend and Role of Science and Technology After Sendai Framework for Disaster Risk Reduction. Journal of Civil and Environmental Engineering Research. 37(4). pp.765-773.
-
Chung SJ, Jung S, Jeon H S and Leem C S. 2020. Network Characteristics Analysis of the Eco-Industrial Park Program Using a Social Network Analysis (SNA) Method. Journal of The Korean Society of Mineral and Energy Resources Engineers. 57(2). pp.168-175.
[https://doi.org/10.32390/ksmer.2020.57.2.168]
-
Cutter S L, Ash K D and Emirich C T. 2014. The Geographies of Community Disaster Resilience. Global Environmental Change. 29. pp.65–77.
[https://doi.org/10.1016/j.gloenvcha.2014.08.005]
-
Cutter S L, Burton C G and Emrich C T. 2010. Disaster Resilience Indicators for Benchmarking Baseline Conditions. Journal of Homeland Security and Emergency Management. 7(1). Art 51.
[https://doi.org/10.2202/1547-7355.1732]
-
Dubois D and Prade H. 1978. Operations on fuzzy number, International Journal of System Science. 9(6). pp.613-626.
[https://doi.org/10.1080/00207727808941724]
-
Elmqvist T, Andresson E, Frantzeskaki N, McPhearson T, Olsson P, Gaffney O, Takeuchi K and Folke C. 2019. Sustainability and Resilience for Transformation in the Urban Century. Nature Sustainability. 2(4). pp.267-273.
[https://doi.org/10.1038/s41893-019-0250-1]
- EPA. 2017. Development of a Climate Resilience Screening Index (CSRI) : An Assessment of Resilience to Acute Meteorological Events and Selected Natural Hazards. USA.
-
Flanagan BE, Gregory E W, Hallisey E J, Heitgerd J L and Lewis B. 2011. A Social Vulnerability Index for Disaster Management. Journal of Homeland Security and Emergency Management. 8(1) Art 3.
[https://doi.org/10.2202/1547-7355.1792]
-
Folke C, Carpenter S R, Walker B, Scheffer M, Chapin T and Rockstrom J. 2010. Resilience thinking: integrating resilience, adaptability and transformability. Ecology and Society 15(4). art 20.
[https://doi.org/10.5751/ES-03610-150420]
- Foster K A. 2014. Resilience Measurements: Stocktaking of Ongoing Efforts in Developing Systems for Measuring Resilience. United Nations Development Programme.
- Han SW. 2015. Application of Disaster Prevention-type Urban Planning for Busan Urban Regeneration Area. Busan Development Forum. 156. pp.107-111.
-
Han JS, Lee DH. 2018. Study on the Methodology for the Policy Decision Making with Multi-Criteria. Ordo Economics Journal. 21(4). pp.131-148.
[https://doi.org/10.20436/OEJ.21.4.131]
- ICLEI. 2018. Resilient Cities Report 2018 : Tracking Local Progress on the Resilience Targets of SDG 11.
- International Federation of Red Cross and Red Crescent Societies (IFRC). 2014. IFRC Framework for Community Resilience.
- Jeon EY and Byun BS. 2017. A Study on the Development and Application of Community Resilience EvaluationIndicators for Responding to Climate Change. The Geographical Journal of Korea. 51(1). pp.47-58.
- Jung EJ, Jeong BH and Na JM. 2016. A Study on the Sustainability and Resilience of City. Journal of The Korean Regional Development Association. 28(4). pp.87-108.
- Kang ES. 2002. Policy-Network Analysis on Policy Change: Focusing on Green-Belt Policy. Korean Society and Public Administration. 13(1). pp.103-125.
- Kang SJ, Cho SH and Hong SY. 2013. A Policy Implication for Community Resilience from Natural Disasters. Gyeongi Research Institute.
- Kim DH, Song SK, Kang SJ, Kwon TJ, Kim JO, Nam KC, Yoon DK, Lee DK, Jung JC, Cho SC and Hong SH. 2016. Urban Climate Resilience: Operationalization and Evaluation (Ⅱ). Korea Environment Institute.
- Kim JK, Lim JH and Lee SH. 2016. A Research on Urban Resilience for Urban Regeneration. Land and Housing Institute.
- Kim JK, Lim JH and Lee SH. 2017. A Research on Resilient City Evaluation Criteria and Urban Regeneration Application. Land and Housing Institute.
-
Kim JY, Park JH, Cho BH and Lee SS. 2020. A Comparative Analysis of Disaster Vulnerability Factors between Declining Areas and Urban Areas. Journal of Digital Contents Society. 21(11). pp.2021-2032.
[https://doi.org/10.9728/dcs.2020.21.11.2021]
- Kim OI. 2008. A Study on the Change of Policy Network Structure in Project of the National Education Information System (NEIS): Utilization of Social Network Analysis. Korean Journal of Public Administration. 46(2). pp.255-279.
- Ko KK. 2007. The Review of Studies on Policy Network and the Application of Social Network Analysis. Korean Journal of Public Administration. 45(1). pp.137-164.
- Kwak SY, Yoo SH and Hwang SW. 2009. The Public Assessment of the Urban Regeneration R&D Program: Using Fuzzy Decision Making Analysis. Journal of the Korean Regional Science Association. 25(4). pp.5-21.
-
Kwak SJ and Lee JS. 2015. Deduction of Attributes' Weight for Companies' Job Creation by Applying Fuzzy Decision Making Analysis. Journal of Korea Academia-Industrial cooperation Society. 16(11). pp.7971-7977.
[https://doi.org/10.5762/KAIS.2015.16.11.7971]
-
Kwon YJ and Cha MH. 2016. A Study on the Research Trend of Resilience using Keyword Network Analysis. Korean Journal of Counseling. 17(6). pp.105-121.
[https://doi.org/10.15703/kjc.17.6.201612.105]
-
Lee GS, Jin DY, Song SK and Choi HS. 2019. Text Analysis on the Research Trend of ‘Resilience’ in Korea : Focus on Climate Change and Urban Disaster. Journal of Climate Change Research. 10(4). pp.401-414.
[https://doi.org/10.15531/KSCCR.2019.10.4.401]
- Lee HJ. 2020. Analysis on Research Trends of Sexual Harassment using Keyword Network Analysis. The Women’s Studies. 106(3). pp.209-243.
- Lee JS and Lim JE. 2014. Fuzzy Decision Making Analysis for Assessment of Carbon Dioxide Capture and Storage. Innovation studies. 9(2). pp.99-116.
-
Lee SS. 2014. A Content Analysis of Journal Articles Using the Language Network Analysis Methods. Journal of the Korean society for information management. 31(4). pp.49-68.
[https://doi.org/10.3743/KOSIM.2014.31.4.049]
- Ludwig F, Scheltinga C T, Verhagen J, Kruijt B, Ierland E, Dellink R, Bruin Karianne, Bruin Kelly and Kabat P. 2007. Climate change impacts on developing countries-EU accountability. Europopean Commission.
- Morley P, Parsons M and Johal S. 2017. The Australian Natural Disaster Resilience Index: A System for Assessing the Resilience of Australian Communities to Natural Hazards. Bushfire & Natural Hazards CRC.
-
Newell K A, Adams A B and Turka L A. 2018. Biomarkers of operational tolerance following kidney transplantation–The immune tolerance network studies of spontaneously tolerant kidney transplant reciean Parliament, Brussels.
[https://doi.org/10.1016/j.humimm.2018.02.007]
- Miola A, Paccagnan V, Padadimitriou E and Mandrici A. 2015. Climate resilient development index:theoretical framework, selection criteria andfit-for-purpose indicators. JRC Science and Policy Reports. Eurpients. Human immunology. 79(5). pp.380-387.
- Oh SN. 2020. A Study on Quantitative Risk and Assessment of Urban Natural Disasters by Climate Change. Konetic Report. Korea Environmental Industry & Technology Institute.
-
Park JW, Seo JH, Lee DH, Na KI, Cho SY and Bae MJ. 2018. Evaluation of Results in Pesticide Residues on Incongruity Commercial Agricultural Commodities using Network Analysis Method. Journal of Food Hygiene and Safety. 33(1). pp.23-30.
[https://doi.org/10.13103/JFHS.2018.33.1.23]
- Peacock W G, Brody S, Seitz B, Merrell B and Harris B. 2010. Advancing Resilience of Coastal Localities: Developing, Implementing, and Sustaining the Use of Coastal Resilience Indicators: A Final Report. Hazard Reduction and Recovery Center.
-
Russell C, Dale V, Lee JS, Jensen M H, Kane M and Gregory R. 2001. Experimenting with multi-attribute utility survey methods in a multi-dimensional valuation problem. Ecological Economics. 36(1). pp.87–108.
[https://doi.org/10.1016/S0921-8009(00)00207-X]
-
Seo KC. 2019. An Analysis on the Centrality of Network Structure in Land Markets: Focused on Land Sales Markets in the Seoul Region. APPRAISAL STUDIES. 18(1). pp.203-232.
[https://doi.org/10.23843/as.18.1.9]
-
Shanchez A X, Heijden J and Osmond P. 2018. The city politics of an urban age: urban resilience conceptualisations and policies. Palgrave Communications 25(4).
[https://doi.org/10.1057/s41599-018-0074-z]
- Shaw R, Takeuchi Y, Joerin J, Fernandez G, Tjandradewi B I, Chosadillia, Wataya E, McDonald B, Fukui R, Sharma A, Tsunozaki E and Matsuoka Y. 2010. Climate and Disaster Resilience Initiative: Capacity-building Program. Kyoto University, Citynet and UNISDR Hyogo.
-
Sherrieb K, Norris F H and Galea S. 2010. Measuring Capacities for Community Resilience. Social Indicators Research. 99. pp.227–247.
[https://doi.org/10.1007/s11205-010-9576-9]
- Shin SJ. 2017. Policy Network and Public Conflicts: Focusing on the Policy-making Process on the Seoul Metropolitan Landfill Site. The Korea Association for Policy Studies. 26(3). pp.169-203.
- Shin SY and Kim SG. 2020. Coping with Emerging High-impact Urban Disasters in Seoul. Policy Report 301. Seoul Institute.
-
Shin YH, Lee SM, Chang KW and Yang DM. 2021. Development of Comprehensive Diagnosis Model for Urban Space in Deteriorated Areas : Focusing on Disaster Risk and Resiliency. Journal of Korea Planning Association. 56(1). pp.169-176.
[https://doi.org/10.17208/jkpa.2021.02.56.1.169]
-
Shon KY, Yang JW and Kang CS. 2001. Assimilation of public opinions in nuclear decision-making using risk perception. Annals of Nuclear Energy. 28(6). pp.553-563.
[https://doi.org/10.1016/S0306-4549(00)00076-1]
- The Rockfeller Foundation and Arup. 2014. City Resilience Framework. Arup.
- U.S. IOTWS. 2007. How Resilient is Your Coastal Community? - A Guide for Evaluating Coastal Community Resilienceto Tsunamis and Other Hazards, U.S. Indian Ocean Tsunami Warning System Program.
-
Walker B, Honning C S, Carpenter S R, and Kinzig A. 2004. Resilience, adaptability and transformability insocial-ecological systems. Ecology and society. 9(2). art 5.
[https://doi.org/10.5751/ES-00650-090205]
- World Economic Forum(WEF). 2021, The Global Risks Report 2021.
- Yoon DK and Kang JE. 2013. A Measurement of Community Disaster Resilience in Korea. Proceeding of International Symposium on City Planning 2013.
-
Zadeh L A. 1965. Fuzzy Sets. Information and Control. 8(3). pp.338-353.
[https://doi.org/10.1016/S0019-9958(65)90241-X]
- Zafarani R, Abbasi M A and Liu H. 2014. Social media mining: an introduction. Cambridge University Press.