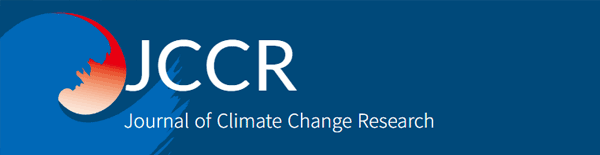
기온과 토양 및 식물 표면 온도 변화


Abstract
This study focuses on changes in air, soil surface and plant surface temperature. The principle results are as follows: (1) The ratio of change in soil and plant surface temperature to air temperature was 0.80-1.17 and 0.88-1.19oC/oC, respectively. (2) The difference in temperature between air temperature and soil and plant surface temperature was a linearly decreasing function of soil surface temperature and plant surface temperature, respectively. (3) A model for estimating air, soil surface and plant surface temperature was developed as a trigonometric function form (TFM) with Root mean square error (RMSE) of 1.75, 1.90-2.41 and 1.85-2.21oC, and NSE of 0.51, 0.37-0.58 and 0.39-0.61, respectively. (4) The longer change frequency the sooner inflection point for air, soil surface and plant surface temperature.
Keywords:
Soil Surface Temperature, Plant Surface Temperature, TFM (Trigonometric Function Model), RMSE (Root Mean Square Error), NSE (Nash-Sutcliffe Coefficient)1. 서론
우리나라 기상청의 『한국기후변화 평가 보고서 2020』에 의하면 우리나라 평균 지표 기온은 1981 ∼ 2010년 기간 동안 0.41 oC/10년, 2001∼2010년 기간에는 0.5 oC/10년의 변화율을 보여 전 지구 평균보다 큰 상승추세가 나타났다. 지표 기온 상승 경향은 한반도 전역에서 공통 적으로 나타나지만, 수도권 및 내륙지역에서 더 뚜렷하다. 이는 우리나라의 지표 기온 상승에 도시화의 효과가 포함되어 있기 때문으로 판단된다고 하였다(KMA, 2020). 기후변화와 관련된 현상 분석을 위한 모형(Model)의 활용에서도 온도 요인은 필수 입력요인이다(Watson and Challinor, 2013; Ye et al., 2013; Kim et al., 2014). 기온뿐 아니라 기온에 영향받는 토양표면 온도(SST : Soil surface temperature) 역시 기후변화의 중요한 지표(indicator) 중 하나이다. 특히, SST는 기후변화와 토양의 특성에 따라 민감하게 변하며(Li and Becker, 1993; Qin et al., 2001). 식물생육과 토양수분에 큰 영향을 미친다(Owen et al., 1998; Zhang et al., 2009; Li et al., 2018; Urqueta et al., 2018; Chi et al., 2020). 따라서 SST는 토양수분과 식물의 농업적 한발을 예측하는 데도 이용되고 있다(Weng et al., 2004; Han et al., 2010; Karnieli et al., 2010; Son et al., 2012; Muro et al., 2018; Ferreira and Duarte, 2019). SST는 기후변화 특히 일사량과 대기조건 및 토양의 물리·화학적 특성에 크게 영향을 받는다. 그러나 본 연구처럼 동일 장소에서 지표 기온과 토양 및 식물 표면 온도를 monitoring 하는 경우 토양특성이 변하지 않는 상태이므로 일사량과 대기 조건뿐 아니라 토양 피복 상태와 식생 상태가 SST 변화의 중요한 요인이 된다(Zhang et al., 2009; Bertoldi et al., 2010; Karnieli et al., 2010; Eleftheriou et al., 2018). 토양 및 식물체 표면 온도는 기상뿐 아니라 토양수분 함량이나 식물체 생리작용 등에 따라 종합적으로 변화하지만, 기온이 변하면 토양 및 식물 표면 온도도 이에 영향을 받아 변하게 되므로 지표 기온 1oC 변화에 대하여 다른 온도들이 얼마만큼 변하는지 구명할 필요가 있다. 그러나 이들을 측정한 raw data 또는 단순 plotting에 의하여 개략적인 변화성은 파악이 되지만, 이들만 가지고는 온도의 변화성 즉 변화 진폭이나 변화 주기성 및 변화 변곡점 시기 등을 계량적으로 산정하기 어려우므로, modeling이라는 과정을 통하여 실측치에 근거한 추정모형을 설정하고 설정된 모형의 계수를 분석하여 그 변동성을 계량적으로 파악할 수 있으므로 온도 변화와 관련한 modeling 연구가 많이 수행되고 있다(Fu et al., 2011; Derradji and Aiche, 2014; Sofyan et al., 2020; Islam et al., 2021). 또한, 어떤 물체의 온도 등을 측정할 경우 그 측정치 및 모형에 의한 추정치는 오차를 포함하고 있다. 이 오차가 참값과 어느 정도의 변이를 나타내는가를 판단하는 것이 정확도(Accuracy) 분석이나 자연현상의 측정에는 참값을 알 수 없으므로 정확도 분석이 불가하여, 일반적으로 그 측정치에 대한 재현성 즉 정밀도(Precision)를 분석하게 된다. 이와 같은 정밀도 분석은 오차 분석 이론(Goodman, 1960; Kendal and Stuart, 1963; Mandel, 1964; Shein et al., 2013; Irsyard et al., 2019; Eom et al., 2020)에 바탕을 두고 이루어져 오고 있다. 또한, 그 추정모형의 적절성(Appropriateness)은 측정치(Observed value)와 모형에 의한 추정치(Estimated value)의 차이에 근거하여 결정계수(R2: Coefficient of determination) 또는 상관계수(r: Correlation coefficient), 평균 편차(MD: Mean deviation), Root mean square error(RMSE), Nash-Sutcliffe coefficient(NSE) 등 여러 가지 지표에 근거하여 그 모형이 『적절하다(Appropriate)』라고 할 수 있는지 판단하게 된다(Nash and Sutcliffe, 1970; Moriasi et al., 2007; Naseri et al., 2021). 『한국기후변화 평가 보고서 2020』은 RMSE를 기준으로 하여 모델의 우세를 비교 판단하였다. 본 연구는 수원에서 기온과 식물 및 그 식물이 생육하고 있는 토양의 표면 온도를 측정하고 측정치에 근거하여 지표 기온 변화에 따른 토양 및 식물 표면 온도의 변화양상을 분석하고, 이들에 대한 추정모형을 trigonometric function 형태인 four-parameter sine-wave model (TFM)로 설정하여, R2(곡선 회귀) 또는 r(직선 회귀), MD, RMSE, NSE 등을 산출하여 모형의 적절성을 판단한 후, 설정된 모형들의 계수를 분석하여 지표 기온과 토양 및 식물 표면 온도의 변동성을 구명코자 실시하였다.
2. 재료 및 방법
본 연구의 온도 조사는 경기도 수원시 우만동 농가 포장에서 2015년 5월 21일부터 7월 24일 기간 동안 기상 조건이 불균일한 일자는 제외하고 42일간 매일 오전 11:00에 측정하였다. 지표 기온은 지상 30 cm 높이의 대기 온도를, 소나무, 장미, 회양목, 고추 등 4종의 식물체 표면 및 온도는 군락 내의 직사광을 받지 않는 방향에서 지상 30 cm 높이에서 온도계의 초점이 가장 잘 맞는 15 cm 거리에서 소나무는 줄기 표면 부분에, 나머지 식물들은 잎 표면에 적외선을 방사시켜 측정하였다. 토양표면 온도측정은 소나무와 장미의 경우는 토양표면이 완전히 피복 된 상태가 아니며 나무표면 온도를 측정한 지점의 뿌리 부근에 나지로 노출된 부분의 직사광선을 받지 않고 나무 그늘로 인한 음지 상태의 토양표면, 회양목과 고추의 경우는 군락이 형성되어 토양표면은 완전히 음지 상태이며 나무표면 온도를 측정한 지점의 뿌리 부근의 토양표면에 대하여 15 cm 거리를 두고 토양표면과 수직 방향으로 적외선을 방사시켜 측정하였다. 식물 표면 온도와 토양 표면 온도는 각각 지정된 측정 지점에서 표면 온도를 각각 5회씩 반복 측정하여 그 평균치를 온도로 삼았다(Fig. 1). 포장의 토양은 토양분류 상 본량통이며, 표토는 세사양토(Fine Sandy Loam)이고 심토는 양토(Loam)이며 토양 배수가 양호한 편이다. 본 연구에서 대상 식물을 소나무, 장미, 회양목, 고추를 선택한 이유와 토양 및 식물 표면 온도를 조사하게 된 이유는 기온이 기후변화에서 중요하지만 토양 및 식물 잎 표면 온도가 직접적으로 기후변화의 영향을 평가하는데 더 중요하다고 생각하며, 식물 잎 표면 온도는 일조시간, 일사량, 태양광에 대한 Albedo, 강수량 등 환경 조건뿐 아니라 식물 구조에 따른 태양광의 수광 각도, 엽면적 지수, 증산에 따른 잠열 손실, 생육 시기 등 식물 내적 조건의 수많은 요인에 영향을 받으며, 토양표면 온도 역시 환경 조건뿐 아니라 식물상에 의한 토양표면 피복도, 토양수분 함량, 토양특성에 따른 토양수분 이동양상, 토양입자 조성에 따른 열전도도, 토양표면을 통한 토양수분 증발과 식물 뿌리를 통한 증산에 의한 토양수분 소모과정(증발산)에서의 잠열 손실, 토양 구조에 따른 수리전도도, 식물 뿌리 생장에 따른 토양 물리성의 변화 등 토양학 적의 수많은 요인에 영향을 받는다(Rattan and Manoj, 2004). 따라서 현재까지 식물 및 토양표면 온도에 대한 명확한 온도 반응 법칙이 구명되어 있지 못한 실정이다. 이와 같은 이유로 본 논문에서 온도의 변화양상에 대하여 식물 생리적으로 또는 토양학적으로 어떤 과정을 통해서 그와 같은 결과가 나왔다고 명확히 밝히는 것은 불가능하지만 자연현상을 조사하여 그 결과를 보고하는 논문으로 작성하였다. 본 연구에서는 같은 포장에서 생육하고 있으며, 식물의 구조, 엽면적 지수, 증발산량, 식물생육 상에 따른 토양피복도 등이 서로 다른 세 가지 나무들과 나무와 더 큰 차이가 있는 재배작물인 고추는 기온 변화에 대한 표면 온도의 변화율, 변화 주기 등 온도 반응은 서로 다를 것이라는 가설에 근거하여 상기 네 가지 식물에 대한 온도를 조사하게 되었다.
지표 기온과 식물 및 토양의 표면 온도에 대한 추정모형을 trigonometric function 형태인 four-parameter sine-wave model (TFM)로 선정(Paul et al., 2004; Derradji and Aiche, 2014; Eom et al., 2014; Lee et al., 2015; Xu et al., 2020; Islam et al., 2021)하고 최소제곱법에 따라 오차가 최소인 계수를 산정하였다(1).
(1) |
단, X : 년 중 해당 순의 순서 [1(1월 상순) ∼ 36(12월 하순)]
yo, a, b, c : 계수
여기서 yo는 모형 전체의 값에 영향을 미치는 절편으로써 보정 계수이며 a는 변화 진폭, b는 변화 주기, c는 변곡점의 시기와 관련된 계수이다.
모형 검증을 위한 지표로 삼은 MD, RMSE 및 NSE는 식 (2), 식 (3) 및 식 (4)과 같이 산정하였다(Moriasi et al., 2007; Frot et al., 2008; Wang and Li, 2010; Moriasi et al., 2015; Nruthya and Srinivas, 2015; Fu et al., 2018; Chen et al., 2019; Fuamba et al., 2019; Naseri et al., 2021).
(2) |
단, Pi : 모형에 의한 추정치(Estimated value)
Oi : 실측치(Observed value)
n : sample 수
(3) |
단, Pi : 모형에 의한 추정치(Estimated value)
Oi : 실측치(Observed value)
n : sample 수
(4) |
단, Pi : 모형에 의한 추정치(Estimated value)
Oi : 실측치(Observed value)
Om : 실측치의 평균
n : sample 수
3. 결과 및 고찰
3.1. 기온과 토양 및 식물 표면 온도의 변화양상
온도측정 기간의 Julian date 별 온도 변화양상은 1 ∼ 2일 사이에서도 온도 차이가 크게 나는 양상을 보였으며(Fig. 2), 평균 기온(Ta)과 토양표면 온도(Ts) 및 식물 표면 온도(Tp)는 각각 23.99, 22.17 ∼ 22.85 및 22.16∼23.31oC 인 것으로 볼 때, Ta > Tp > Ts 순으로 높았다. 특히 고추의 Ts 및 Tp는 나무들의 Ts 및 Tp보다 낮았다(Table 1, Table 2). 모든 측정치의 5회 반복 간 표준편차는 1.0oC 이하로서 매우 작았다. 이와 같은 결과는, 잠재증발산량(PET: Potential evapo-transpiration)에 대한 식물 최대증발산량의 비율인 식물계수(Kp: Plant coefficient)가 고추는 0.84(Eom et al., 2010), 나무들은 0.4-0.6(Doorenbos and Pruitt, 1977)이기 때문에 식물체의 증산에 의한 잠열 손실과 토양수분의 증발산에 의한 잠열 손실이 고추가 나무들보다 더 많았기 때문이라고 사료 된다.
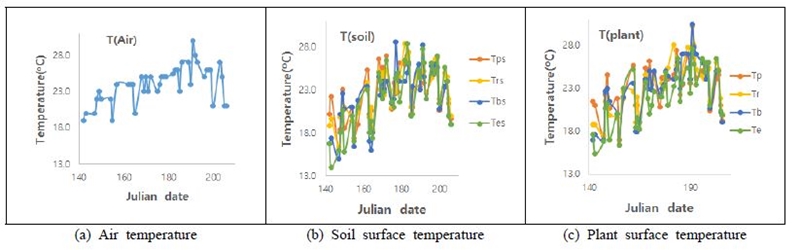
Changes in air [a : T(Air)], soil surface [b : T(soil)] and plant surface [C : T(plant)] temperature
기온과 토양표면 온도 및 식물 표면 온도와의 상관관계는 네 식물 모두 통계적으로 높은 유의성을 보였다(Fig. 3, Fig. 4). 이와 같은 결과는 기온과 토양온도는 깊은 상관관계가 있다는 연구 결과(Romanovsky and Osterkamp, 1997; Vancutsem et al., 2010; Zhu et al., 2013; Kloog et al., 2014; Huang et al., 2015; Yoo et al., 2018; Bayatvarkeshi et al., 2021)와 유사한 결과를 보였다. 두 가지 온도의 상관관계에서 기온에 대한 토양표면 온도 변화의 기울기가 뜻하는 기온 1oC 증가에 따른 토양표면 온도 증가율은 소나무 토양, 장미 토양, 회양목 토양 및 고추 토양의 표면 온도의 경우 각각 0.80, 0.94, 1.05, 1.17oC/oC 이었던 것으로 볼 때 소나무 토양과 장미 토양의 표면 온도 변화율은 기온보다 낮았으나 회양목 토양과 고추 토양의 표면 온도 변화율은 기온보다 높았다(Fig. 3).
기온 1oC 증가에 따른 식물 표면 온도 증가율은 소나무, 장미, 회양목, 고추의 표면 온도의 경우 각각 0.88, 1.07, 1.19, 1.06oC/oC 이었던 것으로 볼 때 소나무의 표면 온도 변화율은 기온보다 낮았으나 장미, 회양목, 고추의 표면 온도 변화율은 기온보다 높았다(Fig. 4).
Fig. 3과 Fig. 4의 결과에 근거하여 Ts의 변화에 대한 Tp의 변화율(dTp/dTs)을 식 (5)에 의하여 산정한 결과, 소나무, 장미, 회양목 및 고추의 경우 각각 1.075, 1.138, 1.133 및 0.846oC/oC로서 나무들은 1.0보다 컸으나 고추는 1.0보다 작았다.
(5) |
단, dTp/dTa: 기온 1 oC 증가에 따른 식물 잎 표면 온도 증가율
dTs/dTa: 기온 1 oC 증가에 따른 토양표면 온도 증가율
이와 같은 결과는 기온 변화에 의한 식물 증산에 의한 잠열 손실(dp)과 토양수분 증발산에 의한 잠열 손실(ds)에 대하여, 나무들 및 고추의 Kp와, 고추처럼 Kp가 높은 작물은 작물에 의한 증산보다 토양수분의 증발산량이 더 많다는 연구결과(Eom et al., 1990)에 근거하여 고찰하여 볼 때, 나무들은 dp가, 고추는 ds가 상대적으로 더 많았던 것에 기인 되었다고 사료 된다.
3.2. 기온과 토양표면 온도와의 차이 및 식물 표면 온도와의 차이 변화양상
토양표면 온도는 기온에 절대적인 영향을 받지만, 기온과 토양표면 온도와의 차이(Ta-Ts)는 네 가지 경우 모두 기온과는 통계적으로 유의성 있는 상관관계를 보여주지 않았으나(Fig. 5), 네 가지 경우 모두 토양표면 온도와는 직선적인 부의 상관관계를 보였다(Fig. 6). 즉, (Ta-Ts)는 기온보다 토양표면 온도와 더 강한 상관관계를 보였다.
기온과 식물 표면 온도와의 차이(Ta-Tp)는 네 가지 경우 모두 기온과는 통계적으로 유의성 있는 상관관계를 보여주지 않았으나(Fig. 7), 네 가지 경우 모두 식물 표면 온도와는 직선적인 부의 상관관계를 보였다(Fig. 8). 즉, (Ta-Tp)는 기온보다 식물 표면 온도와 더 강한 상관관계를 보였다.
3.3. 기온, 토양 및 식물 표면 온도의 추정모형 설정
기온에 대한 추정모형은 식 (6)과 같이 설정되었으며, 모형에 대한 결정계수(R2 : Fig. 9: a) 및 실측치와 추정치 관계의 결정계수(R2 : Fig. 9: b) 모두 통계적으로 유의성이 인정되었다(Fig. 9).
(6) |
토양표면 온도에 대한 추정모형은 식 (7a) ∼ 식 (7d)과 같이 설정되었으며, 네 가지 경우 모두 모형의 결정계수(R2)가 통계적으로 유의성이 인정되었다(Fig. 10).
(7a) |
(7b) |
(7c) |
(7d) |
식물 표면 온도에 대한 추정모형은 각각 식 (8a) ∼ 식 (8d)과 같이 설정되었으며, 네 가지 경우 모두 모형의 결정계수(R2)가 통계적으로 유의성이 인정되었다(Fig. 11).
(8a) |
(8b) |
(8c) |
(8d) |
3.4. 기온, 토양 및 식물 표면 온도의 추정모형 검증 및 계수 분석
어떤 모형에 대한 검증을 위해서는 실측치와 모형에 의한 추정치에 대한 정밀도를 분석하여야 하며, 이 경우 결정계수(R2: Coefficient of determination)에만 근거하여 판단하면 위험하고, 비록 R2값이 통계적 유의성이 있다 하더라도 RMSE 및 NSE 등의 지표와 함께 판단하여야 하며, 이때 RMSE는 낮은 편이면서 NSE가 양수이며 높을수록 모형에 의한 추정치 또는 그 모형이 『적절하다』라고 판단하는 것이 더 합리적인 방법이라 하였다(Moriasi et al., 2007; Moriasi et al., 2015; Naseri et al., 2021; Zhang et al., 2021). 따라서 본 연구에서도 설정된 추정모형에 대하여 『적절하다』라는 판단의 기준으로 R2과 NSE 및 RMSE에 근거하여 판단코자 한다. 기온에 대하여 실측치와 추정치의 관계는 Fig. 9(b)에 나타내었으며, 토양표면 온도와 식물 표면 온도에 대한 실측치와 추정치 관계를 절편 없이 1:1 line의 1차 회귀직선에 대한 상관계수(r)는 네 가지 경우 모두 통계적으로 유의성이 인정되었다(Fig. 12, Fig. 13). 또한, 기온과 토양표면 온도 및 식물 표면 온도에 대하여 식 (2)에 의해 산출된 MD는 기온이 1.43oC 이었으며 토양표면 온도가 1.57 ∼ 2.06oC 식물 표면 온도는 1.54 ∼ 1.93oC 범위로서 모두 낮은 편이었고, 식 (3)에 의해 산출된 RMSE는 기온이 1.75oC 이었으며 토양표면 온도가 1.90 ∼ 2.41oC 범위 식물 표면 온도는 1.85 ∼ 2.21oC 범위로서 모두 낮은 편이었으며, 식 (4)에 의해 산출된 NSE는 기온이 0.51이었으며 토양표면 온도가 0.37 ∼ 0.58 범위 식물 표면 온도는 0.39 ∼ 0.61 범위로서 모두 높은 편이었다(Table 3, Table 4). 이와 같은 결과는, MODIS 모형으로 추정된 토양표면 온도의 RMSE가 3.453 oC이었다는 Fu et al.(2018)의 결과와 비교하여도 본 연구의 모형이 더 우수하다고 판단되며, RMSE는 낮을수록 NSE는 양수이면서 높을수록 모형의 『적절성』이 높아진다는 모델 검증 이론(Moriasi et al., 2007; Moriasi et al., 2015; Naseri et al., 2021; Zhang et al., 2021)에 근거하여 판단할 때 본 연구의 모형(TFM)들은 모두 『적절하다』라고 판단되었다.
온도 추정모형의 계수를 분석해 보면, 온도의 변화 진폭과 관련된 계수 a를 볼 때 토양표면 온도(2.60 ∼ 4.63) ≧ 식물 표면 온도(2.33 ∼ 3.42) > 기온(2.64) 순으로 컸고, 변화 주기와 관련된 계수 b를 볼 때 기온(87.2) ≧ 토양표면 온도(79.3 ∼ 107) ≧ 식물 표면 온도(70.0 ∼ 105) 순으로 길었으며, 변화 변곡점 시기와 관련된 계수 c를 볼 때 기온(0.69) ≧ 토양표면 온도(-0.54 ∼ 3.15) > 식물 표면 온도(-2.54 ∼ 2.69) 순으로 빨랐다. 또한, 계수 b와 c의 상관관계는 고도의 유의성 있는 정비례 관계를 나타낸 것으로 볼 때, 기온과 토양표면 온도 및 식물 표면 온도 모두 변화 주기가 길수록 변화 변곡점을 나타내는 시기가 빨랐다(Fig. 14).
4. 결론
본 연구는 지표 기온과 4종의 식물 표면 온도 및 해당 식물이 생육하고 있는 토양의 표면 온도를 42회 monitoring 하여 구명한 결과는 다음과 같다.
첫째, 기온 1oC 증가에 따른 토양표면 온도 증가율은 0.80 ∼ 1.17oC/oC 이었다.
둘째, 기온 1oC 증가에 따른 식물 표면 온도 증가율은 0.88 ∼ 1.19oC/oC 이었다.
셋째, 기온과 토양표면 온도와의 차이는 토양표면 온도와 강한 상관관계를 보였다.
넷째, 기온과 식물 표면 온도와의 차이는 모두 기온과는 통계적으로 유의성 있는 상관관계를 보여주지 않았으나, 식물 표면 온도와는 모두 직선적인 부의 상관관계를 보였다.
다섯째, 기온과 식물 및 토양의 표면 온도에 대한 추정모형을 trigonometric function 형태인 four-parameter sine-wave model (TFM)로 선정하고 최소제곱법에 따라 오차가 최소인 계수를 산정하였다(1).
(1) |
단, X : 년 중 해당 순의 순서 [1(1월 상순) ∼ 36(12월 하순)]
yo, a, b, c : 계수
여섯째, 기온과 토양표면 온도 및 식물 표면 온도의 RMSE는 각각 1.75, 1.90 ∼ 2.41oC 범위 및 1.85 ∼ 2.21oC 범위로서 모두 낮은 편이었고, NSE는 각각 0.51, 0.37 ∼ 0.58 범위 및 0.39 ∼ 0.61 범위로서 모두 높은 편이었으며, 모델 검증 이론에 근거하여 판단할 때 본 연구의 모형(TFM)들은 모두 『적절하다』라고 판단되었다.
일곱째, 기온과 토양표면 온도 및 식물 표면 온도 모두 변화 주기가 길수록 변화 변곡점을 나타내는 시기가 빨랐다.
본 연구는 위 결과와 함께 다음과 같은 시사점을 준다.
기온 1oC 증가에 따른 세 가지 나무의 표면 온도 증가율이 토양표면 온도 증가율보다 더 높았으나 고추는 그와 반대로 토양표면 온도 증가율이 고추 표면 온도 증가율보다 더 높았다는 것은, 본 연구의 가설과 같이 작물과 나무가 기온 변화에 서로 다르게 반응하는 것으로 사료 된다, 또한, 본 연구의 식물 및 토양표면 온도에 대한 여덟 가지 온도 추정모형들의 계수 분석에서 계수 b 값과 계수 c 값이 직선적인 상관관계가 있다는 것은 토양 및 식물 표면 온도의 변화 양상(Fig. 13, G2)은 일반적인 온도의 주기적 변화 양상(Fig. 13, G1)과 비하여 변화 주기가 길어질수록, 변곡점 발생 시기도 빨라지는 것으로 나타났다. 이와 같은 결과들은 향후 기후변화 관련 연구에 중요한 의미를 부여한다고 사료 된다.
Acknowledgments
본 논문은 농림축산식품부의 재원으로 농림식품기술기획평가원의 농식품기술융합창의인재양성사업의 지원을 받아 연구되었음(과제번호: 120017-02).
References
-
Bayatvarkeshi M, Bhagat SK, Mohamma di K, Kisi O, Farahani M, Hasani A, Deo R, Yaseen ZM. 2021. Modeling soil temperature using air temperature features in diverse climatic conditions with complementary machine learning models. Computers and Electronics in Agriculture. 185:106158.
[https://doi.org/10.1016/j.compag.2021.106158]
-
Bertoldi G, Notarnicola C, Leitinger C, Endrizzi S, Zebisch M, Chiesa SD, Tappeiner U. 2010. Topographical and eco hydrological controls on land surface temperature in an alpine catchment. Eco hydrology 3(2):189-204
[https://doi.org/10.1002/eco.129]
-
Chen S, Mao X, Barry DA, Yang J. 2019. Model of crop growth, water flow, and solute transport in layered soil. Agricultural Water Management. 221(2019): 160-174.
[https://doi.org/10.1016/j.agwat.2019.04.031]
-
Chi Y, Sun J, Sun Y, Liu S, Fu Z. 2020. Multi-temporal characterization of land surface temperature and its relationships with normalized difference vegetation index and soil moisture content in the Yellow River Delta, China. Global Ecology and Conservation 23(2020):e01092
[https://doi.org/10.1016/j.gecco.2020.e01092]
-
Derradji M, Aiche M. 2014. Modeling the soil surface temperature for natural cooling of buildings in hot climates. Procedia Computer Science 32(2014):615-621.
[https://doi.org/10.1016/j.procs.2014.05.468]
- Doorenbos J, Pruitt WO. 1977. Guidelines for predicting crop water requirements. FAO Irrig. and Drainage Paper No.24. Rome : 144.
-
Eleftheriou D, Kiachidis K, Kalmintzis G, Kalea A, Bantasis C, Koumadoraki P, Spathara ME, Tsolaki A, Tzampazidou MI, Gemitzi A. 2018. Determination of annual and seasonal daytime and nighttime trends of MODIS LST over Greece-climate change implications. Sci. Total Environ. :616-617, 937-947.
[https://doi.org/10.1016/j.scitotenv.2017.10.226]
- Eom KC, Son ER, Ryu KS. 1990. Changes in evapotranspiration and soil water status to vinyl mulching. Korean J. Soil Sci. & Fert. 23: 100-106.
- Eom KC, Jung PK, Choi SH, Kim TW, Yoo SY, Park SH, Sonn YK. 2010. Water requirement of red pepper in different growth stages. Korean J. Soil Sci. & Fert. 43: 844-847.
-
Eom KC, Lee BK, Kim YS, Eom HY. 2014. Comparison of the change in daily air and surface temperature of red-pepper leaf and soil. Korean J. Soil Sci. & Fert. 47: 345-350.
[https://doi.org/10.7745/KJSSF.2014.47.5.345]
-
Eom KC, Kim LY, Im CY, Park YH, Park MS. 2020. Advanced analysis of climate data by trigonometric function, polar coordinate and scaling technique. J. Climate Change Res. 11 : 529-538.
[https://doi.org/10.15531/KSCCR.2020.11.5.529]
-
Ferreira LS, Duarte DHS. 2019. Exploring the relationship between urban form, land surface temperature and vegetation indices in a subtropiocal megacity. Urban Clim. 27:105-123.
[https://doi.org/10.1016/j.uclim.2018.11.002]
-
Frot E, Van Wesemael B, Benet AS, House MA. 2008, Water harvesting potential in function of hillslope characteristics: a case study from the Sierra de Gador. J. Arid Environ. 72(2008):1213-1231.
[https://doi.org/10.1016/j.jaridenv.2007.12.009]
-
Fu Xl, Yu Zb, Ding Yj, tang Y, Lu Hs, Jiang Xl, Ju Q. 2018. Analysis og influence of observation operator on sequential data assimilation through soil temperature simulation with common land model. Water Sci. and Engineering, 11(3):196-204.
[https://doi.org/10.1016/j.wse.2018.09.003]
-
Fuamba M, Branger F, Braud I, Batchabani E, Sanzana P, Sarrazin B, Jankowfsky S. 2019. Value of distributed water level and soil moisture data in the evaluation of a distributed hydrological model: Application to the pumma model in the Mercier catchment in France. J. Hydrology 569(2019):753-770.
[https://doi.org/10.1016/j.jhydrol.2018.12.035]
-
Goodman LA. 1960. On the exact variance of products. J. Am. Statist. Ass.55 : 708-713.
[https://doi.org/10.1080/01621459.1960.10483369]
-
Han Y, Wang Y, Zhao Y. 2010. Estimating soil moisture conditions of the Greater Changbai Mountains by land surface temperature and NDVI. IEEE T. Geosci. Remote 48(6):2509-2515.
[https://doi.org/10.1109/TGRS.2010.2040830]
-
Huang R, Zhang C, Huang J, Zhu D, Wang I, Liu J. 2015. Mapping of daily mean air temperature in agricultural regions using daytime and nighttime land surface temperature derived from TERRA and AQUA MODIS data. Remote Sens. 7(7): 8728-8756.
[https://doi.org/10.3390/rs70708728]
-
Irsyard al MI, Halog A, Nepal R. 2019. Renewable energy for climate change mitigation : An analysis of uncertanity and errors. Renewable Energy 130 : 536-546.
[https://doi.org/10.1016/j.renene.2018.06.082]
-
Islam MA, Lubbad R, Amiri SAG, Isaev V, Shevchuk Y, Uvarova AV, Afzal MS, Kumar A. 2021. Modelling the seasonal variations of soil temperatures in the Arctic coasts.Polar Science.
[https://doi.org/10.1016/j.polar.2021.100732]
-
Karnieli A, Agam N, Pinker RT, Anderson M, Imhoff ML, Gutman GG, Panov N, Goldberg A. 2010. Use of NDVI and land surface temperature for drought assessment merits and limitations. J. Clim. 23(3):618-633.
[https://doi.org/10.1175/2009JCLI2900.1]
- Kendal MG, Stuart A. 1963. The advanced theory of statistics. Vol. 1 ; Charles Griefin and Co., London. : 228-245.
-
Kim M, Lee WK, Nam K, Yu H, Choi SE, Kim CG. 2014. Estimating Stand Volume Pinus densiflora Forest Based on Climate Change Scenario in Korea. Journal of Korean Forest Society 103(1):105-112
[https://doi.org/10.14578/jkfs.2014.103.1.105]
- KMA (Korea Meteorological Administration). 2020. Korean climate change assessment report 2020:34-35,234-249.
-
Kloog I, Nordio F, Coull BA, Schwartz, J. 2014. Predicting spatiotemporal mean air temperature using MODIS satelite surface temperature measurements across the Northeastern USA, Remote Sens. Environ. 150:132-139.
[https://doi.org/10.1016/j.rse.2014.04.024]
-
Lee BK, Jung PK, Lee WK, Lim CH, Eom KC. 2015. Changes in air temperature and surface temperature of crop leaf and soil. J. Climate Change Res.:6(3): 209-221.
[https://doi.org/10.15531/ksccr.2015.6.3.209]
-
Li X, Zhou Y, Asrar GR, Zhu Z. 2018. Creating a seamless 1 km resolution daily land surface temperature data set for urban and surrounding area in the conterminous United States. Remote Sens. Environ. 206:84-97.
[https://doi.org/10.1016/j.rse.2017.12.010]
-
Li Z, Becker F. 1993. Feasibility of land surface temperature and emmissivity determination from AVHRR data. Remote Sens. Environ. 43(1):67-85.
[https://doi.org/10.1016/0034-4257(93)90065-6]
- Mandel J. 1964. The statistical analysis of experimental data. John Wiley & Sons, New York : 58-77, 363-389.
-
Moriasi DN, Armold JG, Van Liew MW, Bingner RL, Harmel RD, Veith TL. 2007. Model evaluation guidelines for systematic quantification of accuracy in watershed simulations. T Asabe, 50(3):885-900.
[https://doi.org/10.13031/2013.23153]
-
Moriasi DN, Gitau MW, Pai N, Daggupati P. 2015. Hydrologic and water quality models: Performance measures and evaluation criteria. Transactions of the American Soc. Agri. Bio. Eng. 58(6):1763-1785.
[https://doi.org/10.13031/trans.58.10715]
-
Muro J, Strauch A, Heinamann S, Steinbach S, Thonfeld F, Waske B, Diekkruger B. 2018. Land surface temperature trends as indicator of land use changes in wetlands. Int. J. Appl. Earth Obs. 70:62-71.
[https://doi.org/10.1016/j.jag.2018.02.002]
-
Naseri F, Azari M, Dastorani MT. 2021. Spatial optimization of soil and water conservation practices using coupled SWAT model and evoluyionary algorithm. International Soil and Water Conservation Research.
[https://doi.org/10.1016/j.iswcr.2021.04.002]
-
Nash JE, Sutcliffe JV. 1970. River flow forecasting through conceptual models, Part-I – a discussion of principles. Journal of Hydrology. 10(3):282-290.
[https://doi.org/10.1016/0022-1694(70)90255-6]
-
Nruthya K, Srinivas VV. 2015. Evaluating methods to predict stream flow at Ungauged Sites using regional flow duration curves: a case study. 2015. Aquatic Procedia (4):641-648.
[https://doi.org/10.1016/j.aqpro.2015.02.083]
-
Owen TW, Carlson TN, Gillies RR. 1998. An assessment of satellite remotely sensed land cover parameters in quantitatively describing
[https://doi.org/10.1080/014311698215171]
-
Paul KJ, Polglase PJ, Smethurst PJ, O’Connell AM, Carlyle CJ, Khanna PK. 2004. Soil temperature under forests: a simple model for predicting soil temperature under a range of forest types. Agricultural and Forest Meteorology 121:3-4.the climate effect of urbanization. Int. J. Rem. Sens. 19:1663-1681.
[https://doi.org/10.1016/j.agrformet.2003.08.030]
-
Qin Z, Kamieli A, Berliner P. 2001. A mono-window algorithm for retrieving land surface temperature from Landsat TM data and its application to the Israel-Egypt border region. Int. J. Rem. Sens. 22(18):3719-3746.
[https://doi.org/10.1080/01431160010006971]
- Rattan L, Manoj KS. 2004. Principles of soil physics.: 269-271, Marcel Dekker, Inc. New York·Basel, USA.
-
Romanovsky V, Osterkamp T. 1997. Thawing of the active layer on the coastal plain of the alaskan arctic. Permaft. Periglac. Process 8(1):1-22.
[https://doi.org/10.1002/(SICI)1099-1530(199701)8:1<1::AID-PPP243>3.0.CO;2-U]
-
Shein EV, Dembovetsky AV, Panina SS. 2013. Modeling soil water movement under low head ponding and gravity infiltration using data determined with different methods. Proc. Environ. Sci. 19(2013):553-557.
[https://doi.org/10.1016/j.proenv.2013.06.062]
-
Sofyan SE, Hu E, Kotousov A, Riayatsyah TMI, Khairil, Hamdani. 2020. A new approach to modeling of seasonal soil temperature fluctuations and their impact on the performance of a shallow borehole heat exchanger. Case Studies in Thermal Engineering. 22(2020):100781.
[https://doi.org/10.1016/j.csite.2020.100781]
-
Son NT, Chen CF, Chen CR, Chang LY, Minh VO. 2012. Monitoring agricultural drought in the Lower Mekong Basin using MODIS NDVI and land surface temperature data. Int. J. Appl. Earth Obs. 18(1):417-427.
[https://doi.org/10.1016/j.jag.2012.03.014]
-
Urqueta H, Jodal J, Herrera C, Wilke HG, Medina A, Urrutia J, Custodio E, Rodriguez J. 2018. Land surface temperature as an indicator of the unsaturated zone thickness: a remote sensing approach in the Atacama Desert. Sci. Total Environ. 612:1234-1248.
[https://doi.org/10.1016/j.scitotenv.2017.08.305]
-
Vancutsem C, Crecatio P, Dinku T, Connor SJ. 2010. Evaluation of MODIS land surface temperature data to estimate air ntemperature in different ecosystems over Africa. Remote Sens. Environ. 114(2):449-465.
[https://doi.org/10.1016/j.rse.2009.10.002]
-
Verhoef A, van den Hurk BJ, Jacobs AF, Heusinkveld BG. 1996. Thermal soil properties for vineyard (EFEDA-1) and savanna (HAPEX-sahel) sites. Agricult. Forest Meteorol. 78(1-2):1-18.
[https://doi.org/10.1016/0168-1923(95)02254-6]
-
Wang XC, Li J. 2010. Evaluation of crop yield and soil water estimates using the epic model for the loess plateau of China. Mathematical and Computer Modelling. 51(2010):1390-1397.
[https://doi.org/10.1016/j.mcm.2009.10.030]
-
Watson J, Challinor A. 2013. The relative importance of rainfall, temperature and yield data for a regional-scale crop model. Agricultural and forest Meteorology 170(2013):47-57.
[https://doi.org/10.1016/j.agrformet.2012.08.001]
-
Weng Q, Lu D, Schubring J. 2004. Estimation of land surface temperature-vegetation abundance relationship for urban heat island studies. Remote Sens. Environ. 89:467-483.
[https://doi.org/10.1016/j.rse.2003.11.005]
-
Xu C, Qu JJ, Hao X, Zhu Z, Gutenberg L. 2020. Surface soil temperature seasonal variation estimation in a forested area using combined satellite observations and in-situ measurements. Int. J. Appl. Earth Obs. Geoinformation 91(2020):102156.
[https://doi.org/10.1016/j.jag.2020.102156]
-
Ye L, Xiong W, Li Z, Yang P, Wu W, Yang G, Fu Y, Zou J, Chen Z, van Ranst E, Tang H. 2013. Climate change impact on China food security in 2050. Agronomy for Sustainable Development. 33:363-374.
[https://doi.org/10.1007/s13593-012-0102-0]
-
Yoo C, Im J, Park S, Quackenbush LJ. 2018. Estimation of daily maximum and minimum air temperature in urban landscapes using MODIS time satellite data. ISPRS J. Photogramm. Remote Sens. 137:149-162.
[https://doi.org/10.1016/j.isprsjprs.2018.01.018]
-
Zhang Y, Odeh IOA, Han C. 2009. Bi-temporal characterization of land surface temperature in relation to imprvious surface area. NDVI and NDBI, using a sub-pixel image analysis. Int. J. Appl. Earth Obs. 11(4):256-264.
[https://doi.org/10.1016/j.jag.2009.03.001]
-
Zhang T, Huang JC, Lei Q, Liang X, Lindsey S, Luo J, Zhu AX, Bao W, Liu H. 2021. Catena 208:105696.
[https://doi.org/10.1016/j.catena.2021.105696]
-
Zhu W, Lu A, Jia S. 2013. Estimation of daily maximum and minimum air temperature using MODIS land temperature products. Remote Sens. Environ. 130:62-73.
[https://doi.org/10.1016/j.rse.2012.10.034]