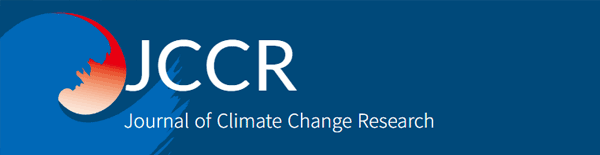
가을 가뭄 기간의 토양수분 함량과 토양온도 추정모형 개발
Abstract
This study focus on development of the estimation models for soil water content (Qv), soil temperature (Ts) and difference (dT) between air temperature (Ta) and Ts (dT=Ta-Ts). at 15 cm depth in Loam under Napa Cabbage cultivation. The cation process of the models were based on the error analysis criteria. Principle results are as follows; (1) The estimation model for Qv according to elapsed drought was developed as a sigmoid function (SWSM: sigmoid model for Qv) jufged 『Fit well』. (2) Results indicated a positive correlation between Ta and Ts, with higher Ta corresponding to higher Ts. (3) The estimation model for Ts as a three dimension function considering both Ta and Qv (3DTs: three dimension model for Ts), was found to be a 『Unfit』 for the data set. (4) The linear regressions for Ts and dT as the LRTs (linear regression model for Ts) and LRdT (linear regression model for dT) judged 『Unfit』 and 『Fit』, respectively. (5) An analysis revealed the higher Ta, combined with lower water contents, led to an increase in dT. (6) The estimation model for dT as a three dimension function considering both Ta and Qv (3DdT: three dimension model for dT), was found to be a very good fit for the data set. (7) The estimation model for Ts (TsFdT: Ts model from dT) using the estimation model for dT (3DrdT: three dimension model by Dr for dT), was found to be a good fit for the data set. In summary, this study successfully developed estimation models for Qv, Ts and dT, employing both sigmoid function and three dimension function approaches. The models were vigorously evaluated using error analysis criteria, proving valuable insights into soil water and temperature dynamics in Loam during drought season in Autumn.
Keywords:
Elapsed Drought, Soil Water Tension, Soil Water Content, Soil Temperature, Three Dimension Function1. 서론
『한국 기후변화 평가보고서 2020』(KMA, 2020)에 의하면, 토양수분은 지표의 증발산량에 영향을 미치고 대기와 지면의 에너지 분배 및 물 수지(Water budget)를 조절하여 기후시스템에 영향을 미친다(Seneviratne et al., 2010). 우리나라 지표 기온 변동 및 추세에 대한 이해를 높이기 위해 향후 우리나라 지역에서 토양수분의 장·단기적인 변동 및 추세 분석과 더불어 온도 추세와의 관련성을 이해하기 위한 토양수분의 변화에 대하여 정성적 및 정량적 구명이 필요하다(KMA, 2020). 토양의 수리학적 특성은 토양온도 (Ts)와 매우 깊은 연관성을 나타낸다(Alsherif and McCartney, 2016; Lahoori et al., 2021; Pham and Sutman, 2023). 기후변화 분야 연구에서 온도 요인은 대단히 중요한 요인이다(Kim et al., 2014; Watson and Challinor, 2013). 기온 (Ta)뿐 아니라 Ts 역시 환경 변화의 중요한 지표 중 하나이며, Ts는 식물생육과 토양수분 상태에 큰 영향을 미친다(Li et al., 2018; Urqueta et al., 2018). 따라서 Ts는 토양수분과 식물의 농업적 한발을 예측하는 데도 이용되고 있다(Ferreira and Duarte, 2019; Muro et al., 2018). 특히, Ts는 불포화 토양의 작용에 결정적인 영향을 미친다는 많은 연구가 있고 (Delage, 2013; Hashemi et al., 2022; Pham and Sutman, 2023; Pham et al., 2023; Thota and Vahedifard, 2021), 토양 경도에 미치는 Ts의 영향 연구(Garcia et al., 2023), 토양 호흡에 미치는 Ts의 영향 연구(Lloyd and Taylor, 1994; Ning et al., 2020) 등이 있으며, 특히, 작물 생육에 미치는 영향은 Ta보다 Ts가 더 크다는 연구 결과도 있다(Chakraborty et al., 2021). Ts는 기상뿐 아니라 Qv, 식생 등에 따라 종합적으로 변화하지만, Ta가 Ts 변화에 영향을 미치는 가장 중요한 인자라는 연구 결과(Smith et al., 2010)가 있으며, Kang et al. (2000)은 Ta과 Ts는 깊은 상관관계가 있다 라고 하였다. 따라서 Ta가 변하면 Ts도 이에 영향을 받아 변하게 되므로 Ta 변화에 대하여 Ts는 어떻게 변하는지 구명할 필요가 있다. 그러나 이들을 측정한 원 데이터(Raw data) 또는 상관 분석 등에 의하여 개략적인 변화성은 파악이 되지만, 이들만 가지고는 토양수분이나 온도 등의 변화성을 계량적으로 산정하기 어려우므로, 모델링(Modeling)이라는 과정을 통하여 실측치에 근거한 추정모형을 설정하고 설정된 모형을 분석하여 그 변동성을 계량적으로 파악할 수 있다. 따라서 온도 변화와 관련한 모델링 연구도 많이 수행되고 있다(Derradji and Aiche, 2014; Fu et al., 2018; Islam et al., 2021). 그러나 대부분은 Ta 및 Ts에 관한 연구 결과이었으며, Ta와 Ts의 온도 차이(dT=Ta-Ts)에 관한 연구 결과는 찾아보기 어려웠다. 온도 변화 관련 연구에서 Ta 및 Ts 그 자체도 중요한 요인이지만, 상대적인 온도 개념인 dT 역시 중요한 요인이라고 사료 된다(Eom, 2023). 따라서 본 연구에서는 Ta 및 Ts 뿐만 아니라 dT에 대한 모델링을 수행하였다. 또한, 자연상태의 토양수분 상태나 온도 등을 측정할 경우 그 측정치와 모형에 의한 추정치는 오차를 포함하고 있다. 이 오차가 참값과 어느 정도 변이를 나타내는가를 판단하는 것이 정확도(Accuracy) 분석이나 자연현상의 측정에는 대부분 경우 진정한 참값을 알 수 없으므로, 모형에 의한 추정치가 실측치와 비교하여 어느 정도 오차를 포함하고 있는가에 근거하여 그 모형의 적절성을 판단하게 된다. 이 경우 Cairo (2020)은 오차는 오류의 동의어가 아니고 불확실성(Uncertainty)의 동의어라 하였다. 일반적으로 모형의 적절성은 다음과 같은 세 가지의 관점(Liemohn et al., 2021)에서 판단하게 된다. 첫째, 확실성(Certainty)의 관점이다. 이를 판단하는 지표로서는 평균제곱근 편차(Root mean square error, RMSE: Wang and Li, 2010; Zhang et al., 2021), Nash & Sutcliffe 계수(NSE: Nash and Sutcliffe, 1970) 등이 주로 사용되고 있다. 본 연구에서는 RMSE를 확실성의 지표로 삼고 산출하였다. 둘째, 상호관련성(Association) 관점이다. 변량 간의 상관관계를 분석하여 결정계수(Coefficient of determination: R2)를 지표로 삼아 통계적 유의성이 인정되는가를 판단하게 된다. 하지만 모형의 검증을 위해서 R2 하나에만 근거하여 모형의 적절성을 판단하는 것은 올바른 모형검증이라고 할 수 없다(Krause et al., 2005; Legates and McCabe, 1999). 이 경우 Cairo (2020)은 변량 간의 상관관계는 인과관계를 뜻하는 것이 아니라고 강조하였다. 셋째, 정밀도(Precision)의 관점이다. 정밀도 분석은 오차 분석 이론에 바탕을 두고 상대정밀도(Relative sensitivity, RS)를 지표로 삼으며 (Mandel, 1964; Shein et al., 2013), 모형에 의한 추정치 (E) 표준편차(Standard deviation, STD)의 실측치 (M) STD에 대한 비율인 실측치 기준 표준편차 비율(Ratio of standard deviation, RSTD)을 지표로 삼기도 한다(Liemohn et al., 2021). 본 연구에서는 RSTD와 M과 E의 직선 회귀식의 기울기 (a) 값 및 RS[E/M] 등을 정밀도의 지표로 삼고 산출하였다. 본 연구는 가을배추 재배 포장에서 Qv, Ta 및 Ts를 측정하고, Qv 변화에 대하여 가뭄 지속에 따른 시계열 분석을 수행함과 동시에 Ta 및 Qv 변화에 따른 Ts와 dT의 변화양상을 분석하고, 이들에 대한 적절한 추정모형(Model)의 함수 형태를 선정하고 최소제곱법에 따라 오차가 최소인 계수를 산정하여 모형을 설정하였으며, 확실성, 상호관련성 및 정밀도의 세 가지 관점에서 모형의 적절성을 검증하고, 설정된 모형들을 분석하여 Qv, Ts 및 dT의 변동성을 구명코자 실시하였다.
2. 재료 및 방법
본 연구는 전라북도 완주군 이서면 반교리 707-19 농촌진흥청 국립원예특작과학원 시험 포장(동경 147.033677도, 위도 35.840791도)에 가을배추(품종명 : 청명 가을)를 2022년 9월 7일 정식하여 자연 강우에 의존하는 무 관수 재배 기간 중 강우가 없었든 2022년 10월 11일부터 2022년 11월 3일의 24일 동안 Qv, Ta 및 Ts를 측정하였다. Ts (oC) 측정 센서(모델명: TENS21)와 Qv (m3 m-3, %) 측정 센서(모델명: TEROS12)를 지하 15 cm에 설치하였고, Data logger (모델명; ZL6)를 통하여 10분 간격으로 자동 수집한 후 무선통신 시스템을 이용하여 Serve에 송신하였다. Ta는 기상장치(모델명: ENCOSYS)와 Data logger (모델명: DT80 Series4)를 통하여 5분 간격으로 자동 수집한 후 무선통신 시스템을 이용하여 Serve에 송신하였다. 본 연구에 사용된 데이터는 매일 10:00에 측정된 데이터이다. 본 연구 포장의 토성은 양토(Loam)이었다.
가뭄 지속 기간 (Dr)에 따른 Qv를 추정할 수 있는 모형을 식 (1)과 같이 Sigmoid 함수(Eom and Eom, 2008; Lieth et al., 1996; Yin et al., 2003)로 선정 (SWSM)하고 최소제곱법에 의해 오차가 최소인 계수를 산정하여 모형을 개발하였다.
(1) |
- 단, E(Qv): 토양수분 함량 추정치, Yo, a, Xo, b : 계수
Ta에 대한 Ts 및 dT의 직선 회귀식(Linear regressions: 식 2)을 Ts 및 dT의 추정모형 (LRTs 및 LRdT)으로 선정하고 최소제곱법에 따라 오차가 최소인 계수를 산정하여 모형을 개발하였다.
(2) |
- 단, a, b : 계수
Ta과 Qv의 두 가지 변량에 대한 함수로 나타낸 Ts 및 dT의 추정모형을 식 (3)과 같이 삼차원 함수(3DTs 및 3ddT)로 선정하고 최소제곱법에 따라 오차가 최소인 계수를 산정하여 모형을 개발하였다.
(3) |
- 단, Yo, a, b : 계수
Ta과 Dr의 두 가지 변량에 대한 함수로 나타낸 dT의 추정모형을 식 (4)와 같이 삼차원 함수 (3DrdT)로 선정하고 최소제곱법에 따라 오차가 최소인 계수를 산정하여 모형을 개발하였다.
(4) |
- 단, Yo, a, b : 계수
모형검증을 위한 지표로 삼은 RMSE는 식 (5), RMSE의 실측치 STD에 대한 비율인 실측치 기준 RMSE 비율(The ratio of RMSE to STD of measurement, RRMSEM)은 식 (6), RMSE의 모형에 의한 추정치 STD에 대한 비율인 추정치 기준 RMSE 비율(the ratio of RMSE to STD of estimation, RRMSEE)는 식 (7), RSTD는 식 (8)과 같이 산정하였다(Moriasi et al., 2007; Naseri et al., 2021).
(5) |
- 단, Ei : 모형에 의한 추정치(Estimated value)
- Mi : 실측치(Observed value)
- n : sample 수
(6) |
(7) |
(8) |
이 경우 확실성 관점에서 RRMSEM와 RRMSEE의 두 가지 값이 모두 0.5 이하이면 그 모형이 『매우 적절함』, 한 가지 값이라도 0.5 ~ 1.0이면 그 모형이 『적절함』이라고 판정한다. 상호관련성 관점에서 추정모형의 R2값에 대하여 통계적으로 유의성 여부에 근거하여 판정하나, 비록 통계적 유의성이 인정되어도 R2값이 낮은 경우는 『적절치 않음』이라고 판정한다. 또한, 정밀도 관점에서 RSTD의 값이 1.0 이하이면 그 모형이 『매우 적절함』 내지 『적절함』이라고 판정한다(Liemohn et al., 2021). 정밀도 관점의 또 다른 기준인 Ta과 Ts 및 dT의 실측치 (M)에 대한 추정모형에 의한 추정치 (E)의 상관관계에 대한 상대정밀도 (Relative sensitivity of estimation to measurement, RS[E/M]) 분석은 식 9부터 식 12까지의 과정을 통하여 분석하였으며, 이 경우 RS[E/M]) 값이 클수록, 또한 M에 대한 E의 상관관계를 일 대 일 line 상(절편이 0)의 기울기 (a) 값이 1.0에 가까울수록 정밀도가 높다고 판정한다(al Irsyard et al., 2019; Goodman, 1960).
(9) |
(10) |
(11) |
(12) |
- 단, SE : E의 표준오차(Standard error)
- SM : M의 표준오차(Standard error)
- R2 : M에 대한 E의 직선 회귀식에 대한 결정계수
3. 결과 및 고찰
3.1. 토양수분 함량 변화양상 및 추정모형 개발
가뭄 경과에 따른 Qv의 변화양상을 보면(Fig. 1), 가뭄 경과 이틀까지는 Qv의 변화가 거의 없었는데 이는 토양수분이 충분한 상태에서 중력에 견디지 못해 빠져나가는 수분 즉, 중력수가 빠져나가는데 소요된 기간이었다고 사료 된다. 그 후부터 Qv는 급격히 감소하는 양상을 보였으며, 13일경과 때(Fig. 1의 왼쪽 원으로 표시된 부분) “Bottleneck effect” (Lal and Shukla, 2004) 라는 굴곡 현상이 나타나면서 Qv의 감소 기울기가 완만하게 변하기 시작했다. 이는 이 시점에 이르러 공극의 크기와 모양이 제각각인 토양의 공극 중에서 직경이 비교적 큰 공극에 보유되어 있던 수분이 모두 빠져나갔다는 것으로 해석되며, 22일경과 때 (Fig. 1의 오른쪽 원으로 표시된 부분) 또다시 “Bottleneck effect” 현상이 나타나면서 Qv의 감소 기울기가 더욱 완만해져 그 후부터는 거의 변화가 없었다. 이는 이 시점에 이르러 직경이 비교적 작은 공극에 보유되어 있던 수분마저 모두 빠져나갔다는 것으로 해석된다. 가뭄 경과 24일 때 Qv는 가뭄 시작 때의 31.40%로부터 22.1%까지 낮아졌다(Fig. 1 및 Table 1).
Dr에 따른 Qv를 추정할 수 있는 모형을 Sigmoid 함수로 선정(SWSM)하고 최소제곱법에 의해 오차가 최소인 계수를 산정하여 개발한 모형(Fig. 2: Left)은 식 (13)과 같았다.
(13) |
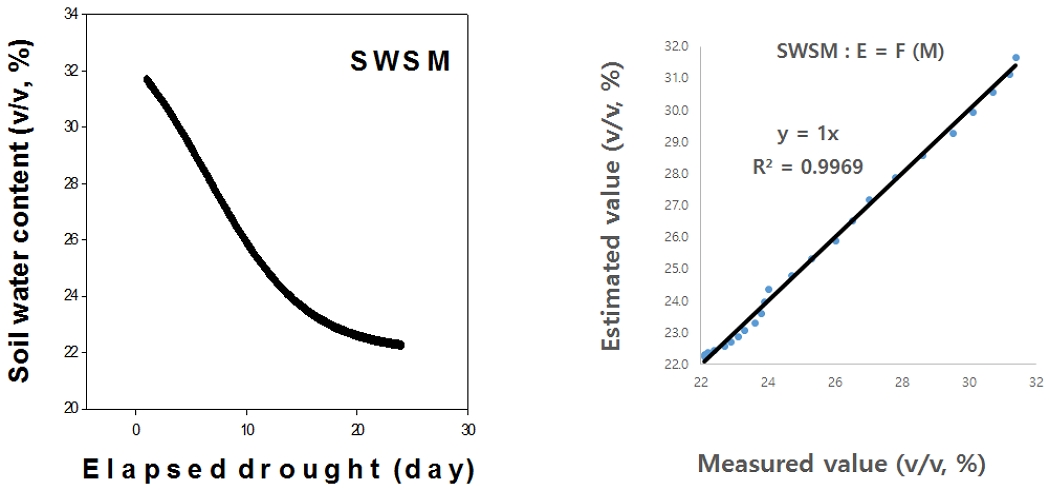
Estimation model (SWSM : Left) for soil water contents (Qv) and the relationship between measured and estimated value for Qv (Right)
SWSM 모형 검증결과, 확실성 관점에서 볼 때, Qv의 RMSE는 0.173이었으며, RRMSEM과 RRMSEE 모두 0.055로서 매우 작았기에(Table 2) 『매우 적절함』으로 판정되었다. 상호관련성 관점에서 볼 때, 모형의 R2값이 0.997로서 통계적으로 고도의 유의성이 인정되어 『매우 적절함』으로 판정되었다. 정밀도 관점에서 볼 때, RSTD는 0.998로서 1.0 이하이었으며, SWSM 모형에 의한 추정치와 실측치의 상호 1 대 1 line 선상의 상관관계(Fig. 2: Right)에 근거한 a 값은 1.00이었고, RS[E/M]는 0.997로서 매우 높았으므로(Table 3) 『매우 적절함』으로 판정되었다. 이와 같은 결과로 볼 때, SWSM은 Qv의 추정모형으로서는 『매우 적절함』으로 판단되었다.

The parameters and indices of measured (M) and estimated value (E) to verify the certainty and association of the SWSM for soil water contents (QV)
3.2. 토양온도 및 기온과 온도 차이 추정모형 개발
가뭄 경과에 따른 Ta 및 Ts의 경시적 변화양상을 보면(Fig. 3: Left 및 Table 4), 가뭄 경과 24일 동안의 Ta 및 Ts의 평균온도는 각각 18.31 및 14.42oC 이었으며 Ts가 Ta보다 낮았다. 또한, 최고온도와 최저온도의 차이인 범위(Range)는 Ta 및 Ts 각각 13.60 및 6.10oC 이었으며 Ts는 Ta과 비하여 온도의 변화폭이 적었다. Ta는 하루 또는 이틀 간격으로, Ts는 이틀 또는 사흘 간격으로 오르락내리락 하지만 전체 경향은 온도가 계속 낮아지는 양상을 보였으나(Fig. 3: Left), dT는 하루 또는 사흘 간격으로 오르락내리락 하지만 전체 경향은 온도가 증가 또는 감소 등 일정한 경향을 보이지 않았다(Fig. 3: Right).
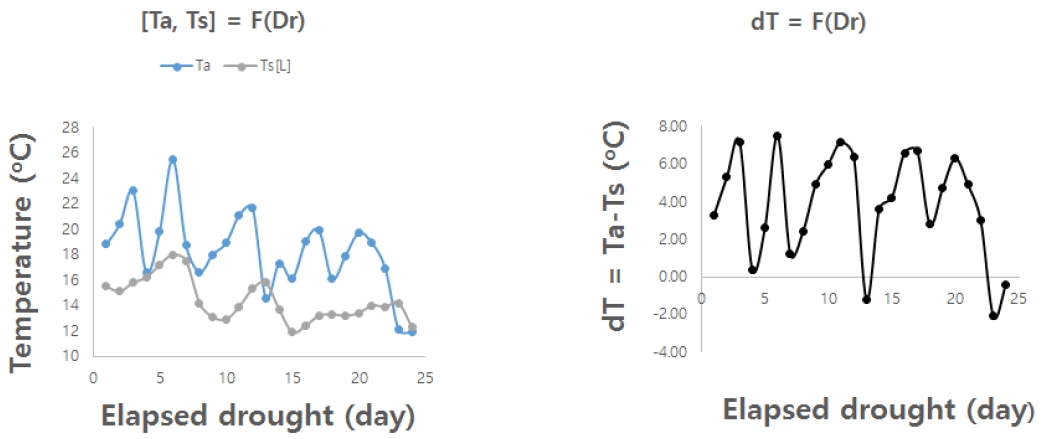
Changes in air (Ta) and soil temperature (Ts) (Left), and the difference (dT=Ta-Ts: Light) according to elapsed drought (Right)
3.2.2.1. 기온과 토양온도의 상관관계를 이용한 토양온도(Ts) 추정모형
Ta 변화에 따른 Ts의 변화 추정모형을 Ta에 대한 직선 회귀 식인 일차함수(LRTs)로 선정하고 최소제곱법에 따라 오차가 최소인 계수를 산정하여 식 (14)와 같이 모형을 설정하였다(Fig. 4: Left), Ta가 높을수록 Ts도 높은 경향이었다. 이와 같은 결과는 Ta가 Ts 변화에 영향을 미치는 가장 중요한 인자라는 연구(Grosse et al., 2016; Park et al., 2014) 결과 및 Ta과 Ts는 깊은 상관관계가 있다는 연구(Kang et al., 2000) 결과와 유사한 결과를 나타내었다. 또한, 이와 같은 결과는 Ta가 높을수록 태양열이라는 수열 인자(Input parameter of heat)의 영향으로 Ta와 Ts는 정비례 관계를 나타내었다고 사료 된다.
(14) |
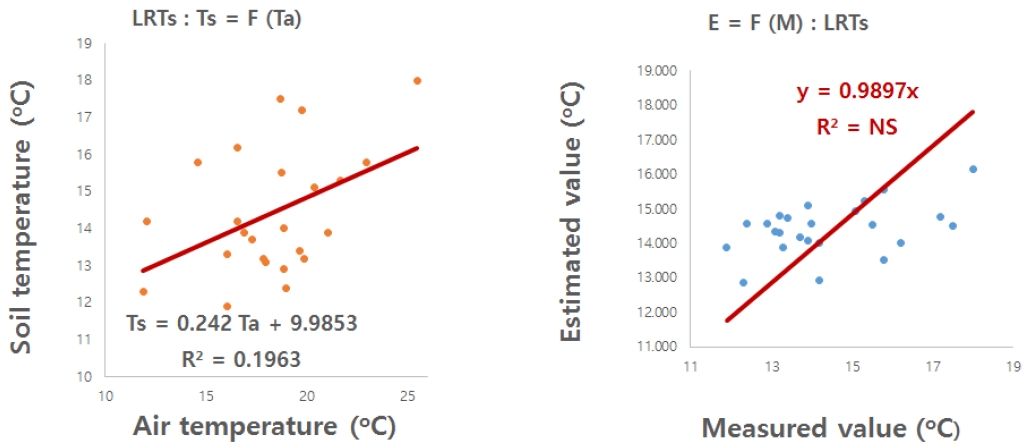
Relationship between air and soil temperature (Left), and measured and estimated value by the LRTs for soil temperature (Right)
LRTs 모형 검증결과, 확실성 관점에서 볼 때, RMSE는 1.475이었으며, RRMSEM과 RRMSEE는 각각 0.878과 0.981으로서 (Table 5) 0.5 ~ 1.0 이하이었기에 『적절함』으로 판정되었다. 상호관련성 관점에서 볼 때, R2값은 0.196으로서 비록 통계적으로 5%의 유의수준에서 유의성은 인정되었으나 그 값이 너무 낮기에 『적절치 않음』으로 판정되었다(Table 5 및 Fig. 4: Left). 정밀도 관점에서 볼 때, RSTD는 0.443이었으나 a 값은 통계적으로 유의성이 없었고 RS[E/M]는 워낙 낮아서 (Table 6 및 Fig. 4: Right)『적절치 않음』으로 판정되었다. 이와 같은 결과로 볼 때, LRTs은 Ts의 추정모형으로서는 『적절치 않음』으로 판단되었다.

The parameters and indices of measured (M) and estimated value (E) to verify the certainty and association of the LRTs for soil temperature (Ts)

The parameters and indices of measured (M) and estimated value (E) to verify the precision of the LRTs for soil temperature (Ts)
3.2.2.2. 기온(Ta)과 토양수분 함량(Qv)에 의한 토양온도(Ts) 추정모형
비록 Ts가 Ta과 정비례 관계가 있으나, Ts에 영향을 미치는 요인이 Ta 하나라고 보기는 어렵다고 판단되어 Qv라는 또 다른 요인과 복합적인 작용의 결과에 따라 Ts가 변할 것이라는 가설하에, Ta와 Qv의 두 가지 변량에 대한 함수로 나타내는 Ts의 추정모형을 삼차원 함수(3DTs)로 선정하고 최소제곱법 방법을 이용하여 오차가 최소인 계수를 산정하여 모형을 설정한 결과, Fig. 5: Left 및 식 (15)와 같다. 즉, Ta가 높을수록 또 Qv가 높을수록 Ts는 높았다. 이와 같은 결과는 태양열이라는 수열 인자의 영향으로 Ta과 Ts는 정비례 관계가 있고, 또한 Qv가 높을수록 토양수분의 비열(specific heat)이 상대적으로 높아서 방사율이 낮은 것(Lal and Shukla, 2004)에 기인 되었다고 사료 된다.
(15) |
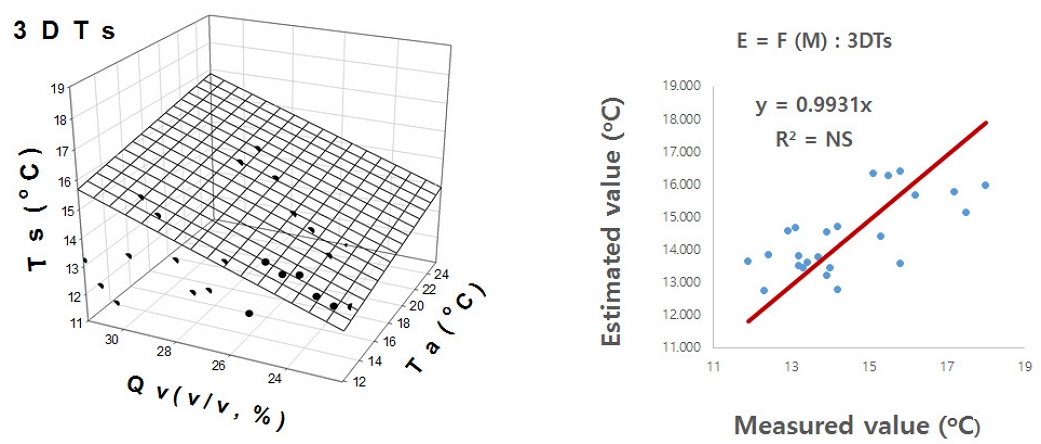
Estimation model (3DTs) for soil temperature (Ts) as a function of air temperature (Ta) and soil water content (Qv) (Left), and the relationship between measured and estimated value for Ts (Right)
3DTs 모형 검증결과, 확실성 관점에서 볼 때, RMSE는 1.207이었으며, RRMSEM 및 RRMSEE는 각각 0.718 및 1.056으로서 0.5 ~ 1.0 범위로 볼 수 있기에(Table 7) 『적절함』으로 판정되었다. 상호관련성 관점에서 볼 때, R2값은 0.462로서 비록 통계적인 유의성은 인정되었으나 그 값이 낮으므로 『적절치 않음』으로 판정되었다. 정밀도 관점에서 볼 때, RSTD는 0.680이었으며 a 값은 통계적으로 유의성이 없었고 RS[E/M]은 워낙 낮았기에(Fig. 5: Right 및 Table 8) 『적절치 않음』으로 판정되었다. 이와 같은 결과로 볼 때, Ts의 추정모형으로서 3DTs는 『적절치 않음』으로 판단되었다.

The parameters and indices of measured (M) and estimated value (E) to verify the certainty and association of the 3DTs for soil temperature (Ts)
3.2.3.1. 기온(Ta)과 온도 차이(dT)의 상관관계를 이용한 dT 추정모형
Ta 변화에 따른 dT의 변화 추정모형을 Ta에 대한 직선 회귀 식인 일차함수(LRdT)로 선정하고 최소제곱법에 따라 오차가 최소인 계수를 산정하여 식 (16)과 같이 모형을 설정하였다(Fig. 6: Left), Ta가 높을수록 dT는 높은 경향이었다. 이와 같은 결과는 공기보다 토양의 비열이 더 크기 때문에 방사율이 상대적으로 적으므로(Lal and Shukla, 2004), Ta가 계속 낮아질수록 Ta보다 Ts의 낮아지는 정도가 더 작았던 것에 기인 되었다고 사료 된다.
(16) |
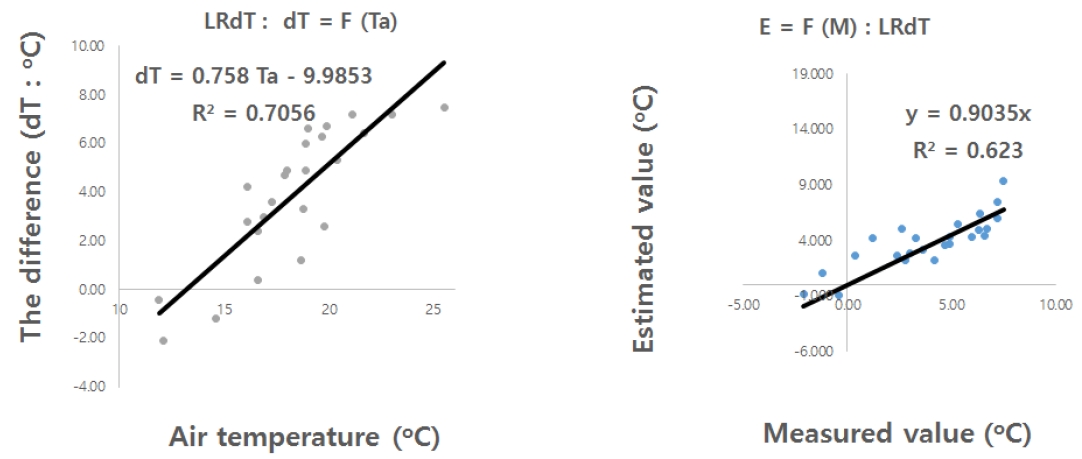
Relationship between air and soil temperature (Left), and measured and estimated value by the LRTs for soil temperature (Right)
LRdT 모형 검증결과, 확실성 관점에서 볼 때, RMSE는 1.475이었으며, RRMSEM과 RRMSEE는 각각 0.531과 0.6321이었기에(Table 9). 『적절함』으로 판정되었다. 상호관련성 관점에서 볼 때, R2값은 0.706으로서 통계적으로 유의성이 인정되어 『적절함』으로 판정되었다(Table 9 및 Fig. 6: Left). 정밀도 관점에서 볼 때, RSTD는 0.840이었으며 a 값은 0.931로서 1.0에 근접하였고 RS[E/M]은 0.632이었기에(Table 10 및 Fig. 6: Right)『적절함』으로 판정되었다. 이와 같은 결과로 볼 때, LRdT는 dT의 추정모형으로서 『적절함』으로 판단되었다.

The parameters and indices of measured (M) and estimated value (E) to verify the certainty and association of the LRdT for the difference (dT=Ta-Ts)

The parameters and indices of measured (M) and estimated value (E) to verify the precision of the LRdT for the difference (dT=Ta-Ts)
3.2.3.2. 기온(Ta)과 토양수분 함량(Qv)에 의한 온도 차이(dT) 추정모형
Ts 경우처럼 dT 또한 Ta와 Qv의 두 가지 변량에 대한 함수로 나타내는 dT의 추정모형을 삼차원 함수(3ddT)로 선정하고 최소제곱법 방법을 이용하여 오차가 최소인 계수를 산정하여 모형을 설정한 결과 Fig. 7: Left 및 식 (17)과 같다. 즉, Ta가 높을수록 또 Qv가 낮을수록 dT는 높았다. 이와 같은 결과는 Ta가 계속 낮아질수록 공기보다 토양의 비열이 더 크기 때문에 Ts가 낮아지는 정도가 더 작았던 것에 기인하여 Ta와 dT는 정비례 관계를 나타내었고, 본 연구의 시험 시기가 온도가 계속 낮아지는 시기이었기에 Qv가 낮을수록 Qv가 높은 경우보다 상대적으로 비열이 낮아 열 방사율이 더 크기 때문에(Lal and Shukla, 2004) Ts의 낮아지는 정도가 더 빠른 것에 기인 되었다고 사료된다.
(17) |
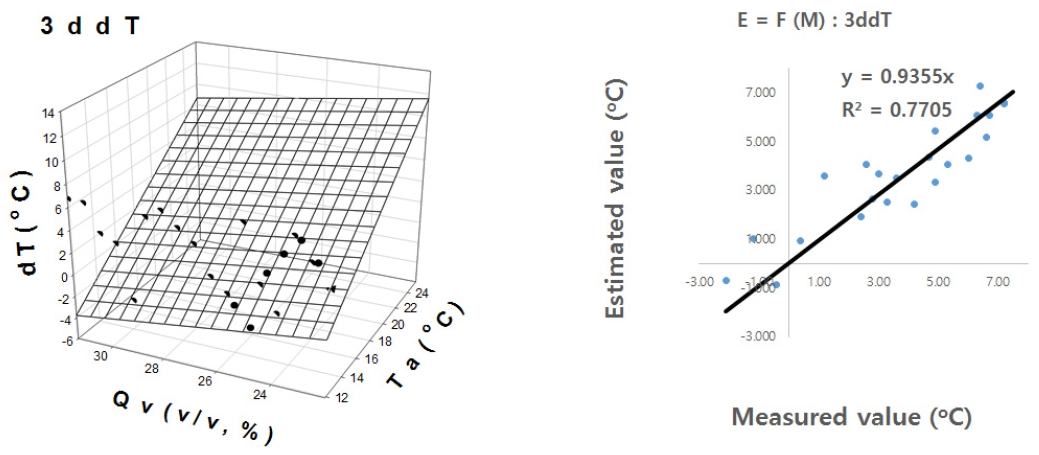
Estimation model (3ddT) for the difference (dT) as a function of air temperature (Ta) and soil water content (Qv) (Left), and the relationship between measured and estimated value for dT (Right)
3ddT의 검증결과, 확실성 관점에서 볼 때, RMSE는 1.207이었으며, RRMSEM 및 RRMSEE는 각각 0.435 및 0.485로서 0.5 이하이었기에(Table 11) 『매우 적절함』으로 판정되었다. 상호관련성 관점에서 볼 때, R2값은 0.803으로서 통계적으로 고도의 유의성이 인정되어 『매우 적절함』으로 판정되었다. 정밀도 관점에서 볼 때, RSTD는 0.896이었으며 a 값은 0.936으로서 1.0에 근접하였고 RS[E/M]는 0.824 (Fig. 7: Right 및 Table 12)으로서 『매우 적절함』으로 판정되었다. 이와 같은 결과로 볼 때, 3ddT는 dT의 추정모형으로서 『매우 적절함』으로 판단되었다.

The parameters and indices of measured (M) and estimated value (E) to verify the certainty and association of the 3ddT for the difference (dT=Ta-Ts)

The parameters and indices of measured (M) and estimated value (E) to verify the precision of the 3ddT for the difference (dT=Ta-Ts)
3.2.3.3. 기온(Ta)과 가뭄 지속기간(Dr)에 의한 온도 차이(dT) 추정모형
Ta와 Dr의 두 가지 변량에 대한 함수로 나타내는 dT의 추정모형을 삼차원 함수(3DrdT)로 선정하고 최소제곱법 방법을 이용하여 오차가 최소인 계수를 산정하여 모형을 설정한 결과 Fig. 8: Left 및 식 (18)과 같다. 즉, Ta가 높을수록 또 Dr이 길수록 dT는 높았다. 이와 같은 결과는 Ta가 계속 낮아질수록 공기보다 토양의 비열이 더 크기 때문에 Ts가 낮아지는 정도가 더 작았던 것에 기인하여 Ta와 dT는 정비례 관계를 나타내었고, Dr이 길수록 Dr이 짧은 경우보다 Qv가 낮아지기 때문에 상대적으로 비열이 낮아 열 방사율이 더 크기 때문에 (Lal and Shukla, 2004) Ts의 낮아지는 정도가 더 빠른 것에 기인 되었다고 사료 된다.
(18) |
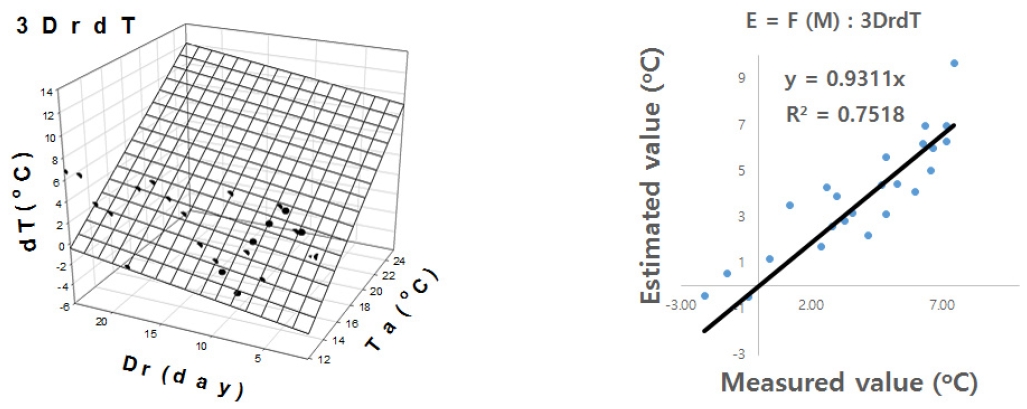
Estimation model (3DrdT) for the difference (dT) as a function of air temperature (Ta) and elapsed drought (Dr) (Left), and the relationship between measured and estimated value for dT (Right)
3DrdT의 검증결과, 확실성 관점에서 볼 때, RMSE는 1.248이었으며, RRMSEM 및 RRMSEE는 각각 0.449 및 0.506으로서 0.5 이하로 볼 수 있는 범위이었기에(Table 13) 『매우 적절함』으로 판정되었다. 상호관련성 관점에서 볼 때, R2값은 0.790으로서 통계적으로 고도의 유의성이 인정되어 『매우 적절함』으로 판정되었다. 정밀도 관점에서 볼 때, RSTD는 0.889이었으며 a 값은 0.931로서 1.0에 근접하였고 RS[E/M]는 0.807 (Fig. 8: Right 및 Table 14)으로서 『매우 적절함』으로 판정되었다. 이와 같은 결과로 볼 때, 3DrdT는 dT의 추정모형으로서 『매우 적절함』으로 판단되었다.

The parameters and indices of measured (M) and estimated value (E) to verify the certainty and association of the 3DrdT for the difference (dT=Ta-Ts)

The parameters and indices of measured (M) and estimated value (E) to verify the precision of the 3DrdT for the difference (dT=Ta-Ts)
3.2.3.4. 3DrdT를 이용한 토양온도(Ts) 추정모형 개발
본 연구에서 분석한 Ts 추정모형들 즉, LRTs 및 3DTs는 모두 『적절치 않음』으로 판단 되었기에 『매우 적절함』으로 판단된 3DrdT를 이용한 Ts의 추정모형(TsFdT)을 dT = Ta–Ts 이므로 식 (19)와 같이 선정하고, TsFdT에 의한 Ts의 추정치와 실측치와의 상관관계를 나타낸 Fig. 9이다.
(19) |
TsFdT의 검증결과, 확실성 관점에서 볼 때, RMSE는 1.248으로서 3DrdT의 경우와 같았으며, RRMSEM 및 RRMSEE는 각각 0.742 및 1.137이었기에(Table 15) 『적절함』 내지 『적절치 않음』으로 판정되었다. 상호관련성 관점에서 볼 때, R2값은 0.425로서 통계적으로 유의성이 인정되어 『적절함』으로 판정되었다. 정밀도 관점에서 볼 때, RSTD는 0.652이었으며 a 값은 0.425로서 비교적 낮았고 RS[E/M]는 1.000이었기에(Fig. 9 및 Table 16) 『적절함』으로 판정되었다. 이와 같은 결과로 볼 때, TsFdT는 dT의 추정모형으로서 『적절함』으로 판단되었다.

The parameters and indices of measured (M) and estimated value (E) to verify the certainty and association of the TsFdT for soil temperature (Ts)

The parameters and indices of measured (M) and estimated value (E) to verify the precision of the TsFdT for soil temperature (Ts)
이상의 결과를 종합하여 결론적으로 볼 때, Dr에 따른 Qv의 추정모형인 SWSM는 매우 적절하였으며, Ts에 대한 적절성이 인정되는 추정모형은 TsFdT 이었고, dT에 대한 적절성이 높은 추정모형은 3ddT 및 3DrdT 이었다.
4. 결론
본 연구는 전북 완주에 소재한 농촌진흥청 국립원예특작과학원의 양토 시험 포장에 가을배추를 재배하면서, 가뭄 기간이었든 2022년 10월 11일부터 2022년 11월 03일까지 매일 10:00에 측정된 토양수분 함량(Qv), 기온(Ta), 토양온도(Ts)와 함께 Ta와 Ts의 온도 차이(dT=Ta-Ts)을 분석하여, 가을 가뭄 지속기간(Dr)에 따른 Qv 추정모형과, Ta와 Qv에 의한 Ts 및 dT 추정모형을 개발하고 그 모형의 적절성을 검증한 결과는 다음과 같다.
첫째, Dr에 따른 Qv의 시계열 변화 모형을 식 (1-1)의 Sigmoid 함수(SWSM)로 설정하였으며, SWSM에 대한 검증결과, 『매우 적절함』으로 판단되었다.
(1-1) |
둘째, Ta에 대한 Ts의 직선 회귀 식 (식 2-1)을 Ts 추정모형 (LRTs)으로 설정하고 LRTs에 대한 검증결과, 『적절치 않음』으로 판단되었다.
(2-1) |
셋째, Ta과 Qv의 두 가지 변량에 대한 함수로 나타낸 Ts 추정모형을 식 (3-1)의 삼차원 함수(3DTs)로 설정하였다. Ts는 Ta가 높고 Qv가 높을수록 높았다. 3DTs에 대한 검증결과, 『적절치 않음』으로 판단되었다.
(3-1) |
넷째, Ta에 대한 dT의 직선 회귀식(식 4-1)을 dT 추정모형(LRdT)으로 설정하고 LRdT에 대한 검증결과, 『적절함』으로 판단되었다.
(4-1) |
다섯째, Ta과 Qv의 두 가지 변량에 대한 함수로 나타낸 dT 추정모형을 식 (5-1)의 삼차원 함수(3ddT)로 설정하였다. dT는 Ta가 높고 Qv가 낮을수록 높았다. 3ddT에 대한 검증결과, 『매우 적절함』으로 판단되었다.
(5-1) |
여섯째, Ta과 Dr의 두 가지 변량에 대한 함수로 나타낸 dT 추정모형을 식 (6-1)의 삼차원 함수(3DrdT)로 설정하였다. dT는 Ta가 높고 Dr이 길수록 높았다. 3DrdT에 대한 검증결과, 『매우 적절함』으로 판단되었다.
(6-1) |
일곱째, 3DrdT를 이용한 Ts 추정모형(TsFdT)을 식 (7-1)과 같이 설정하고. TsFdT에 대한 검증결과, 『적절함』으로 판단되었다.
(7-1) |
결론적으로 볼 때, Dr에 따른 Qv의 추정모형인 SWSM는 매우 적절하였으며, Ts에 대한 적절성이 인정되는 추정모형은 TsFdT 이었고, dT에 대한 적절성이 높은 추정모형은 3ddT 및 3DrdT 이었다.
Acknowledgments
본 논문은 농촌진흥청 연구사업(공동과제명 : 지역별 재배 적지 밭작물 작부체계 실태조사, D/B 구축 및 경제성 분석, 공동과제 번호 : PJ01528904)의 지원에 의해 수행되었습니다.
References
- al Irsyard MI, Halog A, Nepal R. 2019. Renewable energy for climate change mitigation: An analysis of uncertanity and errors. Renew Energy 130: 536-546.
- Alsherif NA, McCartney JS. 2016. Yielding of silt at high temperature and suction magnitudes. Geotech Geol Eng 34(2): 501-514.
- Cairo A. 2020. How chart lie: Getting smarter about visual information. New York: W. W. Norton. p. 198-242.
- Chakraborty PK, Banerjee S, Nath R, Samanta S. 2021. Aseessing congenial soiltemperature and its impact on root growth, grain yield of summer rice under varying water stress condition in Lower Gangetic Plain of India. J Saudi Soc Agric Sci 21(2): 98-107.
- Delage P. 2013. On the thermal impact on the excavation damaged zone around deep radioactive waste disposal. J Rock Mech Geotech Eng 5(3): 179-190.
- Derradji M, Aiche M. 2014. Modeling the soil surface temperature for natural cooling of buildings in hot climates. Procedia Comput Sci 32: 615-621.
- Eom K. 2023. Numerical approach for air temperature, soil temperature and the difference between them with Julian date under Napa cabbage (in Korean with English abstract). Korean J Soil Sci Fert 56(4): 419-430.
- Eom KC, Eom KR. 2008. Mathematics for agricultural sciences. Seoul: Daewon Publishers. p. 123-491.
- Ferreira LS, Duarte DHS. 2019. Exploring the relationship between urban form, land surface temperature and vegetation indices in a subtropiocal megacity. Urban Clim 27: 105-123.
- Fu X, Yu Z, Ding Y, Tang Y, Lü H, Jiang X, Ju Q. 2018. Analysis of influence of observation operator on sequential data assimilation through soil temperature simulation with common land model. Water Sci Eng 11(3): 196-204.
- Garcia LM, Pinyol NM, Lloret A, Soncco EA. 2023. Influence of temperature on residual strength of clayey soils. Eng Geol 323: 107220.
- Goodman LA. 1960. On the exact variance of products. J Am Stat Assoc 55(292): 708-713.
- Grosse G, Goetz S, McGuire AD, Romanovsky VE, Schuur EAG. 2016. Changing permafrost in a warming world and feedbacks to the Earth system. Environ Res Lett 11(4): 040201.
- Hashemi A, Sutman M, Abuel-Naga H. 2022. Thermomechanical response of kaolin clay-concrete interface in the context of energy geostructures. Can Geotech J 60(3): 380-396.
- Islam MA, Lubbad R, Amiri SAG, Isaev V, Shevchuk Y, Uvarova AV, Afzal MS, Kumar A. 2021. Modelling the seasonal variations of soil temperatures in the Arctic coasts. Polar Sci 30: 100732.
- Kang SS, Kim S, Oh S, Lee D. 2000. Predicting spatial and temporal patterns of soil temperature based on topography, surface cover and air temperature. For Ecol Manag 136(1-3): 173-184.
- Kim M, Lee WK, Nam K, Yu H, Choi SE, Kim CG. 2014. Estimating stand volume Pinus densiflora forest based on climate change scenario in Korea (in Korean with English abstract). J Korean For Soc 103(1): 105-112.
- KMA (Korea Meteorological Administration). 2020. Korean climate change assessment report 2020. Seoul, Korea: KMA. p. 34-35, 234-249.
- Krause P, Boyle DP, Base F. 2005. Comparison of different efficiency criteria for hydrological model assessment. Adv Geosci 5: 89-97.
- Lahoori M, Rosin-Paumier S, Masrouri F. 2021. Effect of monotonic and cyclic temperature variations on the mechanical behavior of a compacted soil. Engi Geol 290: 106195.
- Lal R, Shukla MK. 2004. Soil air and aeration. In: Principles of soil physics. New York: Marcel Dekker. p. 515-555.
- Legates DR Jr, McCabe GL. 1999. Evaluating the use of “goodness-of-fit” measures in hydrologic and hydroclimatic model validation. Water Resour Res 35(1): 233-241.
- Li X, Zhou Y, Asrar GR, Zhu Z. 2018. Creating a seamless 1 km resolution daily land surface temperature dataset for urban and surrounding areas in the conterminous United States. Remote Sens Environ 206: 84-97.
- Liemohn MW, Shane AD, Azari AR, Petersen AK, Swiger BM, Mukhopadhyay A. 2021. RMSE is not enough: Guidelines to robust data-model comparisons for magnetospheric physics. J Atmos Solar-Terr Phys 218: 105624.
- Lieth JH, Fisher PR, Heins RD, 1996. A phasic model for the analysis of sigmoid patterns of growth. Acta Hortic 417: 113-118.
- Lloyd J, Taylor JA. 1994. On the temperature dependence of soil respiration. Funct Ecol 8(3): 315-323.
- Mandel J. 1964. The statistical analysis of experimental data. New York: John Wiley & Sons. p. 58-77, 363-389.
- Moriasi DN, Armold JG, Van Liew MW, Bingner RL, Harmel RD, Veith TL. 2007. Model evaluation guidelines for systematic quantification of accuracy in watershed simulations. Trans ASABE 50(3): 885-900.
- Muro J, Strauch A, Heinamann S, Steinbach S, Thonfeld F, Waske B, Diekkruger B. 2018. Land surface temperature trends as indicator of land use changes in wetlands. Int J Appl Earth Obs 70: 62-71.
- Naseri F, Azari M, Dastorani MT. 2021. Spatial optimization of soil and water conservation practices using coupled SWAT model and evoluyionary algorithm. Int Soil Water Conserv Res 9(4): 566-577.
- Nash JE, Sutcliffe JV. 1970. River flow forecasting through conceptual models part I - A discussion of principles. J Hydrol 10(3): 282-290.
- Ning S, Song J, Ru J, Zhou Z, Zhang J, Liu X, Hui Y, Ma Y, Wan S. 2020. Nonadditive and legacy effects of spring and autumn warming on soil respiration in an old-field grassland. Ecosystems 24(2): 421-433.
- Park H, Sherstiukov AB, Fedorov AN, Polyakov LV, Walsh JE. 2014. An observation based assessment of the influences of air temperature and snow depth on soil temperature in Russia. Environ Res Lett 9(6): 064026.
- Pham TA, Hashemi A, Sutman M, Medero GM. 2023. Effect of temperature on the soil-water retention characteristics in unsaturated soils: Analytical and experimental approaches. Soils Found 63(3): 101301.
- Pham TA, Sutman M. 2023. A simplified method for bearing capacity analysis of energy piles integrating temperature-dependent model of soil-water characteristic curve. J Geotech Geo-environ Eng 149(9): 04023080.
- Seneviratne SI, Corti T, Davin EL, Hirschi M, Jaeger EB, Lehner I, Orlowsky B, Teuling AJ. 2010. Investigating soil moisture-climate interactions in a changing climate: A review. Earth-Sci Rev 99(3-4): 125-161.
- Shein EV, Dembovetsky AV, Panina SS. 2013. Modeling soil water movement under low head ponding and gravity infiltration using data determined with different methods. Proc Environ Sci 19: 553-557.
- Smith SL, Romanovsky VE, Lewkowicz AG, Burn CR, Allard M, Clow GD, Yoshikawa K, Throop J. 2010. Thermal state of permafrost in North America: A contribution to the international polar year. Permafr Periglac Process 21(2): 117-135.
- Thota SK, Vahedifard F. 2021. Stability analysis of unsaturated slopes under elevated temperatures. Eng Geol 293: 106317.
- Urqueta H, Jodal J, Herrera C, Wilke HG, Medina A, Urrutia J, Custodio E, Rodriguez J. 2018. Land surface temperature as an indicator of the unsaturated zone thickness: A remote sensing approach in the Atacama Desert. Sci Total Environ 612: 1234-1248.
- Wang XC, Li J. 2010. Evaluation of crop yield and soil water estimates using the epic model for the loess plateau of China. Math Comput Model 51(11-12): 1390-1397.
- Watson J, Challinor A. 2013. The relative importance of rainfall, temperature and yield data for a regional-scale crop model. Agric For Meteorol 170: 47-57.
- Yin X, Goudriaan J, Lantinga EA, Vos J, Spiertz HJ. 2003. A flexible sigmoid function of determinate growth. Ann Bot 91(3): 361-371.
- Zhang T, Huang JC, Lei Q, Liang X, Lindsey S, Luo J, Zhu AX, Bao W, Liu H. 2021. Empirical estimation of soil temperature and its controlling factors in Australia: Implication for interaction between geographic setting and air temperature. Catena 208: 105696.