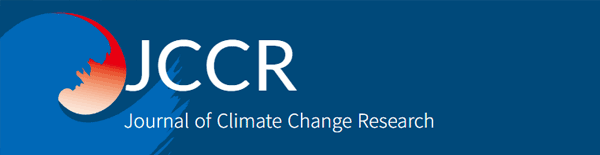
기후변화에 따른 멸종위기 야생생물 2급 백부자의 잠재서식처 변화와 파편화 예측
Abstract
Climate change influences various aspects of life on earth including distribution shifts, phenology, and interaction with other organisms. Endangered species respond more sensitively to changes within their limited distribution range. In this study, we analyzed and modeled the current distribution habitat of Aconitum coreanum, which is classified as an endangered species level 2, and further projected potential distribution changes and habitat fragmentation under climate change scenarios (Shared Socio-economic Pathway, SSP). For species distribution modeling (SDM), current habitat coordinates were collected from the national ecosystem survey and the national survey on the distribution of endangered species, and environmental data were obtained from Worldclim. An ensemble model was used as a SDM for potential habitat analysis, and landscape metrics were used for fragmentation analysis. The potential habitat area was about 15,216 km2 and the distribution included the Yeongseo region of Gangwon-do and some areas of Chungcheongbuk-do in the current climate. Modeling revealed potential habitat decreases of at least 80% and complete disappearance in SSP3-7.0. The number of patches, mean patch area, total core area, and patch cohesion index showed a decreasing trend compared to the current climate. Our results provide fundamental information for understanding the potential effects of climate change on the distribution of Aconitum coreanum. Adding ecological processes such as dispersal and population dynamics to such an analysis will make it possible to accurately predict the future impacts of climate change on the distribution of endangered species.
Keywords:
Aconitum Coreanum, Endangered Species, Climate Change, Habitat Fragmentation1. 서론
20세기 이후 기후변화는 2001 ~ 2020년의 지구 표면온도를 1850 ~ 1900년 대비 1.09°C 상승시켰다(IPCC, 2021). 기후변화는 지구 표면 온도만 상승시키는 것에 그치지 않고 전 지구적 열적 에너지의 평행 상태를 급격하게 변화시켰으며, 이로 인해 기존 상태에 적응하고 있던 동식물들에게 서식처 상실, 생존을 위한 먹이자원 감소, 질병의 증가 등 많은 부정적 영향을 주었다(Caldwell et al., 1998; Barnes et al., 2022). 한국이 포함되어 있는 중위도 지역에서 식물의 생장기는 10년마다 2일 상승하였고 양서파충류의 질병 증가가 보고된 바 있다(IPCC, 2021; Park, 2012). 전세계적 기후변화 추세를 연구하기 위한 다국적 연구자 그룹인 IPCC (Intergovernmental Panel on Climate Change)에서는 1988년 1차 종합평가보고서를 시작으로 2021년 6차 보고서를 발간하였으며 최근 6차 보고서에서는 21세기에 발생한 기후변화가 인간의 무분별한 활동에 의해 발생한 것으로 규정하였다(IPCC, 2021). 이러한 기후변화 추세가 계속 이어진다면 21세기 말에는 시나리오에 따라 온도가 1.0 ~ 5.7°C까지 상승하고 강수량 등 물순환 불균형이 더욱 강화할 것으로 예측된다(IPCC, 2021).
기후변화와 함께 인간에 의한 활동 증가는 생물종다양성에도 영향을 미쳤다. 특히 21세기 이후에 지속적으로 증가하고 있는 생물종의 멸종을 경고하기 위해 최근의 빨라진 생물종 멸종속도를 제6차 대멸종 또는 인류세 멸종이라 지칭하기도 한다(Ceballos et al., 2010). 이러한 멸종 추세를 늦추고 생물종 보전을 위해서 IUCN (International Union for Conservation of Nature)에서는 사라져가는 생물종에 관한 정보와 보전을 위한 자료를 적색목록(IUCN Redlist)으로 만들어서 제공하고 있다(Rodrigues et al., 2006). 전세계 redlist에 들어있는 종의 숫자는 2022년 기준으로 CR (Critically Endangered), EN (Endangered), VU (Vulnerable)에 40,084종으로 2019년의 28,200종 보다 42% 증가하였다(IUCN 2019; IUCN 2021). 국내에서는 국내 서식처를 가지는 생물종에 관한 보호와 복원을 위하여 환경부에서는 1989년부터 특정야생동식물 203종을 지정하였으며 2012년부터 멸종위기 야생생물 명칭을 개정하여 관리하고 있다. 2023년 멸종위기 야생생물 3차 개정 기준으로 282종이 지정되어 1989년에 비해 39% 증가한 추세를 보였다(NIE, 2022).
멸종위기 야생생물에서 육상식물은 92종(1급 13종, 2급 79종)이 있으며 백부자는 미나리아재과 초오속의 여러해살이풀로 2005년부터 멸종위기 야생생물 2급으로 지정되어 보호받고 있다(NIE, 2022). 백부자는 과거 약용식물로 무분별한 채취로 인하여 야생개체군이 줄어들게 되었고 그에 따라 멸종위기 야생생물로 지정되었으며 IUCN 적색목록에는 관심대상(LC), 국가적색목록에서는 취약(VU)로 평가받고 있다(NIBR, 2018a). 한국과 중국 등 동아시아에서 약용식물로 사용되기 때문에 선행연구의 대부분이 추출물에 대한 향균효과 및 독성에 관한 것이다(Hwang et al., 2020; Xu et al., 2020). 일부 연구에서 백부자의 화분매개곤충 등 생태에 관한 것들이 진행되었지만 멸종위기 야생생물로써 기후변화가 백부자에 미치는 영향을 모형을 통해서 확인한 연구는 현재까지 수행되지 않았다(Han et al., 2013).
종분포모형은 Hutchinson (1957)의 생태적 지위 이론에 기반한 모형으로 생물의 출현은 다양한 환경 요인들에 의해 결정된다는 가정하에서 시작한다(Guisan and Zimmermann, 2000). 다양한 환경 요인들은 모형을 적용하는 스케일과 자료의 여건에 따라 연구자에 판단에 의해 결정된다(Austin and Van Niel, 2011). 환경요인과 종의 관계를 통계적 관계로 확인하는 모형을 상관모형(Correlative model)이라고 하며 환경기작을 통해서 종에 적합한 환경을 밝혀내는 모형을 기작모형(Mechanistic model)이라 한다(Latimer et al., 2006; Kearney and Porter, 2009). 상관모형은 적은 데이터를 활용하여서 모형의 구현이 가능하기 때문에 기후변화와 관련된 연구(Kearney et al., 2010), 보호지역 설정과 관련된 연구(Pawar et al., 2007; Fuller et al., 2008; Kremen et al., 2008), 멸종위기 종의 새로운 개체군을 찾는 연구들(Guisan et al. 2006; de Siqueira et al., 2009)에서 자주 활용된다. 멸종위기종과 같이 생태적 자료 및 서식에 관한 선행연구가 많지 않은 경우에 상관모형을 활용하여 적합한 잠재서식처를 찾을 수 있다(Freeman et al. 2019; Zhao et al. 2020).
서식처 연결성은 생물종이 서식할 수 있는 다양한 패치들이 물리적, 기능적으로 연결되어 있는 상태를 의미한다(Schumaker, 1996; Fall et al., 2007). 특히 물리적 연결은 생물의 확산 능력에 따라 신규 서식지로 이동을 가능하게 할 수 있다(Diniz et al., 2020). 이러한 서식처의 연결성이 감소한다면 패치들 간의 파편화가 증가하고 기존 생물들의 고립은 증가하고 다양한 외부 요인에 의해 생물종이 생존의 위협을 받을 가능성은 증가한다(Uroy et al., 2019). 그렇기 때문에 경관의 연결성을 평가하는 것은 생물에 대한 서식처 상태를 확인할 수 있는 좋은 지표로 활용된다(Liu et al., 2014). 선행 연구들에서는 파편화 분석과 종분포모형, 개체군 모형을 함께 결합모형으로 개발하여 서식처의 상태와 다양한 외부요인으로부터 서식처 변화를 평가하는데 활용되고 있다(Bishop-Taylor et al., 2018; Carvajal et al., 2018; McCluskey et al., 2022).
본 연구는 멸종위기 야생생물 2급으로 지정되어서 보호받고 있는 백부자를 대상으로 기후 자료를 이용한 종분포모형을 활용하여 서식처 분포를 예측하고 기존에 알려진 서식처와의 적합도를 추정하였다. 또한 기후변화에 따라 미래 서식처 변화 양상을 시뮬레이션 하였다. 미래 기후는 IPCC 6차 보고서에서 제시된 공통사회경제경로(SSP, Shared Socioeconomic Pathways) 시나리오에 따라 예측하고, 이를 바탕으로 백부자의 미래 서식처가 북쪽으로 이동할 것인지, 서식처 면적은 줄어들 것인지, 파편화 정도는 심해질 것인지에 관한 질문에 답을 찾고자 하였다. 이를 통해 멸종위기종인 백부자에 관한 서식처 보전 및 복원에 관한 기초자료로 활용될 수 있을 것이다.
2. 연구재료 및 방법
2.1. 연구대상 및 연구지역
백부자(Aconitum coreanum)는 미나리아재비과 여러해살이풀로 국내에서 강원도, 충청북도, 경기도 일대로 높지 않은 산지 중산간에서 나타나며 전세계적으로 중국 동북부 지역, 러시아 극동 지역과 몽골에서 서식하는 것으로 알려져 있다(Nyirimigabo et al., 2015; Kim et al., 2016). 개화시기는 7월이며 열매가 맺는 시기는 8월로 알려져 있다(NIE, 2022). 덩이뿌리가 발달하며 높이는 최대 1m 중반까지 이르고 짧은 잎자루를 가진다(NIBR, 2018a). 열매는 동골(follicle)의 형태를 보이며 길이는 1 ~ 2 cm 정도이고 씨는 타원형으로 좁은 날개를 가진다(NIBR, 2018a). 백부자는 백부, 관백부, 노랑동쩌귀의 이명을 가지는 독성을 품은 약재로 특정 알칼로이드를 가지고 있어 뇌졸증, 간질, 구안와사 등의 약재로 한국과 중국에서 활용되고 있다(Joe, 2019). 그렇기 때문에 백부자에 관한 선행연구로는 추출물이 동물의 심장에 미치는 작용을 관찰한 연구들과 항균 및 항산화 효과 등의 생리적 효능에 관한 연구가 주로 이루어졌으며 일부 화분매개곤충을 파악하고자 한 연구들과 식물상에 관한 연구가 대부분을 차지한다(Yoon et al., 2005; Han et al., 2013; Kang et al., 2015).
연구대상 지역인 대한민국은 동고서저 형태의 지형적 특징을 보이며 지리적으로 중위도 온대성 기후대에 위치하고 있어 봄과 가을에는 이동성 저기압으로 맑고 건조하며 여름에는 고온 다습한 기후를 보인다(KMA, 2012; NGII, 2019). 또한 겨울에는 대륙성 고기압에 의해 춥고 건조한 날씨를 보인다. 이러한 특징과 함께 연평균기온은 7-15°C에 이르며 지역적 차이가 존재한다. 또한 연 강수량은 1306.3 mm에 이르지만 여름철 강수량이 연 강수량의 50% 중반을 차지하는 등의 계절적 차이를 나타낸다(KMA, 2022). 2010년대 말 토지피복을 기준으로 살펴보면 전 국토의 68.7%가 산림이며 농경지는 19.7%, 시가화건조지역은 5.6%를 이루고 있다(NGII, 2020). 농경지와 시가화·건조지역은 동고서저의 지형적 특징으로 대부분이 서부와 남부 지역에 위치한다. 1980년대 말부터 현재까지 시가화·건조지역이 서울을 비롯한 대도시권 중심으로 확장하였으며 이로 인해 농업지, 산림지역 등 생태계를 이루는 많은 토지피복들이 사라졌다(NGII, 2020).
2.2. 분포자료 및 환경변수들
종분포모형은 허친슨의 기본 지위에 기반을 한 모형으로 종에 적합한 서식처는 특정 환경의 총합으로 이루어진다고 가정한다(Hutchinson, 1957; Guisan and Zimmermann, 2000). 종의 출현과 특정 환경간의 관계를 통계적 기법을 통해서 도출하는 모형을 상관모형(Correlative model)이라 하며 회귀방식을 사용한 일반화 선형 모형(Generalized Linear Model, GLM) (Guisan et al., 1999), 비선형적 관계를 표현한 일반화 가법 모형(Generalized Additive Model, GAM) (Ikegami and Jenkins, 2018), 통계기법을 통해서 많은 데이터세트를 생성하고 이를 활용하여 모형의 정확도를 높이는 기계학습모형(Machine learning model) (Evans et al., 2011; Merow et al., 2013) 이 활용되며 이들 모형의 결과를 합하여 불확실성을 최소화하는 앙상블 모형도 사용된다. 종분포모형의 개발을 위해서 연구대상종인 백부자의 분포 데이터가 필요하며 이를 위해 멸종위기야생생물 전국분포조사(2014 ~ 2019)와 제2 ~ 4차 전국자연환경조사(1997 ~ 2018)에서 확인된 백부자의 135개 분포지점을 수집하였다(NIBR, 2021, 2018b, 2015, 2012; NIE, 2020; NIER, 2013, 2006). 또한 상관모형을 구현함에 있어서 통계적 자기상관을 최소화하기 위하여 환경변수의 한 격자에서 중복되어 나타나는 지점을 제거하여 총 82개의 출현지점을 사용하여 모형을 구현하였으며 비출현자료는 총 500개를 무작위 추출하여 사용하였다.
모형의 변수로 활용된 생물기후변수(Bioclimatic variables, Bioclim)는 생물의 성장과 생존에 영향을 미치는 특정 시기에 따라 기온과 강수를 활용하여 만들어졌다(O'Donnell and Ignizio, 2012). 총 19개의 변수가 있으며 각 변수들 중에서 연구대상종에 따라 특정 변수를 선택하거나 상관관계가 적은 변수(0.75 이하)를 선택해야 하며 본 연구에서는 상관관계가 적은 bio01, bio02, bio03, bio12, bio13, bio14를 선택하였다(Table 1). 변수들은 Worldclim에서 제공하는 30 arc-Seconds(ca 1 km2) 해상도의 공간자료를 사용하였다(Fick and Hijmans, 2017). 백부자의 미래 기후변화 예측을 위해서 IPCC 6차 종합보고서에서 제안된 결합 모델 상호비교 프로젝트(Coupled Model Intercomparison Project Phase 6, CMIP6) 기준에 맞는 6개 일반 순환 모형(General Circulation Model, GCM)들과 공통사회경제경로(Shared Socioeconomic Pathway, SSP) 시나리오를 활용하였다(Table 2) (Riahi et al., 2017). 미래 예측은 2050년대(2041년 ~ 2060년 평균)와 2100년대(2081년 ~ 2100년 평균) 기후예측 자료를 사용하여 백부자의 잠재서식처 면적 변화를 추정하였다.
2.3. 종분포모형과 파편화 분석
종분포모형에서 개별 알고리즘은 각기 장단점이 존재하며 앙상블모형은 각기 다른 상관모형을 통해서 나온 결과를 통합하여 사용하는 모형으로 각각의 모형에서 오는 장단점을 평균화 할 수 있다. 본 연구에서는 9개의 개별 종분포모형의 알고리즘((Generalized Linear Models (GLM), Generalized Boosted Models (GBM), Generalized Additive Models (GAM), Classification Tree Analysis (CTA), Artificial Neural Networks (ANN), Surface Range Envelope (SRE), Flexible Discriminant Analysis (FDA), Multivariate Adaptive Regression Splines (MARS), Random Forest (RF))을 사용하여 개별 모형을 구현하고 각 결과를 활용하여 앙상블 모형을 개발하였다(Thuiller et al., 2009). 모형의 검증을 위한 데이터 분할을 7:3으로 하여 모형의 개발과 검증에 활용하였고 모형의 정확도는 AUC (Area under the ROC curve)와 TSS (True Skill Statistic)를 통해서 평가하였다(Allouche et al., 2006; Jimenez-Valverde, 2012). AUC와 TSS는 0에서 1까지의 범위를 가지며 AUC는 0.8 이상은 정확도가 높음 이상이며 TSS는 0.6 ~ 0.7은 높음으로 평가받는다(Park et al., 2016; Chung et al., 2020). 앙상블모형은 모형의 정확도를 나타내는 TSS (True Skill Statistic)가 0.7 이상인 개별모형들에 TSS에 따라 가중치를 주어서 구현하였다. 모형의 임계점은 TSS가 최대값이 되는 지점으로 지정하였으며 개별모형과 앙상블모형 개발은 R 패키지인 biomod2를 활용하였다(Thuiller et al., 2009).
파편화 분석은 기후변화가 잠재서식처 분포와 함께 파편화에 미치는 영향을 확인할 수 있다. 본 연구에서는 현재와 미래 잠재서식처를 활용하여 잠재서식처의 이동과 파편화를 살펴보았다. 현재와 미래 잠재서식처의 이동을 살펴보기 위해 잠재서식처 중심좌표와 평균고도를 산출하였다. 또한 파편화를 확인하기 위해 잠재서식처의 패치의 수, 패치의 평균면적, 핵심지역 총면적, 응집지수를 계산하였다(McGarigal, 1995). 패치의 수, 패치의 평균면적과 핵심지역의 총면적은 잠재서식처의 파편화 진행 수준을 확인할 수 있으며 응집지수는 잠재서식처들간의 연결성을 보여주는 지수로써 높을수록 잠재서식처들이 연결되어 있음을 보여준다. 잠재서식처의 이동과 파편화 분석은 R패키지인 SDMTools를 활용하였다(Jeremy et al., 2014).
3. 연구결과
3.1. 모형의 정확도와 변수의 중요도
백부자의 잠재서식처 분석을 위해 9개 알고리즘과 20개 데이터세트를 사용하여 총 180개의 개별 종분포모형을 구현하였다. 이렇게 구현된 종분포모형은 20개 데이터세트에서 검증 데이터를 사용하여 AUC와 TSS를 산출하여서 모형의 정확도를 평가하였다. 이를 통해서 9개 개별 알고리즘별 평균 AUC와 표준편차, 평균 TSS와 표준편차를 산출하여 AUC와 TSS의 Boxplot을 생성하였다(Fig. 1). AUC를 기준으로 살펴보면 RF와 GBM이 상대적으로 높은 수치를 보였으며 CTA, SRE이 낮은 정확도가 나왔다. TSS는 AUC보다 낮은 정확도를 보이지만 각 알고리즘별 정확도의 추세는 비슷하게 확인되었다. 개별 알고리즘에 따라 비슷한 표준편차를 보이지만 AUC에서 CTA의 경우 상대적으로 다른 알고리즘에 비해 높은 표준편차를 보였다.
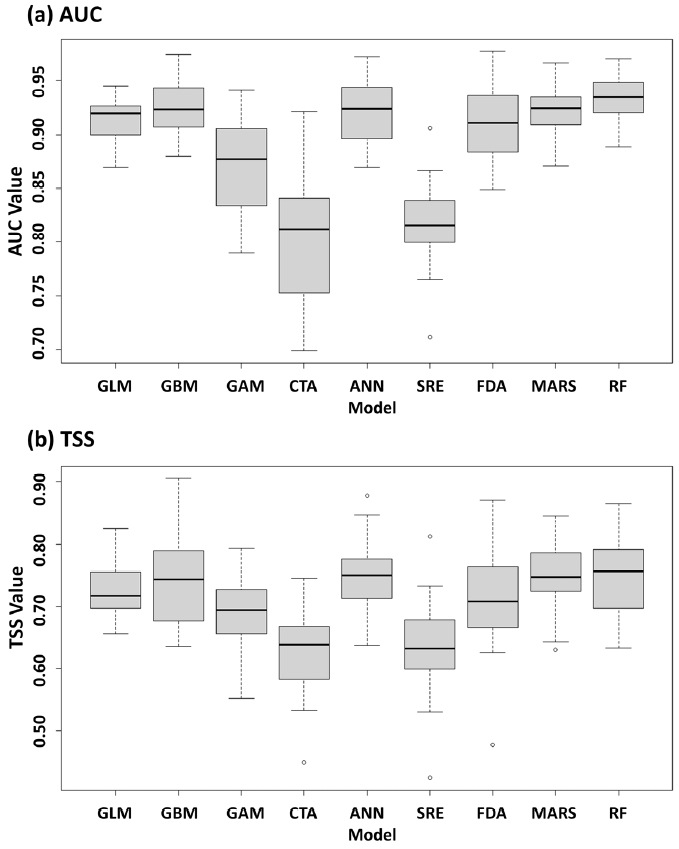
Boxplot of accuracy of the different algorithms (area under the curve, AUC) (a) and (true skill statistics TSS) (b). (GLM: generalized linear models, GBM: generalized boosted models, GAM: generalized additive models, CTA: classification tree analysis, ANN: artificial neural networks, SRE: surface range envelope, FDA: flexible discriminant analysis, MARS: multivariate adaptive regression splines, RF: random forest)
앙상블 모형은 180개 개별 종분포모형들에서 TSS가 0.7 이상인 모형들을 TSS에 따라 가중치를 주어서 구현하였다. 180개 개별 종분포모형에서 TSS가 0.7 이상인 103개 모형들이 앙상블모형을 구현하는데 활용되었다(Table 3). 앙상블 모형의 정확도는 TSS가 0.891, AUC가 0.984로 개별 종분포모형들 보다 높게 나타났다. 앙상블 모형을 구현하는데 사용된 103개 모형들의 변수 중요도를 산출하여서 계산한 결과, 연평균기온이 높은 중요도를 보였으며 연 강수량과 건기의 강수량이 상대적으로 낮은 중요도를 나타났다(Fig. 2).

Accuracy for ensemble model of Aconitum coreanum and the number of SDMs for ensemble model (TSS: True Skill Statistics, AUC: Area Under the Curve)
3.2. 기후변화에 따른 잠재서식처 변화 예측
현재 기후에서 백부자의 잠재서식처를 예측한 결과, 잠재서식처 면적은 약 15,216 km2로 예측되었다(Table 4). 기후 변화에 따라 백부자의 잠재서식처 변화를 예측하기 위해 CMIP6에 해당하는 6개 GCM의 미래 기후 예측 자료를 사용하여 백부자의 미래 잠재서식처 변화를 분석하였다. 기후변화 시나리오는 SSP-2.6, SSP2-4.5, SSP3-7.0를 활용하여 2050년대(2041 ~ 2060년 평균)와 2100년대(2081 ~ 2100년)를 추정하였다. 6개 GCM의 미래 잠재서식처 면적 결과를 평균하여서 미래 면적을 계산한 결과, SSP1-2.6 시나리오에서는 현재 잠재서식처 면적 대비 평균 77.5% 감소하며 SSP2-4.5에서는 85.5%, SSP3-7.0에서는 90.5%가 줄어들었다(Table 4). 미래 예측 시기에 따라 비교를 하면 현재와 비교해서 2050년대에는 79.7%, 2100년대에는 89.3%가 사라지는 것으로 나타났다.

Current potential habitat area, mean area of future potential habitat and ratio of future potential habitat to current habitat of Aconitum coreanum
현재 기후에서 강원도 영서지역과 충청북도 일부 지역에서 잠재서식처가 주로 나타났으며 강원도 정선군, 평창군, 영월군 일대에서 잠재서식처의 적합도가 중간에서 높음( > 0.584)으로 확인되었다(Fig. 3). 현재 기후에서 잠재서식처 분포 형태를 살펴보면 강원도에서 경상북도를 지나는 백두대간의 서쪽과 북쪽 방향으로 잠재서식처가 예측되었다. 기후변화 시나리오에 따라 잠재서식처 분포 변화를 예측한 결과, 강원도 영서지역과 충청북도 일부 지역에 넓게 분포하고 있던 잠재서식처의 상당수가 사라지고 잠재서식처 적합도 또한 낮아지는 것(0.435-0.584)으로 분석되었다(Fig. 3). SSP1-2.6 시나리오에서는 현재 기후에서 DMZ부터 충청북도까지 연결되어 있던 잠재서식처가 분리되었으며 충청북도 일대에 있던 잠재서식처 또한 사라졌다(Fig. 4-(a)). 또한 2050년에 강원도 영서지역에 분포하고 있던 잠재서식처가 2100년대에는 잠재서식처의 주변부가 사라지는 것으로 예측되었다(Fig. 4-(b)). SSP2-4.5와 SSP3-7.0 시나리오에서는 2050년대 잠재서식지의 분포 형태가 SSP1-2.6 시나리오와 유사하게 나타났지만 2100년대에 이르면 잠재서식처의 대부분 지역이 사라졌다(Fig. 4-(c), (d), (e), (f)).
3.3. 기후변화에 따른 잠재서식처 이동 및 파편화 예측
기후변화가 백부자의 잠재서식처 이동과 파편화에 미치는 영향을 살펴보기 위해 잠재서식처 중심점과 파편화 분석을 수행하였다. 중심점의 이동은 잠재서식처 중심 좌표와 평균 고도를 통해서 확인하였다. 현재 기후의 잠재서식처 중심좌표에서 SSP1-2.6시나리오의 이동거리는 2050년에 49.2 km, 2100년에 8.1 km로 나타났으며 SSP2-4.5시나리오에서는 2050년에 49.4 km, 2100년에 42.4 km로 계산되었다. SSP3-7.0 시나리오는 2050년에 53 km, 2100년에 57.7km 이동하는 것으로 예측되었다. SSP시나리오에 따른 평균 이동거리를 살펴본 결과 SSP1-2.6, SSP2-4.5, SSP3-7.0 순으로 증가하는 것으로 나타났다. 현재 기후에서 잠재서식처의 평균 고도는 436 m로 SSP1-2.6 시나리오의 2050년에는 810 m, 2100년에는 841 m로 상승하며 SSP2-4.5 시나리오에서는 2050년에 839 m, 2100년에 1014 m로 증가하였다. SSP3-7.0 시나리오에서 평균 고도는 2050년에 882 m, 2100년에 1051 m로 분석되었다. SSP시나리오에 따라 평균 고도 상승은 SSP1-2.6, SSP2-4.5, SSP3-7.0의 순서로 나타났다.
기후변화에 따른 백부자의 잠재서식처 파편화 영향은 패치의 수, 패치들의 평균 면적, 핵심지역의 총면적과 응집지수를 통해서 평가하였다(Table 5). 현재 기후에서 패치의 수, 패치들의 평균 면적, 핵심지역의 총 면적은 각각 276개, 55.1 km2, 7,873 km2로 분석되었다. 현재에 비해 SSP 시나리오들에서 패치의 수, 패치들의 평균 면적, 핵심지역의 총면적, 응집지수 등 모든 수치가 감소하였다. 감소폭은 SSP3-7.0, SSP2-4.5, SSP1-2.6 시나리오 순으로 크게 나타났다. 특히 SSP3-7.0 시나리오의 2100년대에는 현재와 비교해서 패치의수는 94%, 패치들의 평균 면적은 91%, 핵심지역의 총면적은 99% 감소하였다. 응집지수는 현재와 비교하여 64% 축소하였다.
4. 고찰
기후는 지역에 장기간에 걸쳐서 나타난 대기의 합으로 식물은 기후에 의해 분포가 결정된다(Merriam, 1898; Kelly and Goulden, 2008). 기후변화는 이러한 장기간에 걸친 환경이 변화하는 것을 의미하며 이는 이동성이 떨어지는 식물은 기후변화에 더욱 큰 영향을 받을 수 있다(Corlett and Westcott, 2013). 난대성 수종은 기후변화에 따라 서식할 수 있는 공간이 확대될 것으로 예측되며 이와 반대로 한대성 수종은 기후변화에 따라 서식 공간이 수평적, 수직적으로 감소할 것으로 예측된다(Koo et al., 2015; Koo et al., 2018). 멸종위기종과 같이 제한적인 기후 지위를 가는 종은 기후변화에 취약하며 이동성이 제한적인 식물은 더욱 취약할 수 있다(Cai et al., 2022). 본 연구에서는 멸종위기 야생생물인 백부자를 대상으로 기후변화에 따라 잠재서식처의 면적과 중심점 이동을 통해서 기후변화 영향을 살펴보았다. 백부자의 잠재서식처는 현재와 비교해서 84% 감소하는 것으로 나타났으며 1년에 평균 0.96 km 이동하는 것으로 분석되었다. 이는 분포가 제한적으로 나타나는 멸종위기 야생생물에게 기후변화가 주요한 위협요인이 될 수 있음을 보여줬다.
서식처 파편화는 서식처의 주변지역을 확대하기 때문에 서식 환경을 약화시킨다(Laurance et al., 2006; Wilkinson et al., 2018). 그렇기 때문에 경관 생태학적 관점에서 큰 서식지가 서로 연결되어 있는 형태가 좋은 서식처의 형태로 간주된다(Bennett et al., 2006). 본 연구에서는 기후변화가 백부자의 잠재서식처 파편화를 강화시키는 것으로 가정하고 파편화 분석을 수행하였다. 파편화 정도를 살펴볼 수 있는 패치의 수, 패치들의 평균 면적, 핵심지역의 총 면적과 융합지수를 분석한 결과, 현재 기후에 비해 미래 기후에서 모든 수치가 감소하였다. 기후변화가 백부자의 잠재서식처 파편화에 부정적 영향을 주었으며 이는 기후변화에 의한 서식처 파편화가 백부자의 위협요인이 될 수 있음을 알려준다.
기후변화는 기온 상승과 더불어 강수 패턴을 변화시켜 이에 적응하고 있던 다양한 생물들의 생존에 심각한 악영향을 미친다(Gustafson et al., 2017; Dai et al., 2018; Konapala et al., 2020). 특히 이러한 기후변화 추세가 계속 이어져서 지구 평균 온도 상승이 1.5°C를 초과한다면 육상, 해양 생물다양성의 감소와 극한 기상으로 인류는 생존의 위협에 직면하게 된다(IPCC, 2022). 본 연구에서 적용한 기후변화 시나리오인 SSP1-2.6은 지구 평균 온도 상승 1.5°C에 제일 유사한 결과를 보여주는 것으로 해당 시나리오에서 백부자의 잠재서식처 면적 감소는 다른 시나리오에 비해 적은 것으로 예측되었다. 멸종위기종은 다른 종들에 비해 특정 기후 지위를 가지며 적은 개체군에 인해 유전적 다양성이 낮아 기후변화와 같은 환경 적응력이 떨어지기 때문에 멸종위기에 쉽게 노출될 수 있다(Frankham, 2005; Li et al., 2012). 그렇기 때문에 우리는 멸종위기에 처한 생물의 보호를 위해 기후변화를 최소화 할 수 있는 생활, 산업적 노력을 이행해야한다. 또한 멸종위기종의 서식처에 관한 기초 생태연구를 통해 적절한 서식환경 분석, 증식을 수행하고 서식지 이전과 복원 등에 관한 정책적 노력을 기울여야 한다.
본 연구에서 사용한 상관모형은 식물의 성장 및 생존 등의 생리적 관계를 고려하지 못한다는 단점이 존재한다(Austin, 2007). 또한 기존 RCP 시나리오에서 사회 발전을 가정한 SSP 시나리오를 사용하였지만 토지이용 변화와 같은 인간에 의한 영향을 고려하지 못하였다(Riahi et al., 2017). 종에 관한 자료가 충분하지 못한 멸종위기 야생생물과 달리 종에 관한 연구가 충분한 기후변화 지표종의 경우, 개별종의 산포, 개체군 변동과 같은 생태적 과정을 고려한 기후변화 연구가 수행되었다(Park et al., 2019; Machado-Stredel et al., 2021; Koo and Park, 2022). 본 연구는 이러한 특성을 고려한 기후변화 취약성 연구들 보다는 현실성이 떨어진다. 또한 대한민국 정도의 공간적 스케일을 가지는 연구로써 분포지점이 적고 제한적이기 때문에 미소서식지에 대한 중요성이 있는 백부자 연구에는 한계점이 존재한다.
이러한 단점을 극복하기 위해서는 식물의 생물학적 관계를 가지는 변수를 연구에서 고려하는 등으로 보완할 수 있다(Penteriani et al., 2019). 또한 산포와 토지이용 예측 등을 고려한 다양한 모형을 같이 사용하는 결합모형의 개발이 필요하다(Koo and Park, 2022). 미소서식지가 다른 지표종보다 중요한 멸종위기종이기 때문에 공간적 스케일을 2개로 가져감으로써 필요에 따라 대상지역에 관한 미소서식처 분석을 통해 멸종위기종에 관한 기후변화 영향 및 취약성을 평가 할 수 있게 되어야 한다(Hattab et al., 2014). 미소서식처 단위에서 연구가 진행될 경우에 개체군 단위에서 서식처를 식별하고 평가하는 모형을 구현해야 하며 이를 위해서는 개체군 기반의 모니터링 기법 및 수행이 선행되어야 한다(Koo and Park, 2021).
멸종위기종은 분포가 제한적이고 적은 개체군을 가지고 있기 때문에 기후변화와 같은 환경변화에 더욱 취약한 것으로 알려져 있다(Imperio et al., 2013; Keith et al., 2014). 그렇지만 자료 접근의 한계와 연구가 충분이 이루어지지 못했기 때문에 기후변화에 관한 연구는 거의 이루어지지 못했다. 하지만 백부자는 다른 멸종위기종들보다 분포 데이터가 풍부하며 이를 통해서 공간모형을 활용한 기후변화 영향을 살펴볼 수 있었다. 본 연구를 통해서 백부자는 기후변화에 따라 잠재서식처 면적이 급격하게 줄어들고 그에 따라 생존에 큰 위협을 받는 것으로 예측되었다. 본 연구 결과는 미소서식지 단위에서 개체군 기반의 백부자의 기후변화 취약성 평가가 이루어질 수 있는 토대를 제공하였다. 또한 공간 모형 기반의 기후변화 영향평가는 기존에 이루어지지 않은 멸종위기종에 대한 기후변화 영향을 판단하고 대응 정책 수립을 위한 기초자료로 활용될 수 있다.
5. 결론
본 연구에서는 멸종위기 야생생물 2급 백부자를 대상으로 기후변화에 따른 서식처와 파편화를 예측하였다. 현재 기후에서 백부자의 잠재서식처는 강원도와 충청북도 지역으로 예측되었다. 미래 기후에서 잠재서식처가 축소하는 것으로 예측되었다. 서식처 적합성도 현재기후에서 높게 나타났단 강원도 태백, 영월, 충북 단양 등이 낮게 예측되었으며 서식처 적합성이 낮았던 지역의 대부분이 사라지는 것으로 확인되었다. 파편화 분석에서 현재 기후의 패치의 숫자와 평균 면적 모두 미래 기후에서 감소하는 것으로 분석되었다. 백부자에 적합한 서식처는 매우 줄어들고 파편화 정도도 악화되는 것으로 예측되었다. 본 연구는 백부자가 공간모형 기반의 기후변화 영향평가를 통해서 살펴본 결과, 기후변화에 취약한 것으로 나타났다. 현재 연구보다 정확한 기후변화 평가 연구를 위해서는 현재 연구 스케일보다 작은 스케일에서 미소서식지를 찾고 기후변화에 관한 개체군 단위에서 영향을 확인하는 연구가 필요할 것으로 보인다.
Acknowledgments
본 연구는 환경부의 재원으로 국립생태원의 지원(NIE-기반연구-2023-34)과 한국환경연구원에서 수행 중인 "기후변화에 따른 부문별 사회·경제적 피해비용 분석 연구(과제번호:2021-096)"의 지원을 받아 수행되었습니다.
References
-
Allouche O, Tsoar A, Kadmon R. 2006. Assessing the accuracy of species distribution models: prevalence, kappa and the true skill statistic (TSS). J. Appl Ecol. 43(6):1223–1232. doi:10.1111/j.1365-2664.2006.01214.x.
[https://doi.org/10.1111/j.1365-2664.2006.01214.x]
-
Austin M. 2007. Species distribution models and ecological theory: A critical assessment and some possible new approaches. Ecol Modell. 200(1):1–19.
[https://doi.org/10.1016/j.ecolmodel.2006.07.005]
-
Austin MP, Van Niel KP. 2011. Improving species distribution models for climate change studies: variable selection and scale. J. Biogeogr. 38(1):1–8.
[https://doi.org/10.1111/j.1365-2699.2010.02416.x]
-
Barnes PW, Robson TM, Neale PJ, Williamson CE, Zepp RG, Madronich S, Wilson SR, Andrady AL, Heikkilä AM, Bernhard GH, et al. 2022. Environmental effects of stratospheric ozone depletion, UV radiation, and interactions with climate change: UNEP Environmental Effects Assessment Panel, Update 2021. Photochem. Photobiol. Sci. 21(3):275–301. doi:10.1007/s43630-022-00176-5.
[https://doi.org/10.1007/s43630-022-00176-5]
-
Bennett AF, Radford JQ, Haslem A. 2006. Properties of land mosaics: Implications for nature conservation in agricultural environments. Biol. Conserv. 133(2):250–264. doi:10.1016/j.biocon.2006.06.008.
[https://doi.org/10.1016/j.biocon.2006.06.008]
-
Bishop-Taylor R, Tulbure MG, Broich M. 2018. Impact of hydroclimatic variability on regional-scale landscape connectivity across a dynamic dryland region. Ecol. Indic. 94:142–150. doi:10.1016/j.ecolind.2017.07.029.
[https://doi.org/10.1016/j.ecolind.2017.07.029]
-
Cai C, Zhang X, Zha J, Li Jie, Li Junmin. 2022. Predicting climate change impacts on the rare and endangered Horsfieldia tetratepala in China. Forests. 13(7):1051. doi:10.3390/f13071051.
[https://doi.org/10.3390/f13071051]
-
Caldwell MM, Björn LO, Bornman JF, Flint SD, Kulandaivelu G, Teramura AH, Tevini M. 1998. Effects of increased solar ultraviolet radiation on terrestrial ecosystems. J. Photochem. Photobiol. B, Biol. 46(1):40–52. doi:10.1016/S1011-1344(98)00184-5.
[https://doi.org/10.1016/S1011-1344(98)00184-5]
-
Carvajal MA, Alaniz AJ, Smith-Ramírez C, Sieving KE. 2018. Assessing habitat loss and fragmentation and their effects on population viability of forest specialist birds: Linking biogeographical and population approaches. Divers. Distrib. 24(6):820–830. doi:10.1111/ddi.12730.
[https://doi.org/10.1111/ddi.12730]
- Ceballos G, Garcia A, Ehrlich P. 2010. The sixth extinction crisis. J. Cosmol. 8:180–185.
-
Chung HI, Choi Y, Ryu J, Jeon SW. 2020. Accuracy evaluation of potential habitat distribution in Pinus thunbergii using a species distribution model: Verification of the ensemble methodology. J. Climate Change Res. 11(1):37–51. doi:10.15531/KSCCR.2020.11.1.37.
[https://doi.org/10.15531/KSCCR.2020.11.1.37]
-
Corlett RT, Westcott DA. 2013. Will plant movements keep up with climate change? Trends Ecol. Evol. 28(8):482–488. doi:10.1016/j.tree.2013.04.003.
[https://doi.org/10.1016/j.tree.2013.04.003]
-
Dai A, Zhao T, Chen J. 2018. Climate change and drought: a precipitation and evaporation perspective. Curr Clim Change Rep. 4(3):301–312. doi:10.1007/s40641-018-0101-6.
[https://doi.org/10.1007/s40641-018-0101-6]
-
Diniz MF, Cushman SA, Machado RB, De Marco Júnior P. 2020. Landscape connectivity modeling from the perspective of animal dispersal. Landscape Ecol. 35(1):41–58. doi:10.1007/s10980-019-00935-3.
[https://doi.org/10.1007/s10980-019-00935-3]
-
Evans JS, Murphy MA, Holden ZA, Cushman SA. 2011. Modeling species distribution and change using random forest.. In: Drew CA, Wiersma YF, Huettmann F, editors. Predictive Species and Habitat Modeling in Landscape Ecology: Concepts and Applications. New York, NY: Springer. p. 139–159. [accessed 2022 Sep 15]. https://doi.org/10.1007/978-1-4419-7390-0_8.
[https://doi.org/10.1007/978-1-4419-7390-0_8]
-
Fall A, Fortin M-J, Manseau M, O’Brien D. 2007. Spatial graphs: Principles and applications for habitat connectivity. Ecosystems (N. Y., Print). 10(3):448–461. doi:10.1007/s10021-007-9038-7.
[https://doi.org/10.1007/s10021-007-9038-7]
-
Fick SE, Hijmans RJ. 2017. WorldClim 2: new 1-km spatial resolution climate surfaces for global land areas. Int J. Climatol. 37(12):4302–4315. doi:10.1002/joc.5086.
[https://doi.org/10.1002/joc.5086]
-
Frankham R. 2005. Stress and adaptation in conservation genetics. J. Evol. Biol. 18(4):750–755. doi:10.1111/j.1420-9101.2005.00885.x.
[https://doi.org/10.1111/j.1420-9101.2005.00885.x]
-
Freeman B, Roehrdanz PR, Peterson AT. 2019. Modeling endangered mammal species distributions and forest connectivity across the humid Upper Guinea lowland rainforest of West Africa. Biodivers. Conserv. 28(3):671–685. doi:10.1007/s10531-018-01684-6.
[https://doi.org/10.1007/s10531-018-01684-6]
-
Fuller T, Morton DP, Sarkar S. 2008. Incorporating uncertainty about species’ potential distributions under climate change into the selection of conservation areas with a case study from the Arctic Coastal Plain of Alaska. Biol. Conserv. 141(6):1547–1559. doi:10.1016/j.biocon.2008.03.021.
[https://doi.org/10.1016/j.biocon.2008.03.021]
-
Guisan A, Broennimann O, Engler R, Vust M, Yoccoz NG, Lehmann A, Zimmermann NE. 2006. Using niche-based models to improve the sampling of rare species. Conserv. Biol. 20(2):501–511. doi:10.1111/j.1523-1739.2006.00354.x.
[https://doi.org/10.1111/j.1523-1739.2006.00354.x]
-
Guisan A, Weiss SB, Weiss AD. 1999. GLM versus CCA spatial modeling of plant species distribution. Plant Ecol. (143):107–122.
[https://doi.org/10.1023/A:1009841519580]
-
Guisan A, Zimmermann NE. 2000. Predictive habitat distribution models in ecology. Ecol Modell. 135(2):147–186. doi:10.1016/S0304-3800(00)00354-9.
[https://doi.org/10.1016/S0304-3800(00)00354-9]
-
Gustafson EJ, Miranda BR, De Bruijn AMG, Sturtevant BR, Kubiske ME. 2017. Do rising temperatures always increase forest productivity? Interacting effects of temperature, precipitation, cloudiness and soil texture on tree species growth and competition. Environ Model Softw 97:171–183. doi:10.1016/j.envsoft.2017.08.001.
[https://doi.org/10.1016/j.envsoft.2017.08.001]
- Han KS, Jeon JI, Lee JS. 2013 Apr. A pollinating insect of the endangered species, Aconitum coreanum (Lev.) Lev. Proceedings of the Korean Society of Applied Entomolohy Conference.:218.
-
Hattab T, Albouy C, Lasram FBR, Somot S, Le Loc’h F, Leprieur F. 2014. Towards a better understanding of potential impacts of climate change on marine species distribution: a multiscale modelling approach. Glob. Ecol. Biogeogr. 23(12):1417–1429. doi:10.1111/geb.12217.
[https://doi.org/10.1111/geb.12217]
-
Hutchinson GE. 1957. Concluding remarks. Cold Spring Harb. Symp. Quant. Biol. (22):415–427.
[https://doi.org/10.1101/SQB.1957.022.01.039]
-
Hwang SH, Lee jin W, La EH, Ahn JK. 2020. Flora of the vascular plants of the Baekdudaegan conservation area: Deok-chi to Yuk-sim-nyeong. Kor. J. Plant Tax. 50(1):56–79. doi:10.11110/kjpt.2020.50.1.56. (in Korean with English abstract)
[https://doi.org/10.11110/kjpt.2020.50.1.56]
-
Ikegami M, Jenkins TAR. 2018. Estimate global risks of a forest disease under current and future climates using species distribution model and simple thermal model – Pine Wilt disease as a model case. For. Ecol. Manag. 409:343–352. doi:10.1016/j.foreco.2017.11.005.
[https://doi.org/10.1016/j.foreco.2017.11.005]
-
Imperio S, Bionda R, Viterbi R, Provenzale A. 2013. Climate change and human disturbance can lead to local extinction of alpine rock ptarmigan: new insight from the western Italian Alps. PLoS One. 8(11):e81598. doi:10.1371/journal.pone.0081598.
[https://doi.org/10.1371/journal.pone.0081598]
- IPCC. 2021: Summary for Policymakers. In: Climate Change 2021: The Physical Science Basis. Contribution of Working Group I to the Sixth Assessment Report of the Intergovernmental Panel on Climate Change [Masson-Delmotte, V., P. Zhai, A. Pirani, S.L. Connors, C. Péan, S. Berger, N. Caud, Y. Chen, L. Goldfarb, M.I. Gomis, M. Huang, K. Leitzell, E. Lonnoy, J.B.R. Matthews, T.K. Maycock, T. Waterfield, O. Yelekçi, R. Yu, and B. Zhou (eds.)]. Cambridge University Press, Cambridge, United Kingdom and New York, NY, USA, pp. 3−32, doi:10.1017/9781009157896.001.
-
IPCC. 2022. Global Warming of 1.5°C: IPCC Special Report on impacts of global warming of 1.5°C above pre-industrial levels in context of strengthening response to climate change, sustainable development, and efforts to eradicate poverty. 1st ed. Cambridge University Press. [accessed 2022 Nov 29]. https://www.cambridge.org/core/product/identifier/9781009157940/type/book, .
[https://doi.org/10.1017/9781009157940]
- IUCN. 2019. The IUCN Red List of Threatened Species. Version 2019-2. https://www.iucnredlist.org, .
- IUCN. 2021. The IUCN Red List of Threatened Species. Version 2021-3. https://www.iucnredlist.org, .
- Jeremy V, Lorena F, Stephanie J, Luke S, Collin S. 2014. SDMTools: Species Distribution Modelling Tools: Tools for processing data associated with species distribution modelling exercises. R package version 11-221. https://CRAN.R-project.org/package=SDMTools, .
-
Jiménez-Valverde A. 2012. Insights into the area under the receiver operating characteristic curve (AUC) as a discrimination measure in species distribution modelling. Glob. Ecol. Biogeogr. 21(4):498–507. doi:10.1111/j.1466-8238.2011.00683.x.
[https://doi.org/10.1111/j.1466-8238.2011.00683.x]
- Joe K. 2019. Molecular Identification and Chemical Analyses of Korean Herbal Medicines. Seoul: Seoul National University. (in Korean with English abstract)
-
Kang SY, An SY, Lee MW, Kwon SK, Lee DH, Jeon BH, Kim KJ, You YO. 2015. Effects of Aconitum koreanum extract on the growth, acid production, adhesion and insoluble glucan synthesis of streptococcus mutans. J. Physiol & Pathol Korean Med. 29(1):27–32. doi:10.15188/kjopp.2015.02.29.1.27. (in Korean with English abstract)
[https://doi.org/10.15188/kjopp.2015.02.29.1.27]
-
Kearney M, Porter W. 2009. Mechanistic niche modelling: combining physiological and spatial data to predict species’ ranges. Ecol. Lett. 12(4):334–350. doi:10.1111/j.1461-0248.2008.01277.x.
[https://doi.org/10.1111/j.1461-0248.2008.01277.x]
-
Kearney MR, Wintle BA, Porter WP. 2010. Correlative and mechanistic models of species distribution provide congruent forecasts under climate change. Conserv. Lett. 3(3):203–213. doi:10.1111/j.1755-263X.2010.00097.x.
[https://doi.org/10.1111/j.1755-263X.2010.00097.x]
-
Keith DA, Mahony M, Hines H, Elith J, Regan TJ, Baumgartner JB, Hunter D, Heard GW, Mitchell NJ, Parris KM, et al. 2014. Detecting extinction risk from climate change by IUCN Red list criteria. Conserv. Biol. 28(3):810–819. doi:10.1111/cobi.12234.
[https://doi.org/10.1111/cobi.12234]
-
Kelly AE, Goulden ML. 2008. Rapid shifts in plant distribution with recent climate change. Proc. Natl. Acad. Sci. U.S.A. 105(33):11823–11826. doi:10.1073/pnas.0802891105.
[https://doi.org/10.1073/pnas.0802891105]
-
Kim Y-C, Chae H-H, Hong B-R, Oh H-K, Lee K-H, Lee K-S. 2016. The status of endangered plants distributed in the Middle Eastern area of Korea and evaluation of the risk factors. Korean J. Environ. Ecol. 30(3):291–307. doi:10.13047/KJEE.2016.30.3.291. (in Korean with English abstract)
[https://doi.org/10.13047/KJEE.2016.30.3.291]
-
Konapala G, Mishra AK, Wada Y, Mann ME. 2020. Climate change will affect global water availability through compounding changes in seasonal precipitation and evaporation. Nat Commun. 11(1):3044. doi:10.1038/s41467-020-16757-w.
[https://doi.org/10.1038/s41467-020-16757-w]
-
Koo KA, Kong W-S, Nibbelink NP, Hopkinson CS, Lee JH. 2015. Potential effects of climate change on the distribution of cold-tolerant evergreen broadleaved woody plants in the Korean peninsula. PLoS One. 10(8):e0134043. doi:10.1371/journal.pone.0134043.
[https://doi.org/10.1371/journal.pone.0134043]
-
Koo KA, Park S-U. 2021. A review of ecological niche theory from the early 1900s to the present. Korean J. Environ. Ecol. 35(4):316–335. doi:10.13047/KJEE.2021.35.4.316. (in Korean with English abstract)
[https://doi.org/10.13047/KJEE.2021.35.4.316]
-
Koo KA, Park SU. 2022. The effect of interplays among climate change, land-use change, and dispersal capacity on plant redistribution. Ecol. Indic. 142:109192. doi:10.1016/j.ecolind.2022.109192.
[https://doi.org/10.1016/j.ecolind.2022.109192]
-
Koo KA, Park SU, Hong S, Jang I, Seo C. 2018. Future distributions of warm-adapted evergreen trees, Neolitsea sericea and Camellia japonica under climate change: ensemble forecasts and predictive uncertainty. Ecol Res. 33(2):313–325. doi:10.1007/s11284-017-1535-3.
[https://doi.org/10.1007/s11284-017-1535-3]
- KMA. 2012. The Climate Atlas of Korea. Seoul: Korea Meteorological Administration.
- KMA. 2022. Annual Climatological Report. Seoul: Korea Meteorological Administration.
-
Kremen C, Cameron A, Moilanen A, Phillips SJ, Thomas CD, Beentje H, Dransfield J, Fisher BL, Glaw F, Good TC, et al. 2008. Aligning conservation priorities across taxa in Madagascar with high-resolution planning tools. Science. 320(5873):222–226. doi:10.1126/science.1155193.
[https://doi.org/10.1126/science.1155193]
-
Latimer AM, Wu S, Gelfand AE, Silander Jr. JA. 2006. Building statistical models to analyze species distributions. Ecol Appl. 16(1):33–50. doi:10.1890/04-0609.
[https://doi.org/10.1890/04-0609]
-
Laurance WF, Nascimento HEM, Laurance SG, Andrade A, Ribeiro JELS, Giraldo JP, Lovejoy TE, Condit R, Chave J, Harms KE, et al. 2006. Rapid decay of tree-community composition in Amazonian forest fragments. Proc. Natl. Acad. Sci. U.S.A. 103(50):19010–19014. doi:10.1073/pnas.0609048103.
[https://doi.org/10.1073/pnas.0609048103]
-
Li Y-Y, Guan S-M, Yang S-Z, Luo Y, Chen X-Y. 2012. Genetic decline and inbreeding depression in an extremely rare tree. Conserv Genet. 13(2):343–347. doi:10.1007/s10592-011-0286-x.
[https://doi.org/10.1007/s10592-011-0286-x]
-
Liu S, Deng L, Dong S, Zhao Q, Yang J, Wang C. 2014. Landscape connectivity dynamics based on network analysis in the Xishuangbanna Nature Reserve, China. Acta Oncol. 55:66–77. doi:10.1016/j.actao.2013.12.001.
[https://doi.org/10.1016/j.actao.2013.12.001]
-
Machado-Stredel F, Cobos ME, Peterson AT. 2021. A simulation-based method for selecting calibration areas for ecological niche models and species distribution models. Front. Biogeogr. 13(4). doi:10.21425/F5FBG48814.
[https://doi.org/10.21425/F5FBG48814]
-
McCluskey EM, Lulla V, Peterman WE, Stryszowska-Hill KM, Denton RD, Fries AC, Langen TA, Johnson G, Mockford SW, Gonser RA. 2022. Linking genetic structure, landscape genetics, and species distribution modeling for regional conservation of a threatened freshwater turtle. Landsc Ecol. 37(4):1017–1034. doi:10.1007/s10980-022-01420-0.
[https://doi.org/10.1007/s10980-022-01420-0]
-
McGarigal K. 1995. FRAGSTATS: Spatial Pattern Analysis Program for Quantifying Landscape Structure. U.S. Department of Agriculture, Forest Service, Pacific Northwest Research Station.
[https://doi.org/10.2737/PNW-GTR-351]
-
Merow C, Smith MJ, Silander Jr JA. 2013. A practical guide to MaxEnt for modeling species’ distributions: what it does, and why inputs and settings matter. Ecography. 36(10):1058–1069. doi:10.1111/j.1600-0587.2013.07872.x.
[https://doi.org/10.1111/j.1600-0587.2013.07872.x]
-
Merriam CH. 1898. Life Zones and Crop Zones of the United States. U.S. Government Printing Office.
[https://doi.org/10.5962/bhl.title.35423]
- NGII. 2019. The National Atlas of Korea 1st Edition. Suwon: National Geographic Information Institute.
- NGII. 2020. The National Atlas of Korea 2st Edition. Suwon: National Geographic Information Institute.
- NIBR. 2012. The 2nd National survey on the Distribution of Endangered Species 2007-2011 report. Incheon: National Institute of Biological Resources.
- NIBR. 2015. The 3rd National survey on the Distribution of Endangered Species 2012-2014 report. Incheon: National Institute of Biological Resources.
- NIBR. 2018a. An overview of Endangered species in Korea. Incheon: National Institute of Biological Resources.
- NIBR. 2018b. The 4th National survey on the Distribution of Endangered Species 2015-2017 report. Incheon: National Institute of Biological Resources.
- NIBR. 2021. The 5th National survey on the Distribution of Endangered Species 2018-2020 report. Incheon: National Institute of Biological Resources.
- NIE. 2020. The 4th National Ecosystem Survey Data book 1~3. Seocheon: National Institute of Ecology.
- NIE. 2022. Endangered species portal. National Institute of Ecology https://www.nie.re.kr/endangered_species, .
- NIER. 2006. The 2nd National Ecosystem Survey 1997-2005 Report. Incheon: National Institute of Environmental Research.
- NIER. 2013. The 3rd National Ecosystem Survey 2006-2012 Report. Incheon: National Institute of Environmental Research.
-
Nyirimigabo E, Xu Y, Li Y, Wang Y, Agyemang K, Zhang Y. 2015. A review on phytochemistry, pharmacology and toxicology studies of Aconitum. J. Pharm. Pharmacol. 67(1):1–19. doi:10.1111/jphp.12310.
[https://doi.org/10.1111/jphp.12310]
-
O’Donnell MS, Ignizio DA. 2012. Bioclimatic predictors for supporting ecological applications in the conterminous United States. 691st ed. U.S. Geological Survey Data Series.
[https://doi.org/10.3133/ds691]
-
Park G-M. 2012. Pathogenic parasites of Korean wild reptiles and amphibians: a study of implications for pathogen transmission in a changing climate. Korean J. Nat. Conserv. 6(2):94–101. (in Korean with English abstract)
[https://doi.org/10.11624/KJNC.2012.6.2.94]
- Park SU, Koo KA, Kong W-S. 2016. Potential impact of climate change on distribution of warm temperate evergreen broad-leaved trees in the Korean peninsula. J. Korean. Geogr. Soc. 51(2):201–217. (in Korean with English abstract)
-
Park SU, Koo KA, Kong W-S. 2019. Climate-related range shifts of climate-sensitive biological indicator species in the Korean peninsula: a role of dispersal capacity. J. Climate Change Res. 10(3):185–198. doi:10.15531/KSCCR.2019.10.3.185. (in Korean with English abstract)
[https://doi.org/10.15531/KSCCR.2019.10.3.185]
-
Pawar S, Koo MS, Kelley C, Ahmed MF, Chaudhuri S, Sarkar S. 2007. Conservation assessment and prioritization of areas in Northeast India: Priorities for amphibians and reptiles. Biol. Conserv. 136(3):346–361. doi:10.1016/j.biocon.2006.12.012.
[https://doi.org/10.1016/j.biocon.2006.12.012]
-
Penteriani V, Zarzo-Arias A, Novo-Fernández A, Bombieri G, López-Sánchez CA. 2019. Responses of an endangered brown bear population to climate change based on predictable food resource and shelter alterations. Glob Chang Biol. 25(3):1133–1151. doi:10.1111/gcb.14564.
[https://doi.org/10.1111/gcb.14564]
-
Riahi K, van Vuuren DP, Kriegler E, Edmonds J, O’Neill BC, Fujimori S, Bauer N, Calvin K, Dellink R, Fricko O, et al. 2017. The Shared Socioeconomic Pathways and their energy, land use, and greenhouse gas emissions implications: An overview. Glob Environ Change. 42:153–168. doi:10.1016/j.gloenvcha.2016.05.009.
[https://doi.org/10.1016/j.gloenvcha.2016.05.009]
-
Rodrigues ASL, Pilgrim JD, Lamoreux JF, Hoffmann M, Brooks TM. 2006. The value of the IUCN Red List for conservation. Trends Ecol. Evol. 21(2):71–76. doi:10.1016/j.tree.2005.10.010.
[https://doi.org/10.1016/j.tree.2005.10.010]
-
Schumaker NH. 1996. Using landscape indices to predict habitat connectivity. Ecology. 77(4):1210–1225. doi:10.2307/2265590.
[https://doi.org/10.2307/2265590]
-
de Siqueira MF, Durigan G, de Marco Júnior P, Peterson AT. 2009. Something from nothing: Using landscape similarity and ecological niche modeling to find rare plant species. Nat. Conserv. 17(1):25–32. doi:10.1016/j.jnc.2008.11.001.
[https://doi.org/10.1016/j.jnc.2008.11.001]
-
Thuiller W, Lafourcade B, Engler R, Araújo MB. 2009. BIOMOD – a platform for ensemble forecasting of species distributions. Ecography. 32(3):369–373. doi:10.1111/j.1600-0587.2008.05742.x.
[https://doi.org/10.1111/j.1600-0587.2008.05742.x]
-
Uroy L, Ernoult A, Mony C. 2019. Effect of landscape connectivity on plant communities: a review of response patterns. Landscape Ecol. 34(2):203–225. doi:10.1007/s10980-019-00771-5.
[https://doi.org/10.1007/s10980-019-00771-5]
-
Wilkinson DA, Marshall JC, French NP, Hayman DTS. 2018. Habitat fragmentation, biodiversity loss and the risk of novel infectious disease emergence. J. R. Soc. Interface. 15(149):20180403. doi:10.1098/rsif.2018.0403.
[https://doi.org/10.1098/rsif.2018.0403]
-
Xu J-B, Luo Y-Y, Huang S, Gao F, Zhou X-L. 2020. Four new diterpenoid alkaloids from the roots of Aconitum coreanum. Chem. Biodivers. 17(1):e1900600. doi:10.1002/cbdv.201900600.
[https://doi.org/10.1002/cbdv.201900600]
- Yoon S-J, Kim J-H, Lee K-H, Kwon H-J, Chun S-S, Cho Y-J. 2005. Antimicrobial effects and antioxidative activity of Baek-bu-ja (Aconiti koreani Rhizoma) by extraction solvent ratio. Appl. Biol. Chem. 48(3):258–262.
-
Zhao R, Chu X, He Q, Tang Y, Song M, Zhu Z. 2020. Modeling current and future potential geographical distribution of Carpinus tientaiensis, a critically endangered species from China. Forests. 11(7):774. doi:10.3390/f11070774.
[https://doi.org/10.3390/f11070774]