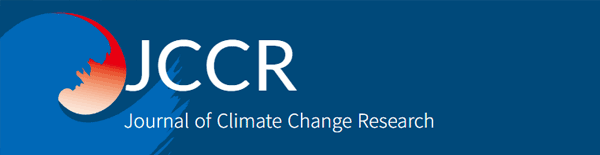
Prediction of forest fire risk according to climate change in Bhutan using a shared socioeconomic pathways (SSP) scenario and random forest
Abstract
Forest fires destroy millions of forest acres globally, damaging ecosystem services, emitting carbon into the atmosphere, and causing billions of dollars in socio-economic losses, including loss of life. Bhutan, despite its small size, is grappling with forest fires compounded by climate extremes, a remote location, and a lack of preparedness. Under such conditions, it is important to develop policy, technology, and action to respond to forest fires based on scientific research. This study used the Random Forest model (RF) and Shared Socioeconomic Pathways (SSP) approach to create a model for predicting forest fires in Bhutan by considering climate data, spatial data, and forest fire occurrence factors. The RF algorithm was used to analyze the correlations between various input data and predict the change in future forest fire risk in Bhutan. Using SSP scenarios that considered changes due to future population and economic growth, it was possible to predict the risk of forest fires accurately and spatially in Bhutan. The study found that the average forest fire risk was highest during the months of October to January, which are also the dry seasons with low precipitation. However, it also showed high variability across climate change scenarios. SSP scenarios also confirmed the possibility of a significant increase in forest fire risk in the future. The results of this study can be used to support specific policy decisions for forest fire prevention and response in Bhutan and to contribute to the development of forest fire prediction technology in the light of the changing global climate.
Keywords:
Forest Fire, Random Forest Model, Bhutan, Risks, Climate Change1. Introduction
Forest fires are a disaster that can damage people and the environment in a lot of ways, and they happen all the time (Bowman et al., 2020). In particular, as climate change makes forest fires more frequent and severe, damage from forest fires is also increasing, and forests are being lost as a result (Jones et al., 2022). Consequently, forest loss due to forest fire has global consequences in the era of climate change as it leads to reduced ecosystem services and the loss of environmental assets (Bowman et al., 2009). Since the degree of climate change varies depending on human response, forest fire outbreaks have a negative effect on climate change. As a result, a vicious cycle of climate change occurs in which the risk of forest fires increases again due to climate change. Accordingly, the development and application of forest fire prediction technology are critical to conserving forest resources and their consequent ecosystem services. Forest fires are caused by a wide variety of causes due to a combination of natural and anthropogenic factors (Neary et al., 1999). Therefore, it is necessary to make a comprehensive analysis and prediction by considering various factors related to forest fire occurrence, and in this regard, the development of a forest fire prediction model is in progress. While there exist multiple models to analyze forest fires and predict future risks, the Random Forest (RF) model is popularly used to develop prediction models, especially for forest fires (Tyralis et al., 2019; Ziegler and König, 2014). The RF model has great prediction performance and the ability to mitigate overfitting issues (Fawagreh et al., 2014; Hastie et al., 2009; More and Rana, 2017). Such studies have improved our ability to monitor and respond to increasing incidences of forest fires, especially under global environmental and climate changes (Bjånes et al., 2021; Eslami et al., 2021; Gigović et al., 2019; Latifah et al., 2019; Milanović et al., 2021; Song et al., 2017).
Despite its small size, the forest of Bhutan is experiencing a dramatic increase in the frequency of forest fires and the area burned over the years (Rai, 2023). According to Rai (2023), forest fire incidences and area damaged in Bhutan increased from 40 cases destroying 352.72 hectares in 2019 to 43 cases destroying 4308.05 hectares in 2021 alone. Although there have been few studies on Bhutan’s adaptive capacity to deal with forest fires, research on forest fire occurrence, prediction, and risk is very scarce (Tshering et al., 2020; Vilà-Vilardell et al., 2020). Therefore, this study aims to: i) spatially analyze the risk of forest fire occurrence and identify the most influential factors by utilizing spatial information, field survey data, past occurrence history, and climate data of Bhutan; and ii) develop a forest fire prediction model using machine learning by integrating climate change scenario data to predict and analyze future forest fire risk changes according to climate change patterns of Bhutan. As a result, the spatial distribution of forest fire risk is analyzed by integrating various factors through the machine learning (ML) model and analyzing the time period with the greatest risk. In addition, future climate change scenarios are applied to the developed ML model to analyze and evaluate changes in future forest fire risk.
In this study, modeling was conducted by collecting various input variables, such as climate data, spatial data, and forest fire history data in Bhutan. The RF model algorithm is proven to produce highly reliable results for forest fire studies (Gao et al., 2023; Oliveira et al., 2012; Pang et al., 2022). Our study used the RF model to predict forest fires by analyzing the correlation between various input data. In addition, to consider future climate change, the Shared Socioeconomic Pathways (SSP) scenario is used to reflect the future climate change situation in the RF model. Since the SSP scenario is a climate change scenario that considers future population and economic growth, it can play a more important role in the study of forest fires than the Representative Concentration Pathways (RCP) scenario, in which anthropogenic factors affect their occurrence. In addition, since the SSP scenario provides climate change data according to the degree of management, the effect of the degree of management of climate change on forest fire risk can be analyzed.
2. Methods and materials
2.1. Study area
Bhutan is a small (38,394 km2) mountainous country situated between 27°30' N latitude and 90°30' E longitude, sandwiched between India and China (Fig. 1). Bhutan is highly mountainous, with almost 95% of the country’s total land area situated above 6000 m above mean sea level (Tshering et al., 2020). The vegetation of the country changes along its rapidly changing elevation, from 97 m in the southern foothills to over 7000 m in the northern mountains (MoAF, 1997).
Vegetation consists mainly of subtropical broadleaved forests up to 1200 m, followed by temperate cool broadleaved forests between 1200 m and 2000 m. On the coniferous front, pure pine forests comprised of blue pine (Pinus wallichiana) and sometimes mixed with oaks and rhododendron are found between 1500 m and 3200 m. Mixed coniferous forests comprised of fir (Abies densa), hemlock (Tsuga dumosa), spruce (Picea spinulosa), larch (Larix griffithii), and juniper (Juniperus indica) are found between 2500 m and 3200 m. In addition, pure stands of chir pine (Pinus roxburgii) are found in dry areas between 1520 m and 1860 m (Wangda and Ohsawa, 2006). The remoteness of the mountainous areas, coupled with steep terrain and a mostly forested area, makes Bhutan highly susceptible to forest fires. The country experiences four seasons: spring (March to May), summer (June to August), autumn (September to November), and winter (December to February). The summer seasons are characterized by high precipitation from monsoons, while winter and spring are dry with high winds, especially during late winter and spring. These geographical and climatic characteristics increase the likelihood of forest fires and act as important variables in developing forest fire prediction models. Therefore, in this study, information such as the topography, geographical characteristics, and climate of Bhutan are comprehensively analyzed to evaluate the possibility of forest fires and develop a forest fire prediction model. Through this, we expect to be able to take more accurate and effective measures to cope with and prevent forest fires in Bhutan.
2.2. Data collection and preprocessing
Various factors were collected to predict the risk of forest fires in Bhutan. Representative data include occurrence history data from 2007 to 2017, geospatial factors, meteorological factors, and future climate scenario factors. These variables can be useful for forest fire prediction because they represent geographic and meteorological conditions related to forest fire occurrence (Lin et al., 2023). Forest fire data from 2007 to 2017 was collected from the fire databases of the department of forests, field offices, and extension officers. Details of this data include the geographical location of forest fires, date, fire start time, duration, and end of fire, cause of fires, area damaged, property losses, and other factors such as meteorological, environmental, and social. This data was further ground-toothed and confirmed with local government officers and community members. Where GPS readings were not consistent or missing, ground truthing was done to obtain GPS readings, and where ground truthing was not possible, Google Earth was used by field researchers who knew the areas well to pick GPS locations. Meteorological data, including mean temperature, accumulated precipitation, mean relative humidity, and maximum wind speed, were collected from the department of hydrology and meteorology of Bhutan.
Studies by Rubí et al. (2023) and Chuvieco and Congalton (1989), confirmed altitude, slope, and aspect as the spatial factors that affect forest fires. Therefore, the DEM data based on the shuttle radar topography mission (SRTM) data was used to generate the altitude, aspect, and slope data in the Bhutan region with a resolution of 30 m (Fig. 2). Next, the meteorological factors that affect forest fires include average humidity, average temperature, average wind speed, and daily precipitation (Sharples et al., 2009; Van Wagner, 1987). To train this data into the RF model, a history of forest fires is required. Therefore, forest fire history data from 2007 to 2017 were acquired from the department of forests, and field observation was utilized (Fig. 2d). Each point in Fig. 2d includes the time of occurrence, and all points have meteorological data at the time of occurrence. Finally, to predict the change in forest fire risk according to future climate conditions using the trained model, global SSP data provided by the Korea Meteorological Administration was used to secure future climate data with the same items as climate factors. For the SSP scenarios, SSP1-2.6, a scenario in which climate change was moderately progressed due to good management, and SSP5-8.5, in which climate change was severely progressed due to poor management, were used according to the degree of management of climate change. In order to reflect future climate change, SSP data for 2030, 2040, and 2050 were used. The collected data was first presented as spatial data and then converted into a comma separated value (CSV) file format. In the data preprocessing process, missing values were processed, and outliers were removed. In addition, the problem of multicollinearity was solved through correlation analysis between variables.
2.3. Random forest model
For forest fire prediction, the RF model was used in this study. The RF model is a machine learning algorithm for constructing a predictive model using multiple decision trees with multiple inputs. The RF model has the advantage of mitigating the overfitting problem and showing high predictive performance (Fawagreh et al., 2014; Hastie et al., 2009). Therefore, RF models are used to develop predictive models in various fields, including forest fire (Tyralis et al., 2019; Ziegler and König, 2014). By analyzing the importance of features’ in the RF model, variables that affect forest fire occurrence were identified. By analyzing the features importance, variables needed to improve the forest fire prediction model were identified.
First, in order to develop an RF model for forest fire prediction, the Bhutan forest fire history data collected in Fig. 2d and meteorological data such as temperature, humidity, wind direction, and wind speed at the time of occurrence are used to train the model. Using the trained model, the month with the highest forest fire risk is analyzed, and the spatial distribution of forest fire risk is identified. Then, using the same SSP scenario as the trained meteorological factors, the change in forest fire risk due to future climate change is analyzed according to the scenario. Through this, this study predicts changes in forest fire risk due to climate change and proposes reasonable forest fire risk response plans accordingly (Fig. 3).
3. Results and discussion
Forest fire risk maps of Bhutan are presented in Fig. 4 based on a trained RF model using historical data. Fig. 4a is the forest fire risk on July 15th, 2009, when the forest fire risk is the lowest, and Fig. 4b is the forest fire risk on March 13th, 2009. When comparing the two, it was found that the distribution of forest fire risk varies with weather conditions at low altitudes. July is the rainy season in Bhutan, and March is the dry season. Thus, even in the same year, the distribution of forest fire risk is different. In the case of the northern region with high altitude, it was found that the risk of forest fire occurrence was low at two time points. This result is also shown in the importance of the input data of the RF model, and it was found that the importance of the altitude (DEM in Fig. 5) was overwhelmingly higher than that of other input data (Fig. 5).
Through the model trained above, a total of 36 monthly forest fire risks were calculated according to the SSP 1-2.6 and 5-8.5 scenarios in 2030, 2040, and 2050. To compare the above results, a histogram statistical analysis was performed to calculate the mean values of all pixel values, and the changes in forest fire risk distribution according to climate change scenarios were analyzed by listing them in chronological order (Fig. 6). he average forest fire risk was found to be highest during the months of October to January. In particular, from September to October, the risk of forest fires rises sharply, which overlaps with Bhutan's dry season, when precipitation decreases and dryness increases. In particular, October is evaluated as having a high risk of forest fires because it is a period in which precipitation is low but the temperature does not drop and the temperature is maintained while being dry. The risk of forest fires in the wet season appears to be low at all time points in all scenarios, but the risk of forest fires in the dry season shows high variability across climate change scenarios, which appears to be affected by the increase in extremes of climate change. Fig. 7 lists the mean, maximum, and minimum values of forest fire risk according to SSP1-2.6 and SSP5-8.5 in chronological order. In the SSP5-8.5 scenario, where the degree of management of climate change is low, the forest fire risk is higher than in the case of SSP1-2.6, where the degree of management is high. Through this, as passive management of climate change increases the risk of forest fires, the mean and max of forest fire risk are identified as high.
Modeling with the RF model and SSP scenarios made it possible to spatially predict where and when forest fire risk is high and how human responses to climate change affect forest fire risk in Bhutan. This can help policymakers in Bhutan make decisions about how to prevent and deal with forest fires and climate change based on scientific evidence. In addition, the study results are expected to make a great contribution to the development of forest fire prediction technology globally. Further, the modeling method using SSP scenarios is expected to be used in the field of future prediction as well.
4. Conclusion
In this study, the spatial pattern of forest fire risk in Bhutan was analyzed, and future risk was predicted using the RF model and SSP scenarios. As a result of the study, the variables that had the greatest impact on forest fire occurrence were altitude, daily minimum temperature, slope, and wind speed, and a RF model was constructed considering these variables. The spatial distribution of forest fire risk was confirmed considering the relevant factors, and it was found that the effect of altitude was the greatest regardless of weather conditions, and the area with high risk was the area where human impact could occur. As a result of applying the SSP scenario, it is predicted that the possibility of forest fire risk in Bhutan will increase over the next 50 years in the SSP5-8.5 scenario compared to the SSP1-2.6 scenario. In both scenarios, the forest fire risk was found to be high during the dry season in Bhutan, and the passive response to climate change increased the forest fire risk. Additionally, the spatial distribution of forest fire risk was found to be the same in future climate scenarios. As a result, active responses to climate change can reduce forest fire risk, and as a result, the extent of climate change will also decrease, enabling positive feedback that reduces forest fire risk.
Acknowledgments
This research was supported by the National Research Foundation of Korea Grant funded by the Korean government (MSIT) (NRF-2020R1I1A1A0107432714) and the OJEong Resilience Institute (OJERI) at Korea University as the Core Research Institute Basic Science Research Program through the National Research Foundation of Korea (NRF) (NRF-2021R1A6A1A10045235).
References
-
Bjånes A, De La Fuente R, Mena P. 2021. A deep learning ensemble model for wildfire susceptibility mapping. Ecological Informatics. 65: 101397.
[https://doi.org/10.1016/j.ecoinf.2021.101397]
-
Bowman DMJS, Balch JK, Artaxo P, Bond WJ, Carlson JM, Cochrane MA, ... Pyne SJ. 2009. Fire in the earth system. Science. 324(5926): 481-484.
[https://doi.org/10.1126/science.1163886]
-
Bowman DMJS, Kolden CA, Abatzoglou JT, Johnston FH, van der Werf GR, Flannigan M. 2020. Vegetation fires in the anthropocene. Nature Reviews Earth & Environment. 1(10): 500-515.
[https://doi.org/10.1038/s43017-020-0085-3]
-
Chuvieco E, Congalton RG. 1989. Application of remote sensing and geographic information systems to forest fire hazard mapping. Remote Sensing of Environment. 29(2): 147-159.
[https://doi.org/10.1016/0034-4257(89)90023-0]
-
Eslami R, Azarnoush M, Kialashki A, Kazemzadeh F. 2021. GIS-based forest fire susceptibility assessment by random forest, artificial neural network and logistic regression methods. Journal of Tropical Forest Science. 33(2): 173-184.
[https://doi.org/10.26525/jtfs2021.33.2.173]
-
Fawagreh K, Gaber MM, Elyan E. 2014. Random forests: From early developments to recent advancements. Systems Science & Control Engineering. 2(1): 602-609.
[https://doi.org/10.1080/21642583.2014.956265]
-
Gao C, Lin H, Hu H. 2023. Forest-fire-risk prediction based on random forest and backpropagation neural network of Heihe area in Heilongjiang province, China. Forests. 14(2): 170.
[https://doi.org/10.3390/f14020170]
-
Gigović L, Pourghasemi HR, Drobnjak S, Bai S. 2019. Testing a new ensemble model based on SVM and random forest in forest fire susceptibility assessment and its mapping in Serbia’s Tara National Park. Forests. 10(5): 408.
[https://doi.org/10.3390/f10050408]
-
Hastie T, Tibshirani R, Friedman JH. 2009. The elements of statistical learning: Data mining, inference, and prediction (2nd ed.). New York: Springer.
[https://doi.org/10.1007/978-0-387-84858-7]
-
Jones MW, Abatzoglou JT, Veraverbeke S, Andela N, Lasslop G, Forkel M, ... Quéré CL. 2022. Global and regional trends and drivers of fire under climate change. Reviews of Geophysics. 60(3): e2020RG000726.
[https://doi.org/10.1029/2020RG000726]
-
Latifah AL, Shabrina A, Wahyuni IN, Sadikin R. 2019. Evaluation of random forest model for forest fire prediction based on climatology over Borneo. Proceedings of 2019 International Conference on Computer, Control, Informatics and its Applications (IC3INA); 2019 Oct 23 ~ Oct 24; Tangerang, Indonesia: 4-8.
[https://doi.org/10.1109/IC3INA48034.2019.8949588]
-
Lin X, Li Z, Chen W, Sun X, Gao D. 2023. Forest fire prediction based on long- and short-term time-series network. Forests. 14(4): 778.
[https://doi.org/10.3390/f14040778]
-
Milanović S, Marković N, Pamučar D, Gigović L, Kostić P, Milanović SD. 2021. Forest fire probability mapping in eastern serbia: Logistic regression versus random forest method. Forests. 12(1): 1-17.
[https://doi.org/10.3390/f12010005]
- MoAF (Ministry of Agriculture and Forests). 1997. Atlas of Bhutan. Thimphu, Bhutan: Land use planning section (LUPS), policy and planning division.
-
Neary DG, Klopatek CC, DeBano LF, Ffolliott PF. 1999. Fire effects on belowground sustainability: A review and synthesis. Forest Ecology and Management. 122(1-2): 51-71.
[https://doi.org/10.1016/S0378-1127(99)00032-8]
-
Oliveira S, Oehler F, San-Miguel-Ayanz J, Camia A, Pereira JMC. 2012. Modeling spatial patterns of fire occurrence in Mediterranean Europe using multiple regression and random forest. Forest Ecology and Management. 275: 117-129.
[https://doi.org/10.1016/j.foreco.2012.03.003]
-
Pang Y, Li Y, Feng Z, Feng Z, Zhao Z, Chen S, Zhang H. 2022. Forest fire occurrence prediction in China based on machine learning methods. Remote Sensing. 14(21): 5546.
[https://doi.org/10.3390/rs14215546]
- Rai M. 2023. Human carelessness is the leading cause of forest fires in Bhutan; [assessed 2023 May 3]. https://thebhutanese.bt/human-carelessness-is-the-leading-cause-of-forest-fires-in-bhutan/
-
Rubí JNS, de Carvalho PHP, Gondim PRL. 2023. Application of machine learning models in the behavioral study of forest fires in the Brazilian federal district region. Engineering Applications of Artificial Intelligence. 118: 105649.
[https://doi.org/10.1016/j.engappai.2022.105649]
-
Sharples JJ, McRae RHD, Weber RO, Gill AM. 2009. A simple index for assessing fire danger rating. Environmental Modelling & Software. 24(6): 764-774.
[https://doi.org/10.1016/j.envsoft.2008.11.004]
-
Song C, Kwan MP, Song W, Zhu J. 2017. A comparison between spatial econometric models and random forest for modeling fire occurrence. Sustainability. 9(5): 819.
[https://doi.org/10.3390/su9050819]
-
Tshering K, Thinley P, Tehrany MS, Thinley U, Shabani F. 2020. A comparison of the qualitative analytic hierarchy process and the quantitative frequency ratio techniques in predicting forest fire-prone areas in Bhutan using GIS. Forecasting. 2(2): 36-58.
[https://doi.org/10.3390/forecast2020003]
-
Tyralis H, Papacharalampous G, Langousis A. 2019. A brief review of random forests for water scientists and practitioners and their recent history in water resources. Water. 11(5): 910.
[https://doi.org/10.3390/w11050910]
-
Vilà-Vilardell L, Keeton WS, Thom D, Gyeltshen C, Tshering K, Gratzer G. 2020. Climate change effects on wildfire hazards in the wildland-urban-interface - Blue pine forests of Bhutan. Forest Ecology and Management. 461: 117927.
[https://doi.org/10.1016/j.foreco.2020.117927]
- Wangda P, Ohsawa M. 2006. Gradational forest change along the climatically dry valley slopes of Bhutan in the midst of humid Eastern Himalaya. Plant Ecology. 186(1): 109-128.
-
Ziegler A, König IR. 2014. Mining data with random forests: Current options for real-world applications. Wiley Interdisciplinary Reviews: Data Mining and Knowledge Discovery. 4(1): 55-63.
[https://doi.org/10.1007/s11258-006-9116-5]