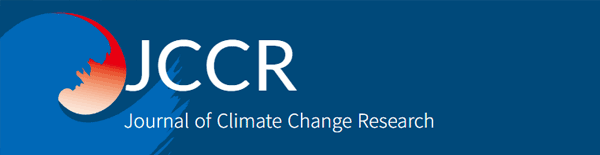
Examining the impact of climate change on green innovation: Empirical evidence from 55 cities along the southeast coast of China
Abstract
Due to global climate change, China is facing severe climate challenges, characterized by extreme high temperatures, urban floods, and intensified heat island effects. These factors pose a serious threat to the economic development of Chinese cities and hinder their resilience. This article studies 55 cities along the southeast coast of China to explore the impact of climate change on green innovation. The purpose is to evaluate the mechanisms by which climate change affects green innovation and explore policies and practices to promote green innovation. We collect a balanced panel and estimate the data from 2009 to 2019 using the System Generalized Method of Moments (SYS-GMM). The study also introduces climate variability as an independent variable to investigate the impact of abnormal temperatures and precipitation on green innovation. The benchmark results indicate that climate change can drive green innovation and maintain robustness across various dependent variables. Under the specific context where precipitation exceeds the national average along the southeast coast of China, further investigation shows that both precipitation and abnormal precipitation significantly affect green innovation. Meanwhile, among the temperature variables, only abnormal high temperatures have a positive impact on green innovation. In the heterogeneity test, abnormal high temperatures have a stimulating effect on green innovation in cities with a larger manufacturing sector, while cities with a larger service sector experience a restraining effect. Abnormal precipitation has no significant effect on these two industries. This analysis reveals the multifaceted interactions among climate change, industrial composition, and green innovation in sample cities. Overall, the research findings provide a reference for policymakers to promote green innovation and alleviate greenhouse gas emissions while facing abnormal weather and urban industrial structural transformation.
Keywords:
Climate Change, Climate Variability, Green Innovation, SYS-GMM, Industrial Structurel1. Introduction
Global climate change and its adverse effects are a shared concern for humanity. Human actions, notably the extensive use of energy by industrialized countries during the industrial revolution, have led to a surge in greenhouse gas concentration. According to the Sixth Assessment Report from the Intergovernmental Panel on Climate Change (IPCC, 2022), human activities, particularly the combustion of fossil fuels and alterations in land use, have played a significant role in global warming for over a century. These activities resulted in the current average temperature being 1.1°C higher than that observed during the pre-industrial era. Simultaneously, climate change, being a global phenomenon, has had profound impacts on urban life (Dulal and Akbar, 2013). Elevated GHG levels contribute to the rise in global temperatures, intensifying the occurrence of extreme weather events like floods, droughts, and storms while broadening the spread of tropical diseases. These ramifications place substantial financial burdens on essential urban services, infrastructure, housing, human welfare, and health (Bell et al., 2017).
In fact, some developed countries have recognized climate change as a serious challenge to national security and incorporated the impacts of climate change into their strategic frameworks. In 2010, the Obama administration underscored the undeniable, pressing, and critical nature of climate change within the United States' national security strategy. It emphasized the possible repercussions of climate change on regional security and the welfare of the public, meanwhile, highlighting the imperative to implement effective measures. Likewise, the Korean government launched a series of initiatives to address climate change. In 2008, it charted a new path for national development by embracing "low-carbon and green" principles. Later on, in 2010, it enacted the Basic Act on Low-Carbon and Green Growth. While Japanese national security strategy of 2013 similarly recognized climate change as a key issue affecting national security. In 2015, under the guidance of the United Nations, 195 parties unanimously agreed to adopt the Climate Accord, the world's first comprehensive climate change agreement, and established a key goal: to limit the rise in global average temperature to less than 2°C compared to the pre-industrial era. Additionally, the accord embraced an even more ambitious aspiration, striving to constrain the temperature increase to less than 1.5°C and prevent more serious consequences. China had become the world’s largest carbon emitter since 2006. It produced 10.67×109 t of CO2 in 2020 which accounted for 30.65% of the global total (Ritchie et al., 2020). In response to increasing environmental and public opinion pressures, the Chinese government took proactive measures and made substantial commitments to reduce emissions. Back in 2007, China unveiled its National Plan for Addressing Climate Change, which laid out ambitious targets to combat climate change by 2010. In 2022, as outlined in “China's National Emission Reduction Achievements, New Targets, and New Measures” submitted to the United Nations Framework Convention on Climate Change (UNFCCC), China made noteworthy pledges. It has embarked on a significant environmental mission with two pivotal objectives: firstly, to attain a peak in CO2 emissions by 2030, and secondly, to realize carbon neutrality by 2060. By 2030, China is resolutely committed to diminishing carbon dioxide emissions per unit of gross domestic product (GDP) by more than 65% in comparison to the levels recorded in 2005. Furthermore, China had a strategic plan to augment the share of non-fossil energy sources in their primary energy consumption to roughly 25%. These announcements underscored China's unwavering dedication to combat climate change and transition towards a more sustainable and nature-conscious energy landscape.
Although there have been many carbon emission commitments and greenhouse gas policies, a more comprehensive approach is necessary to achieve the stated climate goals. Countries must address greenhouse gas emissions at the level of energy consumption and production, promote green innovation, accelerate the transition to sustainable practices and strengthen green and low-carbon economic development system (Chen et al., 2022). Compared with traditional modes of technological innovation, green technological innovation is more oriented towards low-carbon development and has a significant inhibitory effect on carbon emissions, providing key support to countries around the world in addressing climate change and becoming the key to solve the environmental pollution problem (Gao et al., 2018). It is worth noting that some researchers emphasized the continuous growth of climate change related technologies as evidenced by the rising number of patents since the 1970s (Su and Moaniba, 2017). Consequently, a large body of literature has examined the impact of green innovation on climate change, with a particular focus on greenhouse gas emissions.
Conversely, the impact of climate change on green innovation remains a limited area, with research findings only beginning to emerge in recent years. Su and Moaniba (2017) were pioneers in reframing the literature findings into a more specific question: whether emission changes influence the development of environmentally related technologies. Instead of focusing on the conventional concept of “green technology innovation leading to climate change and greenhouse gas emissions reduction”, they examined the idea of “climate change as a catalyst for greenhouse gas emissions and green technology innovation”. Climate change is influenced by complicated environmental factors. The GHGs, like CO2, hold considerable significance. Therefore, numerous scholars utilize them as direct indicators of climate change, often overlooking climate variability and failing to account for the impact of climate uncertainty or extreme weather conditions on green innovation. Unfortunately, most existing literature uses national level samples and data to study the impact of climate change on green innovation, with a greater focus on developed countries. There are few articles in this sub research field that specifically focus on China, a major greenhouse gas emitter. However, this article uses data from prefecture level cities along the southeastern coast of China to supplement the research on urban level climate change on green innovation, enriching the content of this field from a micro perspective. Secondly, this article is based on grid data of temperature and precipitation at meteorological stations in various cities in China, which is more accurate and exogenous than previous studies. Furthermore, when we introduce industrial structure as a moderating variable to test the heterogeneity impact of climate change on green innovation, the results show that there are significant variations in green innovation across cities with different industrial structures. Therefore, the study of moderating effects also enriches the impact of climate change on green innovation under marginal changes in urban industrial transformation.
The remaining part of this article is structured as follows: In Section 2, we conducted an extensive literature review, provided a comprehensive overview of relevant research in this field, and proposed research hypothesis. Section 3 introduced our research design, data sources and variable definitions, as well as the empirical analysis methods used in this study. Section 4 provides a detailed discussion of the benchmark results obtained from the dynamic panel SYS-GMM model. In Section 5, we delved into the moderating effects of climate change on green technology innovation. Finally, in Section 6, we summarized our research findings, limitations, and proposed policy recommendations.
2. Literature review and research hypothesis
The definition of climate change varies across organizations. According to the IPCC (2018), Climate specifically refers to the long-term average or typical weather patterns. Climate change encompasses measurable and statistically testable alterations in regional climate states over time, including specific weather conditions in specific areas. NASA defines climate change as the average or typical variation in weather for a region or city. Climate change always involves transformations in Earth's overarching climatic conditions, which can manifest as variations in its average temperature. The predominant emphasis in the majority of climate change impact studies centers around alterations in mean climate conditions. When considering climate model outputs, these shifts in mean climate are generally more robust compared to changes in climate variability. However, a greater emphasis on changes in climate averages might be resulting in a substantial underestimation of the comprehensive impacts of climate change on both biological and human systems within the natural world. (Thornton et al., 2014). Climate variability refers to the fluctuations and deviations in temperature and precipitation patterns when measured against a long-term average, and it encompasses extreme weather events such as droughts and floods. These phenomena can effectively function as proxies for assessing the forthcoming consequences of climate change. This is particularly important because climate change projections anticipate a heightened occurrence and heightened intensity of both floods and droughts. (Dai, 2013; Hirabayashi et al., 2013; IPCC, 2014). Therefore, climate variability is slightly different from climate change, emphasizing the uncertainty of climate trends and having a significant impact on economic development, investment returns, policy formulation, and asset pricing (Barnett et al., 2022). It is an important emerging field, and it is necessary to consider the variability of climate when studying climate change. In addition, the uncertainty of this temperature level may lead to greater income variability and uncertain investment returns in the natural environment (Linsenmeier et al., 2022).
In addition, as early as 2003, scholars analyzed the relationship between climate change and green technology innovation, covering research on the relationship between innovation and greenhouse gas reduction. The research results emphasized the role of induced innovation (driven by environmental challenges) in reducing greenhouse gas emissions and achieving climate stability, laying the foundation for research on environmental change and green innovation (Jaffe et al., 2003). Afterwards, a large amount of academic research emerged in this field, with particular attention directed toward the intersection of environmental change and green innovation, measured from the perspective of environmental quality or innovation efficiency. Some Chinese researchers, using various green innovation indicators, have confirmed its role in reducing CO2 emissions (Lin and Zhu, 2019; Zhang and Xu, 2017). Subsequent researchers, building upon this foundation, divided China into different regions and affirmed that the eastern region exhibits the highest efficiency in green innovation and the strongest capacity to curb carbon emissions (Cai et al., 2021; Long et al., 2020).
All the above studies have examined the impact of green innovation on climate change, and there is relatively little discussion in the academic community on how climate change affects green innovation. The first possible mechanism is that climate change hinders a country's green innovation by reducing economic growth levels. More specifically, Dell with his colleagues examined the relationship between weather fluctuations and economic growth in the global dimension (Dell et al., 2012). Using large-scale cross-country data from 125 countries spanning 1950 to 2005 and data on surface temperature and rainfall for empirical analysis, they found that rainfall has no influence on the economy, while temperature has a significant impact on the national economy, but the related effects are different. There are significant differences across countries. For a per Celsius increase in temperature, the growth rate of national income in poor countries fell by 1.4 %, while economic growth in rich countries was barely affected. Using national level data, some scholars used a dynamic panel SYS-GMM model to conduct collective analysis on samples from 60 countries (including 36 developed countries and 24 developing countries) between 2008 and 2014. The results indicated that the influence of climate change on green innovation is not significant across all countries, only in developed countries it showed significantly positive (Hakimi and Inglesi-Lotz, 2020). Some researchers in the field of urban planning have used extreme weather to demonstrate that climate change can accelerate funding for sustainable urban design and innovation to enhance urban resilience (Liu et al., 2021). On the contrary, others hold different opinions, believing that there is a negative correlation between extreme temperatures and green innovation, with a more significant impact on green invention and innovation (Hu et al., 2022). As is well known, the view that economic growth can effectively address climate change and promote green innovation has been confirmed by many scholars (Wen et al., 2022; Yang et al., 2022). Therefore, from a theoretical perspective, we can propose our first hypothesis.
- H1: Climate change has an impact on green innovation
Secondly, there is a close relationship between industrial structure and green innovation, and the transformation and evolution of industrial structure have a significant impact on the development of green innovation (Mehmood et al., 2024). The intelligentization of manufacturing industry is conducive to generating "technology promotion effect" and "cost reduction effect", thereby promoting green technology innovation, effectively increasing ideal output, and significantly reducing adverse output. Especially in the eastern region of China, the effect is more significant (Yang et al., 2022). However, the service industry in China faces loose environmental regulations, thus lacking the driving force for green innovation. Also, the service industry is mainly based on human capital, and its relationship with green innovation may not be significant (Yang and Luo, 2023). Further research has found that industrial structure upgrading has spatial spillover effects and is an important way to accelerate urban green innovation and achieve sustainable development. The rationalization of industrial structure has significant positive effects on the direct, indirect, and overall effects of green innovation (Qiu et al., 2023). Based on the above literature analysis, we can propose a second hypothesis.
- H2: Climate change will have significant differences in its impact on green innovation due to different industrial structures.
3. Research design and methodology
In this section, we outline the sample and empirical research methodologies employed in this study.
3.1. Sample
In this study, we examine the relationship between climate change and green innovation in 55 cities located in the provinces of Jiangsu, Zhejiang, Fujian, Guangdong, and the municipality city Shanghai. The cities included in the sample are limited to administrative units of prefecture-level cities and above in China to ensure the accuracy and availability of data. To study the impact of climate change on green innovation, we use annual data on green invention patent applications as proxies for assessing green innovation and also use an alternative dependent variable green utility patent applications to do the robustness test. In China, patents for green inventions typically exhibit a higher degree of innovation quality and technological sophistication compared to patents for green utility, however, both can be reliable indicators of green technological innovation and are widely used in green innovation research.
As we mention before, this study considers temperature and precipitation anomalies as variables to explain climate variability. Temperature anomalies and precipitation anomalies are calculated as deviations from the spatial mean and then divided by the standard deviation within each region, following the method of previous study (Dell et al., 2014). Based on the fundamental model specification, we have also obtained inspiration from latest research and updated the details of the equation, which is more suitable for our research. The first part—the difference in mean—is already captured in a broad sense by the panel model. The second part—scaling by the standard deviation—takes a particular view of the underlying climate–economy model where level changes matter not in an absolute sense but in proportion to an area’s usual variation (Ouyang et al., 2023). Mean temperature and precipitation data for each city are obtained from the China National Scientific Data Sharing Service Platform-China Surface Climate Data Daily Values Dataset (v3.0), and the Barnes method are used for data processing. The Barnes method involves the initial use of IDW square interpolation to form grid point data covering a 500 × 500 grid in China, with a grid size of 0.12319424 longitude × 0.0994549 latitude and then partitioned and averaged for calculation.
Temperature and Precipitation Anomalies Formula:
(1) |
(2) |
AvgTEMPi,t (AvgPRECi,t) means the average temperature(precipitation) of city i in year t, AvgTEMP2009-2019 (AvgPREC2009-2019) is the average temperature (precipitation) of city i from 2009 to 2019, and StdvTEMP2009-2019 (StdvPREC2009-2019) is the standard deviation of the temperature for city i from 2009 to 2019. Table 1 Provides a summary of the variable definitions and sources utilized within this study. For the green patent applications, GDP, population and FDI, we all use nature logarithm number, in order to compress the amplitude range of data, make it easier to process, improve the normality of data, and make it more in line with standard distribution assumptions such as Gaussian distribution.
3.2. Econometric approach
The dynamic panel data model refers to a model that reflects the dynamic lag effect by introducing lagged dependent variables into the static panel data model (Blundell and Bond, 1998). The uniqueness of this model lies in the correlation between the dynamic lag term of the dependent variable and individual effects in the components of random errors, resulting in endogeneity in the estimation. Therefore, in the dynamic panel model, past observable values are allowed to be used, taking into account the impact of past results on current results. In fact, many economic problems are essentially dynamic, and some economic models indicate that current behavior depends on past behavior. For example, GDP growth and patent data growth. The SYS-GMM model is an effective dynamic panel data analysis method that can overcome endogeneity and consistency issues in panel data, improve the accuracy and efficiency of parameter estimation, and is therefore widely used in empirical research in fields such as economics and finance (Judson and Owen, 1999). In general, researchers can use SYS-GMM model in empirical studies when the variables are not strictly exogenous. In this study, both temperature and precipitation are considered to be strictly exogenous variables. Therefore, our temperature anomalies and precipitation anomalies are also strictly exogenous. This condition makes the applicability of the SYS-GMM model to this study questionable. However, in addition to mitigating endogeneity concerns, SYS-GMM model serves as a vital tool for analyzing our dynamic panel data, thereby yielding more robust and consistent estimates. In the recent research paper, temperature not only appears as an independent variable in the SYS-GMM model but also serves as a dependent variable (Deng et al., 2023). Therefore, a series of independent variables, such as temperature and precipitation, can be appropriately applied to the SYS-GMM in this study. Besides, our study selects a 10-year dataset from 2009 to 2019 and uses the sample of 55 cities, following the requirements of the SYS-GMM model “small T, large N” panel data proposed by Roodman (2009a). The estimation of dynamic panel GMM models can be categorized into either one or two step estimation, contingent on the weight matrix employed. Bond (2002) contends that the standard errors of two-step GMM estimates display a notable downward bias in the finite samples. Roodman (2009b) highlights that, with an escalating number of periods, the SYS-GMM generates a substantial number of instrumental variables by default, potentially surpassing the endogenous variables and compromising the model's diagnostic tests. In light of these considerations, we lean towards adopting a two-step system GMM approach for model estimation.
The theoretical underpinning of the GMM estimator consistency hinges on the fulfillment of various assumptions, which need two essential assessments. The first examination involves the validity of instrumental variables, evaluated through Hansen’s (1982) over-identification constraints test, which presumes the absence of a correlation between all instrumental variables and error terms. Subsequently, the second test scrutinizes the second-order serial correlation in the stochastic error terms of the difference equation by employing the Arellano-Bond test for autocorrelation in random error terms (Arellano and Bond, 1991). The original null hypothesis for this test posits the absence of second-order serial correlation in the random error terms of first-order difference equations. The non-rejection of this original hypothesis indicates the validity of the instrumental variables and correct specification of the model. Based on these theoretical mechanisms, this study constructs the following formulas:
(3) |
(4) |
The dependent variable, lnIPATi,t (lnUPATi,t) is quantified as the natural logarithm of green invention patent applications (green utility patent applications), lnIPATi,t-1 (lnUPATi,t-1) denotes the lagged variable of the dependent variable. x1i,t represents the level of climate change and climate variability in city i in year t which include temperature, precipitation, temperature anomalies, and precipitation anomalies. Besides regarding climate change as the main independent variable, this study also incorporates several other variables that have direct or indirect impacts on green innovation. Based on previous research, it has been determined that foreign direct investment positively contributes to the advancement of green innovation in China (Luo et al., 2021). The GDP per capita comprehensively reflects a region's level of economic development and strength, and the number of long-term residents serves as a measure of regional human capital. In fact, green innovation also has an effect on climate change and industrial transformation (Du et al., 2021), thus the causal relationship between the interaction term and the two needs to be considered. Based on the above benchmark model, this paper explores the industry heterogeneity of climate change on green innovation by introducing moderating variables. The value added of manufacturing industry (MA) in GDP and the value added of service industry (Ser) in GDP are used as the moderating variables of the mechanism test, so as to verify the mechanism of climate change's influence on green innovation. The second model of the model is shown as follow and:
(5) |
(6) |
x2i,t only includes temperature anomalies and precipitation anomalies, so x2yi,t represent TANO×MA, TANO×Ser, PANO×MA, PANO×Ser these four intersection terms. Also, Fig. 1 Clearly demonstrating the theoretical framework of the article.
4. Results of dynamic panel SYS-GMM model
4.1. Descriptive analysis and correlation
Table 2 displays descriptive statistics for all variables used in this study during the period 2009-2019, including the mean, standard deviation, maximum and minimum.
The data in Table 2 shows that the standard deviation of precipitation anomalies and temperature anomalies (PANO, TANO) of 1.002 and 1.001 are greater than their mean values of 0.000587 and 0.00108, which normally indicates that there may be extreme anomalies in the dataset if the standard deviation is greater than the mean value, and the data need to be further processed. However, in this study, precipitation anomaly and temperature anomaly (PANO, TANO) indicate the deviation of precipitation and temperature from their mean values, which are intentionally used to reflect the extreme precipitation and temperature conditions in the region, therefore do not need to further process the data. The minimum value of LnIPAT and LnUPAT are 0, because the green invention patent applications and green utility patent applications for some cities in some given years are only 1, and so their natural logarithm are 0. The standard deviation of LnGDP is the smallest among these variables because the cities selected for this study are from Zhejiang Province, Jiangsu Province, Guangdong Province, and Shanghai Municipality, which belong to the comparatively developed provinces in the eastern region of China, and the economic development of the different cities varies, but it is relatively small compared to the other regions of China. From the industrial structure data, we can see that the average added value of manufacturing industry in GDP (47.108) is only slightly higher that of service industry (44.562). It demonstrates that the overall integration of manufacturing industry and service industry in these cities is particularly good. At the same time, we also note that the maximum added value of service industry in GDP (72.90) is much larger than that of manufacturing sector (63.22), indicating that some cities have completed industrial transfer or rely on the single industrial structure. Table 3 shows the degree of correlation between each of the variable.
The correlation matrix reveals whether or not the dataset has a multicollinearity problem. A correlation coefficient greater than 0.80 or 0.9 suggests a potential multicollinearity issue. Table 3 shows that green invention patent is correlated with all independent variables, and green utility patent is correlated with all independent variables except for precipitation. And we notice that only the correlation coefficient between per capita GDP and the two dependent variables is greater than 0.8. GDP per capita is utilized as a control variable in this research. Theoretically, we can narrow our attention to the importance and correlation coefficient of the core explanatory variables, when we regard GDP per capita as the control variable, covariance is somehow not a problem. Especially, the correlation coefficients of green invention patents and green utility patents with other independent variables are all less than 0.8.
4.2. Benchmark analysis results
In this section, we conduct an analysis to assess the connection between the previously mentioned variables (temperature, precipitation, temperature anomalies, precipitation anomalies) and their influence on green innovation.
At beginning, we observe the robustness of the model from three aspects. Firstly, both regression of LnIPAT and LnUPAT show that their lagged variables are positively correlated with them and are statistically significant at the 1% level, implying that the current data on LnIPAT and LnUPAT depend on the number of previous years. Secondly, all p-values for AR1 less than 0.05, indicating the presence of first-order autocorrelation in the equations. Conversely, all p-values for AR2 were greater than 0.05, suggesting the absence of a second-order autocorrelation. Therefore, further higher-order tests are unnecessary, making these results favorable. In the end, all Hansen's test results are greater than 0.1 and therefore do not reject the original hypothesis, which suggests that the SYS-GMM instrumental variable estimates are valid and do not suffer from over-identification problem. These empirical findings enhance the credibility of our results. In F1 of Tables 4 and 5, both LnIPAT and LnUPAT are independent of temperature, so the annual average temperature greatly underestimates the combined impact of climate change on the natural world's biological and human systems. When average temperature is used as a proxy variable for climate change, its impact on green innovation is not significant (Thornton et al., 2014), which is consistent with existing research. Only some scholars have proven that for every 1°C increase in summer, green innovation decreases by 3.2%, and for every 1°C increase in winter, green innovation increases by 1.9%. Secondly, rising summer temperatures will squeeze research and development funds and consume labor, thereby suppressing green innovation (Li and Lu, 2023). In F2, although the correlation coefficients between precipitation and LnIPAT and LnUPAT are small (0.000139 and 0.0000131), they are all positively correlated with them at a significance level of 0.5%. When we jump to F4, at a significance level of 1%, precipitation anomalies are positively correlated with both LnIPAT and LnUPAT. However, in F3, temperature anomalies were only significantly correlated with LnIPAT at a 0.5% level, and there was no significant correlation between LnUPAT and temperature anomalies. Therefore, we can conclude that in the context of global warming, the increase in precipitation and extreme precipitation weather will promote more green innovation activities in the region, investing more scientific research funds and human capital to alleviate the economic losses that extreme precipitation may cause to the region. This conclusion is also in line with the spatiotemporal distribution characteristics of precipitation in China, with the largest magnitude of heavy rainfall events occurring in the southeastern part of the country. In addition, the high population density in eastern China has exacerbated the impact of extreme precipitation changes (Zhang and Zhou, 2020). Secondly, abnormal temperatures can promote green innovation, but it is first reflected in higher professional level green invention patents, which fully proves that climate change not only increases the number of green patents, but also improves the quality of green patents. It is specifically reflected in the transformation of green products, methods, or technological solutions. Economic growth, population growth, and foreign direct investment can also bring more green technology innovation. Tables 4 and 5 show that the coefficients of LnGDP and LnPOP are significantly positive, indicating that both economic growth and population growth can bring more green innovation. This may be because the faster the GDP growth and the higher the level of economic development, the more the government's willingness to support green technology research and innovation, and the greater the investment of research and development funds in green innovation (Song et al., 2019). Population, as another control variable, can measure the size of a city or its economy. The larger the coefficient, the stronger the city's ability to respond to climate change. In addition, the larger the coefficient, the stronger the innovation willingness of big cities to address climate change, and the more significant the impact of climate change (Zhang and Li, 2023). In Table 4, the coefficient of LnFDI is significantly positive, indicating that FDI has the ability to promote green innovation. However, in Table 5, LnUPAT is not correlated with LnFDI. Hence, we may posit that the rise in FDI brings about the infusion of advanced foreign technologies, primarily channeling funds towards research and development of environmentally-friendly invention patents boasting higher technological attributes. This, in turn, fosters green innovation through technological advancement.
5. Moderating effect of climate change impacts on green innovation
Based on the dynamic panel SYS-GMM model of climate change and green innovation constructed in the previous section, we will further consider the added value of manufacturing industry in GDP and service industry in GDP as moderating variables to conduct empirical analysis on the relationship between climate change and green innovation. According to the Porter's Hypothesis appropriate environmental regulation, environmental protection and pollution control can promote green technological innovation and energy efficiency, also environment and economy can be mutually reinforcing (Porter, 1991). On the basis of Pollution Paradise Hypothesis, countries or regions with lower environmental standards also have more pollution-intensive enterprises. In other words, the incentives for green technological innovation may differ depending on the level of environmental standards and governance. Accordingly, countries and regions with different shares of manufacturing and services may differ somewhat in whether they will innovate green technologies in the face of climate change and environmental pollution (Baumol and Oates, 1988).
From Table 6, it can be seen that cities with a high proportion of manufacturing will generate more green innovation in the face of climate change. The regression coefficient under the interaction between MA variables and TANO were significantly positive (0.007) and has a good level of robustness. That is to say, for every 1% increase in the added value of manufacturing industry in GDP, the impact of temperature anomalies on green technology innovation increases by 0.007. However, there is no significant correlation between PANO×MA and LnIPAT. The possible reason is that the Chinese government, in order to normalize industrial production, usually provides high-temperature subsidies to manufacturing and research institutions in the summer from June to September to cope with extreme high temperatures, indirectly improving the labor productivity and green innovation efficiency of workers. However, the Chinese government does not provide specific subsidies for abnormal precipitation weather, which makes the interaction between MA and TANO more sensitive to green innovation. On the contrary, the increase in added value in the service industry cannot drive green technology innovation. From Table 6, it can be seen that the regression coefficient under the interaction between Ser variable and TANO is significantly negative, indicating that the increase in service industry added value to GDP reduces the level of green technology innovation. This may be due to the low carbon dioxide emissions of the service industry itself, which does not require much green technology innovation (although it requires service and management innovation). Moreover, there is no strict regulation or pressure to incentivize the service industry to reduce carbon emissions.
From the Table 7, we notice that there is no significant correlation between the intersection terms and dependent variable LnUPAT. The relatively developed cities along the southeast coast more emphasize on innovation efficiency, while the green utility patents are not highly specialized, so the changes in the proportion of manufacturing and service industries have no significant impact on green utility patents. Therefore, we will not discuss them further in this article. Due to the fact that the manufacturing industry belongs to the heavy asset industry and has more production factors than the light asset industry, it has the ability and motivation to carry out green innovation, reduce production costs, and enable enterprises to expand reproduction and improve performance. At the same time, the manufacturing industry is facing stricter environmental regulations. Under the strict requirements of various energy-saving, carbon reduction, and pollution reduction policies, improving ecological environment quality, and urban heat island effect goals, manufacturing enterprises have to carry out green technology innovation and energy transformation. Therefore, under the dual effects of demand driven and policy driven, cities with a relatively high proportion of manufacturing will generate more green technology innovation in the face of climate change, especially under the abnormal high temperature weather. Cities with a high proportion of service industries have a relatively single industrial structure or have already completed their industrial transformation, are less willing or ability to innovate in a green way.
6. Conclusion, limitation and policy recommendations
6.1. Conclusion
We select panel data from 55 cities along the southeast coast of China from 2009 to 2019, construct a dynamic panel SYS-GMM model, and conduct a heterogeneity analysis based on industrial structure to explore the impact of climate change on green innovation. The research results demonstrate that: Firstly, precipitation, temperature anomalies, and precipitation anomalies as climate change indicators can all impulse green innovation to varying degrees, and after robustness test with alternative dependent variables, the results are still valid. Secondly, the transformation of industrial structure helps to improve the level of green innovation. The manufacturing industry has a positive effect on green innovation, while the service industry has a reverse relationship with green innovation. Finally, economic growth, population growth, and an increase in foreign direct investment can also bring more green innovation. The possible reasons are as follows: On the one hand, when facing the adverse effects of climate change, relatively developed coastal cities in southeast China will improve their risk response capacity and reduce losses through green innovation. On the other hand, under the increasingly stringent climate policies, these cities will leverage their geographical advantages, local finances, human resources, and foreign investment to drive green innovation, thereby maintaining urban competitiveness. Additionally, cities with different proportions of manufacturing and service industries show differences in their willingness to green innovation when facing climate change. Cities with a higher proportion of manufacturing tend to generate more green innovation, while an increase in the service industry does not necessarily stimulate or diminish the willingness for green innovation.
6.2. Limitation
Undoubtedly, this article also has many shortcomings, which are mainly reflected in the following aspects. At first, due to the difficulty in obtaining some data, the robustness of the article is difficult to further verify. For example, economic losses caused by natural disasters in prefecture level cities, heating degree of days, and green patents and temperature data from other regions in China. Secondly, article uses grid data of temperature and precipitation for reprocessing, so there may be some deviation. Finally, the article did not examine some mechanisms within the structure, such as testing the impact of climate change on green innovation based on seasons, and did not provide a deeper analysis of the relationship between high temperature subsidies and employee labor productivity.
6.3. Recommendations
Given these findings, we suggest the following recommendations to help cities better reduce greenhouse gas emissions and mitigate the profound impacts of climate change. Firstly, the southeast coastal regions of China are often affected by meteorological factors such as typhoons and monsoons, leading to frequent and abnormal precipitation as common climate changes. In response, green innovation can be directed towards intelligent rainwater management systems, green building and infrastructure, as well as green technology and data analysis. By establishing rainwater harvesting systems, designing green roofs, and employing advanced data analysis tools, we can better predict precipitation events and take corresponding measures, utilizing rainfall for urban greening, alleviating urban drainage pressures, and ultimately enhancing urban resilience. Secondly, effectively addressing climate change should begin with recognizing and understanding the mechanism of climate change. Research institutions at all levels should focus on the mechanisms of climate change, particularly on the causes of extreme weather and their associated risks. They should also contribute to providing scientific data-sharing services related to climate change and conducting research on climate risk assessment and environmental emergency management. Finally, the government should align its industrial restructuring with the needs of market entities, emphasizing the diversified development of industries. The focus should be on transitioning from traditional manufacturing to modern manufacturing, promoting the intelligent, green, and digital development of modern service industries, while maintaining overall stability in manufacturing. There should be a substantial effort to foster high-tech and strategic emerging industries, especially in the green sector, with a strong emphasis on energy conservation, emissions reduction, and low-carbon emissions. At the same time, government should promote the high-quality development of modern service industries to act as a catalyst for further green innovation in the renewable energy sector.
References
-
Arellano M, Bond S. 1991. Some tests of specification for panel data: Monte Carlo evidence and an application to employment equations. Rev Econ Stud 58(2): 277-297.
[https://doi.org/10.2307/2297968]
-
Barnett M, Brock W, Hansen LP. 2022. Climate change uncertainty spillover in the macroeconomy. NBER Macroecon Annu 36(1): 253-320.
[https://doi.org/10.1086/718668]
- Baumol WJ, Oates WE. 1988. The theory of environmental policy. Cambridge: Cambridge University Press.
-
Bell AH, Summerfield C, Morin EL, Malecek NJ, Ungerleider LG. 2017. Reply to Vinken and Vogels. Curr Biol 27(22): R1212-R1213.
[https://doi.org/10.1016/j.cub.2017.09.021]
-
Blundell R, Bond S. 1998. Initial conditions and moment restrictions in dynamic panel data models. J Econom 87(1): 115-143.
[https://doi.org/10.1016/S0304-4076(98)00009-8]
-
Bond SR. 2002. Dynamic panel data models: A guide to micro data methods and practice. Port Econ J 1: 141-162.
[https://doi.org/10.1007/s10258-002-0009-9]
-
Cai A, Zheng S, Cai LH, Yang H, Comite U. 2021. How does green technology innovation affect carbon emissions? A spatial econometric analysis of China’s provincial panel data. Front Environ Sci 9: 813811.
[https://doi.org/10.3389/fenvs.2021.813811]
-
Chen L, Msigwa G, Yang M, Osman AI, Fawzy S, Rooney DW, Yap PS. 2022. Strategies to achieve a carbon neutral society: A review. Environ Chem Lett 20: 2277-2310.
[https://doi.org/10.1007/s10311-022-01435-8]
-
Dai A. 2013. Increasing drought under global warming in observations and models. Nat Clim Change 3(1): 52-58.
[https://doi.org/10.1038/nclimate1633]
-
Dell M, Jones BF, Olken BA. 2012. Temperature shocks and economic growth: Evidence from the last half century. Am Econ J Macroecon 4(3): 66-95.
[https://doi.org/10.1257/mac.4.3.66]
-
Dell M, Jones BF, Olken BA. 2014. What do we learn from the weather? The new climate-economy literature. J Econ Lit 52(3): 740-798.
[https://doi.org/10.1257/jel.52.3.740]
-
Deng C, Guo Z, Huang X, Shen T. 2023. The dynamic nexus of fossil energy consumption, temperature and carbon emissions: Evidence from simultaneous equation model. Int J Environ Res Public Health 20(3): 2042.
[https://doi.org/10.3390/ijerph20032042]
-
Du K, Cheng Y, Yao X. 2021. Environmental regulation, green technology innovation, and industrial structure upgrading: The road to the green transformation of Chinese cities. Energy Econ 98: 105247.
[https://doi.org/10.1016/j.eneco.2021.105247]
-
Dulal HB, Akbar S. 2013. Greenhouse gas emission reduction options for cities: Finding the “Coincidence of Agendas” between local priorities and climate change mitigation objectives. Habitat Int 38: 100-105.
[https://doi.org/10.1016/j.habitatint.2012.05.001]
-
Gao P, Wang Y, Zou Y, Su X, Che X, Yang X. 2022. Green technology innovation and carbon emissions nexus in China: Does industrial structure upgrading matter? Front Psychol 13: 951172.
[https://doi.org/10.3389/fpsyg.2022.951172]
-
Hakimi A, Inglesi-Lotz R. 2020. Examining the differences in the impact of climate change on innovation between developed and developing countries: Evidence from a panel system GMM analysis. Appl Econ 52(22): 2353-2365.
[https://doi.org/10.1080/00036846.2019.1690126]
-
Hansen LP. 1982. Large sample properties of generalized method of moments estimators. Econometrica 50(4): 1029-1054.
[https://doi.org/10.2307/1912775]
-
Hirabayashi Y, Mahendran R, Koirala S, Konoshima L, Yamazaki D, Watanabe S, Kim H, Kanae S. 2013. Global flood risk under climate change. Nat Clim Change 3: 816-821.
[https://doi.org/10.1038/nclimate1911]
-
Hu H, Wei W, Chang CP. 2022. Examining the impact of extreme temperature on green innovation in China: Evidence from city-level data. Energy Econ 114: 106326.
[https://doi.org/10.1016/j.eneco.2022.106326]
- IPCC (Intergovernmental Panel on Climate Change). 2014. Climate change 2014: Impacts, adaptation, and vulnerability. Part B: Regional aspects. Cambridge, UK and New York, NY: Cambridge University Press.
-
IPCC (Intergovernmental Panel on Climate Change). 2018. Annex I: Glossary. In: Masson-Delmotte V, Zhai P, Pörtner HO, Roberts D, Skea J, Shukla PR, Pirani A, Moufouma-Okia W, Péan C, Pidcock R, Connors S, Matthews JBR, Chen Y, Zhou X, Gomis MI, Lonnoy E, Maycock T, Tignor M, Waterfield T (eds). Global warming of 1.5°C. An IPCC Special Report on the impacts of global warming of 1.5°C above pre-industrial levels and related global greenhouse gas emission pathways, in the context of strengthening the global response to the threat of climate change, sustainable development, and efforts to eradicate poverty. Cambridge, UK and New York, NY: Cambridge University Press. p. 541-562.
[https://doi.org/10.1017/9781009157940.008]
- IPCC (Intergovernmental Panel on Climate Change). 2022. Climate change 2022: Mitigation of climate change. Geneva: IPCC. Working group III contribution to the sixth assessment report of the Intergovernmental Panel on Climate Change.
- Jaffe AB, Newell RG, Stavins RN. 2003. Technological change and the environment. In: Mäler KG, Vincent JR (eds). Handbook of environmental economics, vol. 1. Amsterdam: Elsevier, p. 461-516.
-
Judson RA, Owen AL. 1999. Estimating dynamic panel data models: A guide for macroeconomists. Econ Lett 65(1): 9-15.
[https://doi.org/10.1016/S0165-1765(99)00130-5]
-
Li H, Lu J. 2023. Temperature change and industrial green innovation: Cost increasing or responsibility forcing? J Environ Manag 325: 116492.
[https://doi.org/10.1016/j.jenvman.2022.116492]
-
Lin B, Zhu J. 2019. The role of renewable energy technological innovation on climate change: Empirical evidence from China. Sci Total Environ 659: 1505-1512.
[https://doi.org/10.1016/j.scitotenv.2018.12.449]
-
Linsenmeier M, Mohommad A, Schwerhoff G. 2022. Policy sequencing towards carbon pricing among the world’s largest emitters. Nat Clim Change 12: 1107-1110.
[https://doi.org/10.1038/s41558-022-01538-8]
-
Liu L, Luo Y, Pei J, Wang H, Li J, Li Y. 2021. Temporal and spatial differentiation in urban resilience and its influencing factors in Henan province. Sustainability 13(22): 12460.
[https://doi.org/10.3390/su132212460]
-
Long X, Sun C, Wu C, Chen B, Boateng KA. 2020. Green innovation efficiency across China’s 30 provinces: Estimate, comparison, and convergence. Mitig Adapt Strateg Glob Change 25: 1243-1260.
[https://doi.org/10.1007/s11027-019-09903-3]
-
Luo Y, Salman M, Lu Z. 2021. Heterogeneous impacts of environmental regulations and foreign direct investment on green innovation across different regions in China. Sci Total Environ 759: 143744.
[https://doi.org/10.1016/j.scitotenv.2020.143744]
-
Mehmood S, Zaman K, Khan S, Ali Z, Khan HUR. 2024. The role of green industrial transformation in mitigating carbon emissions: Exploring the channels of technological innovation and environmental regulation. Energy Built Environ 5(3): 464-479.
[https://doi.org/10.1016/j.enbenv.2023.03.001]
-
Ouyang Y, Sun C, Wei X, Xie C. 2023. Will temperature changes in the host country reduce the inflow of international direct investment? Micro evidence from Chinese listed companies. Environ Resour Econ 86(4): 781-806.
[https://doi.org/10.1007/s10640-023-00812-3]
-
Porter ME. 1991. Towards a dynamic theory of strategy. Strateg Manag J 12(S2): 95-117.
[https://doi.org/10.1002/smj.4250121008]
-
Qiu Y, Wang H, Wu J. 2023. Impact of industrial structure upgrading on green innovation: Evidence from Chinese cities. Environ Sci Pollut Res 30(2): 3887-3900.
[https://doi.org/10.1007/s11356-022-22162-1]
- Ritchie H, Rosado P, Roser M. 2020. CO₂ and greenhouse gas emissions. Our World In Data; https://ourworldindata.org/co2-and-greenhouse-gas-emissions
-
Roodman D. 2009a. A note on the theme of too many instruments. Oxf Bull Econ Stat 71(1): 135-158.
[https://doi.org/10.1111/j.1468-0084.2008.00542.x]
-
Roodman D. 2009b. How to do xtabond2: An introduction to difference and system GMM in stata. Stata J 9(1): 86-136.
[https://doi.org/10.1177/1536867X0900900106]
-
Song X, Zhou Y, Jia W. 2019. How do economic openness and R&D investment affect green economic growth? - Evidence from China. Resour Conserv Recycl 146: 405-415.
[https://doi.org/10.1016/j.resconrec.2019.03.050]
-
Su HN, Moaniba IM. 2017. Does innovation respond to climate change? Empirical evidence from patents and greenhouse gas emissions. Technol Forecast Soc Change 122: 49-62.
[https://doi.org/10.1016/j.techfore.2017.04.017]
-
Thornton PK, Ericksen PJ, Herrero M, Challinor, AJ. 2014. Climate variability and vulnerability to climate change: A review. Glob Change Biol 20(11): 3313-3328.
[https://doi.org/10.1111/gcb.12581]
-
Wen J, Li L, Zhao X, Jiao C, Li W. 2022. How government size expansion can affect green innovation - An empirical analysis of data on cross-country green patent filings. Int J Environ Res Public Health 19(12): 7328.
[https://doi.org/10.3390/ijerph19127328]
-
Yang HC, Feng GF, Zhao XX, Chang CP. 2022. The impacts of energy insecurity on green innovation: A multi-country study. Econ Anal Policy 74: 139-154.
[https://doi.org/10.1016/j.eap.2022.01.017]
- Yang QC, Luo LW. 2023. Impact of climate change on green technology innovation - Evidence from 81 developing countries. China Soft Sci 1: 156-170.
-
Zhang J, Li S. 2023. The impact of human capital on green technology innovation - Moderating role of environmental regulations. Int J Environ Res Public Health 20(6): 4803.
[https://doi.org/10.3390/ijerph20064803]
-
Zhang W, Xu H. 2017. Effects of land urbanization and land finance on carbon emissions: A panel data analysis for Chinese provinces. Land Use Policy 63: 493-500.
[https://doi.org/10.1016/j.landusepol.2017.02.006]
-
Zhang W, Zhou T. 2020. Increasing impacts from extreme precipitation on population over China with global warming. Sci Bull 65(3): 243-252.
[https://doi.org/10.1016/j.scib.2019.12.002]