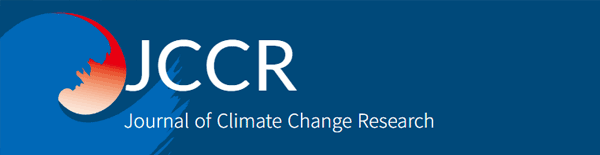
An Analytical Approach for CPS Preparation for Korea’s ODA: Focusing on Environment, Science and Technology Innovation Sector
Abstract
The Country Partnership Strategy (CPS) sets out an important milestone for development assistance. It provides the rational and guidance in overall Official Development Assistance (ODA) decision-making process at a high level from selecting priority countries, focus of assistance areas to the volume of aids. In current CPS preparation process, it involves diagnostic and analytical assessment on economic, social, security status and aspects of development ownership of the recipient country. Although not all development issues could be comprehensively covered by the CPS, the rational for priority sector selection and the process should be clearly established from its initial assessment stage and should appropriately reflect emerging and burdening development issues. This paper presented the potential for a macro-level diagnostic approach for prioritizing countries and its areas of development interests, especially in climate, environment and innovation context. With the use of aggregated country-level indicators, cluster analysis was applied to group countries by the similarities of its indicator value, and principal component analysis was conducted to identify contributing variables that best explains the attributes of each clusters. The attributes of each clusters were described and compared with the priority areas in the current CPS framework. As a result, the current framework prioritized assistance in sectors such as education, water and sanitation, transport and regional development that focuses on enhancing agricultural practices. This indicates that selection of priority sectors in the CPS preparation process should be firmly rooted in the analysis of development issues that could be initially assessed by analyzing appropriate indicators. Methodology proposed in this paper could be useful to prepare CPS in the future, additionally in the areas of environmental conservation, science and technology innovation for developing countries.
Keywords:
Country Partnership Strategy, Cluster Analysis, Principal Component Analysis, Global Indicators, Environmental Conservation, Science and Technology Innovation1. Introduction
The preparation of Country Partnership Strategy (CPS) in Overseas Development Assistance (ODA) was launched in 2005 according to the Paris Declaration. It was prepared to achieve better development results by encouraging recipient countries to have ownership and responsibility for their own development priorities (ADB, 2007). In order to promote mutual accountability both for donor and recipient countries, the CPS is closely aligned with the priorities and plans of developing countries in consultation with its development partners and relevant stakeholders. Such approach has helped formulate a better and relevant strategy of CPS by allowing participation and commitment of various stakeholders in the implementation process. In this context, the process for CPS preparation is important since it provides the rationale and guidance in overall ODA decision-making process at a high level from selecting priority partner countries, focus of assistance areas to the volume of aids.
Korea, as one of the members of OECD Development Assistance Committee (DAC), has continuously increased its ODA commitment for its 24 priority partner countries. The countries were selected based on their income level, political situation, diplomatic relations with Korea and economic cooperation potential. The CPS (2016-2020) was developed for respective 24 partner countries to allocate financial resources in the designated sectors including health, transport and telecommunication, education, agriculture and forestry etc. As indicated in the OECD report, Korea has committed a clear focus on education, health and transport which are higher than that of the EU and average of DAC members (Marx and Soares, 2013). Assistance in these sectors has intensified continuously that Korean government channeled 76.7 per cent of its bilateral assistance into the area of social infrastructure including healthcare and education, as well as in the area of economic infrastructure including transportation and logistics (OECD, 2018). As can be indicated through this engagement, Korea continued to build on its comparative advantage in key economic and social sectors which also reflect Korea’s experience in its own development journey. Korea has striven hard to contribute in increasing aid effectiveness not only in conventional development context and its relevant sectors but in areas that urges increased attention such as emerging niche in science and technology and climate change.
Regardless of its positive trajectory, these areas are too abstractly presented or does not appear to put in place a strategic plans that permeate through CPS. A few previous studies on CPS attempt to address these problems throughout selection and assessment process; focusing on improving country-specific CPS (Kim et al., 2014; Kang et al., 2015), developing criteria and indicators for selecting countries (Park et al., 2013) and recently, a growing interests are shown in preparing CPS results and evaluation framework which emphasizes preparing rigorous measures for promoting aid effectiveness (Jones, 2012; Cho S. 2013; Rankin, 2014; Choi and Kim, 2020). However in order to better coordinate emerging needs of developing countries, parallel to conventional approach to development cooperation, diversified and inclusive growth agenda should be mainstreamed into the design of CPS supported by various research approaches.
This paper intends to propose a particular interest to prepare CPS in the future, additionally, in the areas of climate change, environmental conservation and science and technology innovation. With the use of comprehensive global indicators representing macroeconomic dimensions of determinants of country’s competitiveness – economic, natural and social environment at the national level – it attempts to look for a relevant image of the countries’ grouping in these aspects. In this respect, the paper was structured in analyzing the following: comprehensive grouping by analyzing indicators, applying principal component analysis to identify determinant factors that best represent clusters, and providing policy recommendations for rebuilding the next phase of CPS that appropriately reflects environment and innovations in development context.
2. Material and methods
2.1 Data and Methodology
Three different data sets were prepared that has global coverage – namely CRI (Global Climate Risk Index), EPI (Environment Performance Index) and GCI (Global Competitiveness Index). The range of indicators of these indices were selected for analysis which does not necessarily overlap with each other. K-means clustering was applied twice by grouping GRI and EPI which have some degree of similarity and GCI alone as it shows distinctive dimension for competitiveness. The use of principal component analysis was followed to identify which determinant variables best represent each cluster and to reduce redundant variables. Lastly, cluster matrix was derived on the basis of clustering and principal component analysis which describes critical attributes of cluster combinations explained by determinant variables (Fig. 1).
The Global Climate Risk Index analyses to what extent countries and regions have been affected by impacts of weather-related events such as storms, floods, heat waves etc. It was developed by Germanwatch which quantifies the impacts of extreme weather events based on the data from the Munuch Re NatcatService that is considered to be of most reliable and complete databases globally on this matter. CRI comprehensively takes following data into account; death tool, deaths per 100,000 inhabitants, total losses in million US dollars, losses per unit GDP presented in per cent, and number of total events since 1999. By aggregating and calculating these variables, the countries are raked by the number between 1 to 100 in which the countries ranking highest are the ones most impacted and should consider the CRI as a warning sign that they are at risk of either frequent events or rare, but extraordinary catastrophes (Eckstein et al.,2019).
Environmental Performance Index is designed to measure the comparative performance of countries in the domain of environmental issues regarding protection of human health and ecosystem preservation. The first dimension assesses human health against the negative effects on the environment which is measured by the indicator as Health Impact, Air Quality and Water and Sanitation. Ecosystem vitality assesses ecosystem protection and resource management. Six categories - Water Resources, Agriculture, Forests, Fisheries, Biodiversity and Habitat, and Climate and Energy – are assessed at a country level. It summarized into a single number as EPI score, however, value for each indicators taken from original sources are also provided. It is comprised of 32 indicators for 180 countries which came from trusted sources like national government reports, the World Bank, the UN Development Program, and the World Resources Institute (Popescu et al., 2017; Wendling et al., 2020).
The Global Competitiveness Index provides detailed factors and attributes that drive country’s productivity, growth and human development which is based on the successive aggregations of scores from the indicator level. It is a result of three-year consultative process from international organizations and leading experts in respective fields which is designed to support countries to identify relevant policies and practices. It is comprised of 12 pillars; institutions, infrastructure, ICT adoption, macroeconomic stability, health, skills, product market, labour market, financial system, market size, business dynamism and innovation capability. For individual indicators, raw values are transformed into a score ranging from 0 to 100, with 100 being the ideal state. (Schwab, K.,2019)
Each index is comprises of more than 100 sub-indicators, however, only selective variables are considered for analysis that do not necessarily overlap with each other and are exclusive. We took GRI score, 11 variables from EPI that represent both ecosystem vitality and protection of human health. 12 variables are taken from GCI which shows country’s competitiveness when measured by both macroeconomic indicator and its innovative capability (Table 1).
The Korean government developed Country Partnership Strategies for each priority partner country to maximize synergy effects and to improve ODA effectiveness through strategic concentration (ODA Korea). It identifies the agreed priority areas where Korean government can provide support in achieving the partner country’s development strategy and poverty reduction goals, which is prepared in consultation with the government, development partners and other stakeholders. It outlines the specific development context of partner country, the rationale for selection of certain activities to achieve the goals (Kim et al., 2013). 24 priory partner countries out of 134 economies were selected in 2015 which are comprised of 11 in Asia, 7 Africa, 4 in Central and South American and 2 Middle East and CIS countries. Table 2 indicates focus assistance areas for respective partner countries.
As can be seen in Table 3, the assistance commitment was largely contributed to certain areas; water and sanitation, transport, regional development and education. The commitment for resolving issues regarding environment and climate change, could be reflected accordingly by the Mid-term Strategy for Development Cooperation, which described Korea’s commitment to expanding assistance in sectors such as economic infrastructure, the environment, health and education, and to mainstreaming the environment and climate change issues in its development cooperation policies (OECD DAC, 2017). In this context, it was reported that the Korean government understands the importance of addressing climate change and strengthened its support for developing countries in their efforts to deal with the issue.
2.2 K-means clustering and Principal Component Analysis
This research employed K-means clustering and principal component analysis to classify a set of similar countries categorized by its sub-indicator values and identify determinant components that best represent attributes of each clusters. K-means clustering is the most commonly used machine learning algorithms for partitioning a given data set into a set of k groups in which k represents the number of groups (MacQueen, 1967). It classifies objects in a number of clusters to make that objects within same cluster are as similar as possible. In K-means clustering each cluster is represented by its center or centroid which corresponds to the mean of points, average of all the points, assigned to the cluster (Popescu et al., 2017). Aggregated data sets are classified according to the hierarchical classification method which allows to identify behavioral similarities among the countries. The clustering algorithm aims to group the N row of objects into K clusters. An each stage, an observation or a cluster of observations is absorbed into another cluster. The following elements should be considered:
- N objects are to be clustered
- dij: the distance between clusters i and j
- cluster i contains ni objects
- D : the set of all remaining dij
The first is to find the smallest element dij remaining in D. The second step is to merge cluster i into j into a single new cluster k. Lastly, calculate a new set of distances dkm defined as follows:
(1) |
in which m represents any other cluster than k. New distances will replace dim and djm in D. The three steps are repeated until D contains a distinct group consisting of all objects and similarities decrease while these steps are reiterated. After determining the number of clusters the centroid, the average of points in the respective clusters are calculated.
(2) |
In equation 2, ci is the centroid of cluster ki, K is the number of clusters, ni is the number of objects in the ith cluster.
3. Results and Discussion
3.1 CRI-EPI K-means clustering analysis
K-means clustering analysis was performed on GRI and EPI data sets for 167 countries. A set of countries was divided into three clusters which are categorized according to similar index values that are also statistically significant (Fig. 2). The number of countries grouped in each clusters were 66, 65 and 36 respectively.
The clusters which were originally grouped by the combination of CRI and EPI indices are found to be associated with similar income groups. More than half of the countries grouped in the same clusters represent certain income classification, clusters ordered by number 1, 2 and 3 could be identified as ‘Upper Middle Income, ‘Lower Income’, and ‘High Income’ respectively (Table 4).
The principle component analysis allows identifying determinant variables that best represent attributes for each clusters. Cluster 1, which represents majority of the ‘Upper Middle Income’ countries have four distinctive variables – H2O (sanitation and drinking water), UWD (unsafe drinking water), USD (unsafe sanitation) and HAD (household air pollution from solid fuels) - that determine most significant variable of this cluster. Cluster 2 is mostly comprised of countries that are Lower middle income and Low income economies. The determinant variables of this cluster are – AGR (Sustainable Nitrogen Management Index), ECS (Ecosystem Services), CRI (High Climate Risks), and GIB (GHG Intensity Trend). Lastly, only two variables contributes significantly in identifying determinant variables of Cluster 3 which are AGR and ECS (Fig. 3).
Most notably, Cluster 2 is grouped with countries that mostly fall into ‘Lower Income’ class, which comparably associate with 4 variables, namely AGR, ECS, CRI and GIB. Distinctive feature shown in Cluster 2 is its low cluster mean value of AGR variable when compared to two other clusters. If the number is in the lower ends of the range, it indicates poor agricultural practices among this group of countries. AGR indicates the intensity of nitrogen application for agricultural practices which means, the higher the intensity of nitrogen use for agricultural practices the lower sustainable agricultural practice becomes. Application of sustainable agricultural practices are of primary interests to those countries since the land and water resources becomes scarce as such ineffective methods continues. The cluster mean of CRI in cluster 2, which is -0.22, is considered as significantly below the average, which means that most of the countries are associated with higher climate-related risks.
One of the striking features that represent Cluster 3 is its cluster mean value of AGR. The value is relatively high compared to two other clusters, which means that these countries maintains and controls the nitrogen use for sustainable agricultural practices. In contrast to sustainable use of nitrogen for agricultural activities, cluster mean value for ECS, which is an aggregation of sub-indices including tree cover loss, grassland loss and wetland loss, is found to be relatively low. This indicates that even though some of the agricultural practices can be sustainable such countries needs to strengthen ecosystem services aspects (Table 5).
3.2 GCI K-means Clustering Analysis
Total of 136 countries that contain intact values for all variables in the global competitive index were selected for the analysis (Table 1). As a result, the countries were grouped into three different clusters. The number of countries in each clusters were 43, 28 and 65 respectively (Fig. 4).
These clusters are also associated with income classifications where each clusters corresponds with ‘Lower and Lower Middle income’, ‘High income’ and ‘Upper Middle income’ respectively (Table 6).
There are four determinant variables that attributes Cluster 1 namely, BUS (Business sophistication), INFRA (infrastructure), GME (goods and market efficiency), Tech.Read (Technological Readiness). The high income Cluster shows comparative advantage in overall dimensions such as LME (Labor market efficiency), MS (Market Size), HPE (health and Primary Education) and INFRA (infrastructure). Lastly, for Cluster 3 MS and HPE contributes in the attributes of the group.
Table 7 provides summary statistics for cluster means of each clusters. It is notable that determinant variables for each clusters are exclusive and diversified rather than showing the degree of deviation from cluster means in the same variable.
Cluster 1 is grouped of ‘Lower and Low income’ economies which contains negative values of these 4 variables – BUS (Business sophistication), INFRA (infrastructure), GME (goods and market efficiency), Tech.Read (Technological Readiness). This indicates that lower income economies should be sufficiently and rigorously supported for improving enabling environments for entrepreneurial activities which can significantly improve overall competitiveness of a country. The quantity and quality of local suppliers and the extent of their interaction should be appropriately supported in a way that enables industrial clusters to geographically proximate, heightens efficiency throughout innovation opportunities in processes. By the indication of negative value of INFRA, which stands at -1.11, effective modes of transport including high-quality roads, railroads and airports need to be constructed. They are conducive to economic activities should be facilitated by investment from both the public and private sectors. Technological readiness is significantly below the average, meaning that countries fall into this cluster is short of capabilities that sustain innovation activities which is largely facilitated by investment in science and technology. This will also require sufficient investment in research and development (R&D) which has constantly been challenged for lower income countries because of the absence of high-quality scientific research institutions that can generate the basic knowledge needed to build and accept new technologies.
In contrast, the majority, 65 economies among 136, of countries represent Cluster 3 are composed of ‘High Income’ or ‘Upper Middle Income’ group. The mixture of different income groups in this cluster indicates some extent of discrepancies revealed in capabilities which are market size, health and primary education. Some of resource-rich countries such as Kuwait, Qatar and Oman etc are included in this group which indicates that more inclusive labor market and a better education and training system are key to reinforce economic activities. The high-quality vocational training and academic education will be critical for these economies to create enabling environments for innovation.
3.3 Cluster Matrix
In order to validate the compatibility and reliability of the result of cluster analysis, the result was compared with existing framework; Country Partnership Strategy (CPS). Table 8 describes main attributes of cluster combinations that were identified through cluster and principal component analysis. The description for cluster combination, both GRI and EPI and GCI groupings, is summarized in Table 8. The first cluster group (1,1) is relatively lagging behind in every development aspects, and the second (1,3) shows improved indication for basic sanitation facilities and primary education. The need for third group is more specifically addressed as they require resources for sustainable agricultural practices and measures both for climate mitigation and adaptations. The main source of income for these countries is highly dependant on agricultural activities however non-farming activities that is more or less directly interlinked with industrial development also requires far-reaching support. Lastly for the fourth group, the degree to which the development assistance is necessary might vary according to the income classification, yet it urges comprehensive development support in improving measures for agriculture, climate change and market competitiveness.
Furthermore, countries in the same cluster combination group is identified in Table 9 which is matched with focus areas outlined in existing CPS of Table 8. The result of the cluster groping is compatible to the existing framework in the range of 37.5 to 54.5 per cent (Table 9). The shaded area in Table 9 indicates core cooperation sector that also reflects descriptions addressed in Table 8, and matching compatibility, presented in per cent, was derived by counting the number of sectors on CPS that includes relevant descriptions addressed in Table 8. Such compatibility is resulted from widely applicable thematic areas such as basic need for sanitation, drinking water and education are considered as conventional areas and thus difficult to be discussed in the development cooperation without removing them. However as the results indicates, some of the issues unique to partner country’s context needs more clarity. Even though some of critical issues such as disaster management and the use of renewable energy are addressed and well-outlined, there are still room for further discussion and improvement on the list of needs of resources. The climate action and dedication for innovative activities through science and technology requires far-reaching and unprecedented support and political commitments to bring about changes. Core policies should first and foremost focus on primary areas to ensure well-being of human development but it should be directed in a way that fosters the productivity of the labor force to empower the drivers of growth and employment.
4. Conclusion
This paper analyzed Korea’s Country Partnership Strategy (CPS) and demonstrated its limitations in addressing diverse development aspects that require additional attention in view of climate change and science and technology for innovation. The CPS generally outlines common challenges of priority countries, specific areas that are in need of tailored support, and thus is important since it sets out mid- to long-term milestones for development assistance plans. It provides rationale and guidance in overall ODA decision-making at a high level, from selecting recipient countries, focus of assistance areas to the volume of aids. In other words, these factors comprises critical determinants to strengthen aid effectiveness.
Korea’s successful development and transformation has reiterated throughout history of development cooperation, and in return, the Korean government has been mobilizing financial resources to provide overseas assistance by following its development trajectory. Korea’s ODA commitments - starting from enhancing primary education, basic health and sanitation to investment in major economic infrastructures such as transport and telecommunications – have been recognized by providing the most fundamental needs in conventional development context. Long history of development cooperation in these areas and existing agreement mirroring these conventional approach might explain this concentration.
However, we must recognize that problems which demand development support on the ground have become much complicated. With increasing awareness on the burdening issues of climate change and growing needs for environmental protection parallel to economic development further complicates existing problems. This indicates a particular interest to prepare CPS in the future, additionally in the areas of climate action, environmental preservation and science and technology for innovation to sustain the development support that strengthens aid effectiveness which are of mutual benefit to our own development.
This paper presented the potential for a macro-level diagnostic approach for prioritizing countries and its areas of development interests in climate, environment and innovation context with the use of aggregated country-level indicators. The result of cluster and principal component analysis was compatible at roughly 38 to 55 per cent when compared with the existing CPS. If attributes of macro level data - gaps and inconsistency - are to be complemented by survey and ground data, the methodologies proposed in this research could be applied with further improvement for overcoming some of main challenges in the process for CPS development.
Acknowledgments
This study is supported by Green Technology Center’s research project (C20231), titled Development of R3D Impact Platform for Global Green Climate Technology : Focusing on Public R & D Technology Commercialization.
References
- ADB. 2007. Country Partnership Strategy Guidelines.
-
Cae-One, K., Kim, C.S. and Lee, E., 2014. Improving Aid Effectiveness Through Active Implementation of CPS in Bolivia). KIEP No. KIEP Research Paper No. Policy Analysis, pp.14-47.
[https://doi.org/10.2139/ssrn.2655387]
-
Cho S. 2013. A Study on Evaluation Plan by Country for CPS Performance Management. Journal of International Development Cooperation, 8(4), pp.241-258.
[https://doi.org/10.34225/jidc.2013.4.241]
- Choi, Y.C., and Kim, H.S. 2020. Analysis of CPS (Country Partnership Strategy) Conformity to Vietnam of Korean ODA Projects. International Journal of Advanced Science and Technology, 29(7), 2672-2685. Retrieved from http://sersc.org/journals/index.php/IJAST/article/view/18053
- Eckstein, D., Winges, M., Künzel, V. and Schäfer, L., 2019. Global Climate Risk Index 2020: Who Suffers Most from Extreme Weather Events? Wether-Related Loss Events in 2018 and 1999 to 2018. Germanwatch Nord-Süd Initiative eV.
-
Kang, I.S., Jang, J.Y. and Thein, D.C.C., 2015. A Study on the Cooperation Strategy in Establishing the CPS with Myanmar. KIEP Research Paper No. Policy Analysis-15-44.
[https://doi.org/10.2139/ssrn.2783197]
-
Lee S. 2017. Mid-term (2017-2020) budget research and policy implications for KOICA national cooperation projects based on the 2nd National Cooperation Strategy (CPS). Journal of International Development Cooperation, 12(4), pp.159-196.
[https://doi.org/10.34225/jidc.2017.4.159]
- MacQueen, J., 1967, June. Some methods for classification and analysis of multivariate observations. In Proceedings of the fifth Berkeley symposium on mathematical statistics and probability (Vol. 1, No. 14, pp. 281-297).
-
Marx, A. and Soares, J. 2013. South Korea’s transition from recipient to DAC donor: Assessing Korea’s development cooperation policy. International Development Policy| Revue internationale de politique de développement, 4(4.2), pp.107-142.
[https://doi.org/10.4000/poldev.1535]
- ODA Korea. 2018. Korea and ODA, Beautiful Sharing Wonderful Growing. ODA Korea website http://www.odakorea.go.kr
- OECD. 2017. OECD Economic Outlook, Volume 2017, Issue 1, Korea Country Note, OECD Publishing, Paris. www.oecd.org/eco/outlook/economic-forecast-summary-koreaoecd-economic-outlook-june-2017.pdf, .
- OECD. 2017. OECD DAC Peer Review 2017, Memorandum of Korea. www.oecd.org/eco/outlook/economic-forecast-summary-koreaoecd-economic-outlook-june-2017.pdf, .
- OECD. 2018. OECD Development Co-operation Peer Reviews: Korea 2018, OECD Publishing, Paris.
-
Park, B., Lee, H. and Koo, J.W., 2013. An Analysis on the Criteria and Methodology of Priority Recipient Selection for Country Partnership Strategy). KIEP Research Paper No. Policy Analysis-13-35.
[https://doi.org/10.2139/ssrn.2447537]
-
Popescu, G.H., Sima, V., Nica, E. and Gheorghe, I.G., 2017. Measuring sustainable competitiveness in contemporary economies—Insights from European economy. Sustainability, 9(7), p.1230.
[https://doi.org/10.3390/su9071230]
- Schwab, K., 2018. November. The global competitiveness report 2018. In World Economic Forum.
-
Sima, V. and Gheorghe, I.G., 2017. A multicriterial analysis of national competitiveness: Evidences for a resilient economy. Industrija, 45(2), pp.45-64.
[https://doi.org/10.5937/industrija45-14121]
- Wendling ZA, Emerson JW, de Sherbinin A, Esty DC et al. 2020. 2020 Environmental Performance Index. New Haven, CT: Yale Center for Environmental Law & Policy. epi.yale.edu