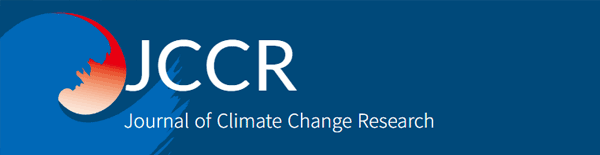
Nonlinear effects of temperature on electricity demand: Identifying endogenous temperature thresholds
Abstract
This study examines the nonlinear effects of temperature on electricity demand in the residential and general services sectors. Using a panel threshold fixed-effects model, it identifies temperature thresholds that define distinct consumption regimes: 19.76°C and 24.10°C for residential service, and 3.61°C and 19.73°C for general service. The second threshold, which marks a turning point for heating and cooling demand, is lower for general service than for residential service. This reflects distinct space-use patterns that necessitate earlier cooling in commercial environments. Seasonal analyses reveal distinct thresholds: 24.10°C and 27.54°C for residential service and 25.66°C for general service in summer, and -3.02°C for residential service with -1.7°C and -2.61°C for general service in winter. During summer, electricity consumption for both types increases across all temperature regimes due to higher cooling demand, while in winter, consumption decreases as rising temperatures reduce heating demand. This study contributes to the literature by investigating both residential and general electricity services, which are both closely related to daily life, and by estimating endogenous temperature thresholds. The findings provide empirical evidence for policymakers in the energy sector to develop sustainable demand-side management strategies. While this study exploits monthly data, future research should leverage micro-level data (e.g., daily or hourly) to further elucidate the relationship between temperature and electricity consumption, offering more detailed insights for sustainable energy policy.
Keywords:
Electricity Consumption, Temperature Thresholds, Nonlinear Effects, Energy Policy, Panel Threshold Fixed-Effects Model1. Introduction
Electricity demand has increased with urbanization and electrification (Cao et al., 2023; Santamouris et al., 2015; G. Zhang et al., 2023; M. Zhang et al., 2021). This demand is shaped by a variety of factors, including economic activity, population growth, and climatic conditions. Among these, temperature stands out as a critical determinant, as it drives the need to regulate indoor environments in response to ambient temperature. This dynamic has become increasingly significant in the context of the climate crisis, which intensifies the variability of weather phenomena. South Korea’s heavy reliance on fossil fuel-based power generation underscores the critical need for effective electricity demand management. As the challenges posed by the climate crisis intensify, managing electricity demand plays a pivotal role in fostering sustainable development and mitigating environmental impacts.
To analyze electricity demand for heating and cooling purposes, it is essential to focus on the electricity consumption of buildings where daily human activities occur. In South Korea, electricity services are categorized into six distinct sectors: residential, general, industrial, educational, agricultural, and street lighting. This study primarily investigates residential and general electricity consumption1). Residential electricity service is provided to various types of housing, including apartments, dormitory-style residences for unmarried individuals, and group home facilities for social welfare purposes. General service refers to electricity consumption by customers who are not classified into any of the five other service categories and is primarily used in stores and offices.
Given their close connection to daily life, these two consumption categories are expected to exhibit unique patterns of variation in response to temperature changes. The existing literature predominantly focuses on residential electricity consumption and often relies on exogenously determined threshold temperatures for analysis. This study analyzes both residential and general electricity consumption, allowing for the endogenous determination of threshold temperatures within the model. The primary objective of this research is to identify temperature thresholds that adjust the effects of regime-dependent variables closely linked to daily activities on electricity consumption.
This study employs a panel threshold fixed-effects model to endogenously estimate temperature thresholds for electricity demand in South Korea, using panel data from 2013 to 2022. By examining the nonlinear temperature effects, the study provides a better understanding of the relationship between temperature and electricity consumption across the country.
This paper is organized as follows. Section 2 reviews the relevant literature, highlighting key findings and gaps that motivate this study. Section 3 provides an overview of the data sources and variables used in this study, while Section 4 details the analytical methods and modeling framework. Section 5 presents and interprets the findings, and Section 6 concludes with key implications.
2. Literature Review
Extensive research has delved into the relationship between temperature and electricity consumption, emphasizing the regional and sectoral differences (Bessec and Fouquau, 2008; Cao et al., 2023; Deschênes and Greenstone, 2011; Santamouris et al., 2015; C. Zhang et al., 2019; G. Zhang et al., 2023; M. Zhang et al., 2021). This relationship has been primarily analyzed through three main approaches: incorporating temperature as a variable in models, segmenting temperatures into exogenously defined bins, or utilizing indices such as HDD (Heating Degree Days) and CDD (Cooling Degree Days) based on predetermined thresholds. When temperature data is directly included (C. Zhang et al., 2019), arbitrarily divided into bins (Deschênes and Greenstone, 2011), or when indices like CDD and HDD are the main explanatory variables (M. Zhang et al., 2021), fixed-effects models have often been used to estimate the marginal effects of temperature on electricity consumption. These studies also employ spline functions to construct temperature-electricity response curves and analyze urban-rural inequalities using temperature bins (G. Zhang et al., 2023).
Although panel fixed-effects models effectively control for time-invariant factors and regional heterogeneity, they fall short in capturing nonlinear effects. Indices such as CDD and HDD, while useful, simplify the dynamics by using a single baseline temperature that is exogenously determined.
Previous research has consistently identified nonlinear patterns, such as V-shape, U-shape, or J-shape relationships (Bessec and Fouquau, 2008; Cao et al., 2023; Deschênes and Greenstone, 2011; Moral-Carcedo and Vicéns-Otero, 2005). For instance, Bessec and Fouquau (2008) used the Panel Smooth Transition Regression (PSTR) method to analyze U-shaped nonlinear relationships in European countries. Similarly, Moral-Carcedo and Vicéns-Otero (2005) employed Logistic Smooth Transition Regression (LSTR) to identify a U-shape relationship in Spain’s daily electricity consumption. Deschênes and Greenstone (2011) confirmed a U-shape using temperature bins and fixed-effects models, while Cao et al. (2023) applied segmented regression to determine threshold temperatures for different sectors.
In South Korea, Shin and Jo (2014) examined the relationship between temperature and electricity consumption using daily maximum electricity data. The study utilized nonlinear models such as Markov regime switching, threshold regression, and smooth transition regression to assess the cumulative temperature effect. They identified a critical temperature range of 17~23℃, with different thresholds for different models. However, they did not differentiate by service type and used national electricity consumption, which lacks spatial and service type heterogeneity.
Building upon these findings, this study employs an endogenous estimation approach to address unobserved time-invariant characteristics by employing a threshold regression model developed by Hansen (1999). The bootstrapping method for estimating multiple thresholds proposed by Wang (2015) has been implemented in Stata, which has yet to be applied to analyze temperature-electricity relationships.
Compared to exogenous temperature bin methods or predetermined thresholds approach like HDD or CDD, the panel fixed-effects threshold model has strength in identifying temperature thresholds endogenously in the model. This approach also improves upon the work of Shin and Jo (2014), which included industrial, educational, agricultural, and street lighting electricity usage without accounting for unobserved spatial and time-invariant heterogeneity. By focusing on residential and general electricity consumption and utilizing sales data from Korea Electric Power Corporation (KEPCO), this study captures cooling and heating demands directly tied to everyday life.
3. Data
This study utilizes two primary datasets from South Korea: electricity consumption categorized by service type and weather observation data. Monthly electricity consumption data were obtained from the Electric Power Data Open Portal System (2024), managed by the KEPCO. KEPCO disclosed this data including nationwide information on the number of customers, sales volume, and sales charges by region, month, and contract type. Data from regions with fewer than five customers were removed prior to discloser to protect personal information. The average number of residential electricity customers showed an increasing trend from 2013 to 2022, starting at approximately 12.6 million in 2013 and reaching 14.4 million in 2022. Similarly, the average number of general electricity customers consistently grew from around 2.5 million in 2013 to nearly 3.0 million in 2022.
Electricity consumption data was processed differently for each sector to account for variations in usage patterns. Residential electricity consumption was calculated by dividing the total monthly electricity consumption at the sigungu level by the number of customers2), reflecting its direct connection to individual customers’ daily lives. In contrast, monthly general electricity consumption at the sigungu level was divided by the sigungu’s population. This approach considers that the general electricity consumption mostly occurs in offices and stores, where the size of population is a primary determinant of electricity demand.
Weather data were sourced from the Korean Meteorological Administration (2024), including key variables such as monthly average temperature (°C), monthly average relative humidity (%), and monthly total precipitation. In this study, temperature is employed as an explanatory variable, as it is well known as a key factor influencing electricity consumption. Relative humidity and precipitation, which are closely related to temperature. are often used as control variables in the literature when analyzing the effects of temperature on residential electricity consumption (G. Zhang et al., 2023; M. Zhang et al., 2021). Accordingly, this study includes relative humidity and precipitation as control variables in the model to examine both residential and general electricity consumption. Both factors are closely associated with electricity consumption in buildings, as relative humidity affects the apparent temperature perceived by individuals, while precipitation influences people’s decisions between indoor and outdoor activities. These variables were aggregated at the sigungu level and combined with electricity consumption data for analysis.
The dataset used for the analysis was constructed by combining monthly sigungu-level customer counts and electricity sales from 2013 to 2022 with annual sigungu-level population administrative data and monthly meteorological data. Figure 1 depicts the relationship between average temperature and monthly electricity consumption across the two service types: residential (Fig. 1(a).) and general service (Fig. 1(b).).
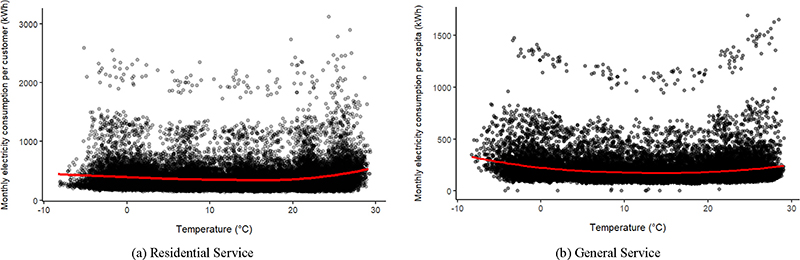
Scatter plots showing the relationship between temperature and monthly electricity consumption by service type
The scatter plots include all observed values at the sigungu levels from 2013 to 2022, capturing outliers. The top plot illustrates the relationship between monthly average temperature and monthly electricity consumption. Monthly residential electricity consumption per customer displays a weak U-shaped pattern, with usage increasing at temperatures above 20°C. For monthly general electricity consumption per capita, the U-shaped pattern is more pronounced, with consumption decreasing at temperatures below 10°C and increasing above 20°C. Despite the noise in the data, the weak U-shaped patterns observed in residential and general electricity consumption indicate the potential existence of one or more thresholds that significantly affect electricity usage.
Summary statistics are shown in Table 1. For residential service, monthly electricity consumption peaks in August at 470 kWh, corresponding to high temperature (25.7°C) and relative humidity levels (80.6%). The lowest monthly consumption occurs in May at 341kWh, during moderate temperature (17.8°C). For general service, consumption also peaks in January at 233kWh, reflecting high temperatures and relative humidity. The lowest consumption is observed in May at 167kWh, during milder weather. General service consumption shows relatively smaller seasonal variation compared with residential service.
The analysis period (2013~2022) is consistent across both service types, but the summary statistics of weather variables exhibit slight differences. This discrepancy arises due to the unequal dataset sizes: 223 sigungu with a total of 26,760 observations for residential electricity consumption and 212 sigungu with a total of 25,440 observations for general electricity consumption. Since the panel fixed-effect threshold model employed in this study requires a balanced panel for analysis3), some data were excluded to construct a balanced panel for each service type on a monthly basis throughout the analysis period.
4. Method
This study employs a panel threshold fixed-effects model to explore nonlinear relationships between weather variables and electricity consumption. Originally developed by Hansen (1999), the model estimates threshold effects in non-dynamic panel data while accounting for individual fixed effects. By minimizing the residual sum of squares, it endogenously identifies thresholds and regression coefficients, allowing for the detection of regime shifts. The analysis begins with cross-sectional dependency and unit roots tests to validate the panel model. Observations are categorized into distinct regimes based on the threshold variable, with regression coefficients varying across these regimes. When the threshold variable exceeds a certain value, the relationship between the dependent and independent variables changes, indicating a regime shift. To ensure more precise estimations, fixed-effects transformations are applied to control for unobserved individual characteristics, effectively addressing heterogeneity within the panel dataset. The basic model, assuming a single threshold, is introduced as follows:
(1) |
The subscripts i and t represent individual sigungu and year-month, respectively. Xi,t is a vector of regime-dependent independent weather variables, which includes monthly average temperature, monthly average relative humidity, and monthly total precipitation. The threshold variable, qi,t represents the monthly average temperature, and γ denotes the estimated threshold value at which regime shifts occur. I( • ) is an indicator function, where the second term on the right-hand side represents the regression coefficient when the threshold variable is smaller than the estimated threshold, and the third term represents the regression coefficient when the threshold variable exceeds the estimated threshold. The final term on the right-hand side is the error term.
The vector Xi,t must consist of time-varying variables, and the error term is assumed to satisfy the assumptions of being independently and identically distributed with a mean of zero and finite variance (Hansen, 1999). In cases where there are two thresholds, the model partitions the data into three distinct regimes. The corresponding specification for the model in such cases is specified as follows:
(2) |
When three thresholds are present, the model divides the data into four regimes, and the model specification can be expressed as follows:
(3) |
In the next section, the number of thresholds will be endogenously determined by testing for threshold effects.
5. Results
5.1. Cross-Sectional Dependency and Unit Root Testing
To analyze panel data, it is essential to assess cross-sectional dependency (Pesaran, 2021). The R package ‘plm’ was used to conduct cross-sectional dependence tests for panel models in each dataset. Table 2 reports the results of these tests. The null hypothesis assumes no cross-sectional dependence, while the alternative hypothesis indicates the presence of dependency among cross-sectional units.
The cross-sectional dependency test statistics for all types and variables are high, strongly rejecting the null hypothesis with a high level of confidence. This indicates that observations across different sigungu are interconnected, likely due to shared climatic conditions or economic factors. Consequently, cross-sectional dependency needs to be accounted for in unit root testing. Given that the datasets are panel and exhibit cross-sectional dependence, the cross-sectionally augmented IPS (CIPS) test is the appropriate method for assessing stationarity (Pesaran, 2007). The analysis was conducted using the ‘bootUR’ package in R, which applies a bootstrap-based unit root test (Smeekes and Wilms, 2023). The results are presented in Table 3.
Table 3 presents the results of the unit root tests conducted to evaluate the stationarity of variables across the residential and general services. The null hypothesis assumes that all series in the panel data contain unit roots and are therefore non-stationary, while the alternative hypothesis posits that at least some series in the panel are stationary. The test statistics and corresponding p-values strongly reject the null hypothesis, indicating that the datasets are stationary. This confirms their suitability for further econometric analysis without requiring additional transformations, such as differencing.
5.2. Testing for Threshold Effects
Threshold effect tests were conducted to determine the appropriate number of thresholds for each dataset using ‘xthreg’ package in StataSE 18. Using monthly average temperature as the threshold variable, the analysis began with the assumption of a triple-threshold model. The null hypothesis for the triple-threshold model posits that no triple-threshold effect exists, implying that a double-threshold model sufficiently explains the data. The results of the threshold effect tests are summarized in Table 4.
The thresholds effect tests for the triple-threshold models fail to reject the null hypothesis, indicating no evidence for the existence of a third threshold. The results suggest that the triple-threshold models are not statistically significant for both residential and general services. The double threshold models are supported for year-round residential and general services, residential service during summer and general service during winter. Single threshold models are supported for residential service during winter and general service during summer. Table 5 presents the threshold estimates of monthly average temperature for residential and general electricity consumption across year-round, summer, and winter seasons.
5.3. Estimation of Panel Threshold Fixed- Effects Models
Table 6 presents the regression estimates from the panel threshold fixed-effects model, detailing the impacts of monthly average temperature, monthly average relative humidity, and monthly total precipitation on electricity consumption across different temperature regimes and seasons for residential and general service types.
Year-round data indicate that electricity consumption increases beyond the second thresholds for both residential (24.10℃) and general electricity (19.73℃). During summer, residential electricity consumption rises significantly at 24.10℃ and again at 27.54℃, while general electricity consumption shows a marked increase above 25.66℃. In winter, residential electricity consumption decreases below -3.02℃, and general electricity consumption declines at two thresholds: -1.70℃ and -2.61℃. Overall, electricity consumption for both residential and general service types increase consistently across all temperature regimes, reflecting heightened cooling demand during warmer periods. Conversely, during winter electricity consumption for both types decreases across all temperature thresholds, driven by reduced heating demand as temperature rise.
Year-round data show differing patterns in the effects of relative humidity and precipitation on electricity consumption for residential and general service types. For relative humidity, residential electricity consumption shows a modest positive effect in first and second regimes but becomes significantly negative in the highest regime (above 24.10℃). In contrast, general electricity consumption exhibits a small positive effect in the lowest regime but becomes negative across all other regimes. Precipitation generally has a consistent, positive effect on residential electricity consumption, with coefficients increasing across regimes year-round. However, general electricity consumption shows mixed effects, showing negative effects in lower regimes and positive impacts in higher regimes.
6. Conclusions and Discussions
This study examines the nonlinear effects of monthly average temperature on monthly electricity consumption across residential and general sectors in South Korea. Using a panel threshold fixed-effects model, the analysis identifies temperature thresholds that define distinct electricity consumption regimes. These empirical findings demonstrate that the threshold temperatures, as well as the magnitude and signs of regression coefficients, vary depending on electricity service type and season when analyzing the impact of temperature on electricity consumption.
In the year-round analysis, the first threshold for monthly residential electricity consumption was estimated at 19.76℃, while the second threshold for monthly general electricity consumption was 19.73℃. Existing literatures have shown that when applying temperature bins in the study conducted in the U.S., the 50-60°F bin was selected as the reference level in the regression model (Deschênes and Greenstone, 2011), whereas 18℃ was commonly used as base temperature for defining low and high-temperature when employing HDD or CDD (M. Zhang et al., 2021). These findings suggest that the estimated temperature thresholds at which electricity consumption patterns change are closely aligned with the exogenous thresholds adopted in previous literature.
For both service types, a transition in demand was observed: monthly electricity consumption exhibited positive temperature effects when temperatures exceeded the second threshold, whereas negative effects were observed when temperatures were below this threshold. Therefore, the analysis suggests that using a single base temperature of 18℃ is not reliable to indicate transition from heating to cooling electricity demand for residential service. Rather, using a distinct base temperatures for CDD at 26℃, which is closer to the second threshold of 24.10℃, is more accurate as seen in the case of China (G. Zhang et al., 2023)
The second threshold for residential service was 24.10℃, while for general service, it was 19.73℃, indicating that the temperature at which electricity demand begins to increase is lower for general service than for residential service. The difference can be attributed to variations in purpose of space use by service type. Residential service applies to living spaces where individuals directly pay the electricity bills for cooling. As a result, customers may hesitate to use cooling appliances until higher temperatures to reduce the bills. In contrast, general service is primarily used in offices and commercial spaces, where a larger number of people occupy the same area, frequently use heat-generating equipment such as lighting and computers, and move in and out more frequently. These factors make it more challenging to maintain a stable indoor temperature. Consequently, cooling systems for general service are more likely to be activated at lower temperatures than for residential service to ensure a comfortable working environment and enhance convenience.
By identifying endogenous temperature thresholds and heterogeneous regimes, this study provides a deeper understanding of the conditions under which electricity consumption responds to temperature variations. The findings suggest several policy implications. First, energy pricing policies should be established based on robust empirical evidence to more effectively manage demand and promote sustainable energy consumption. For instance, residential electricity pricing in South Korea has often been influenced by political considerations rather than scientific data analysis. Second, understanding the heterogeneous effects of temperature on electricity consumption by service type provides insights for infrastructure investment and resource allocation. Third, such research has a potential to develop more sustainable energy planning strategies. This can be achieved by integrating household or customer-level socio-economic data with climate scenarios to project future energy demand.
This study uses nationwide, monthly data spanning a 10-year period at the sigungu level, providing a sufficient time scale for a panel fixed-effects model. Nevertheless, it should be noted that hourly or daily level data can better capture the effects of temperature variations on electricity demand. Given the close relationship between residential and general services and daily life, micro-level data can offer more valuable insights in establishing demand-side management strategies.
Acknowledgments
This work was supported by Korea Environment Industry & Technology Institute (KEITI) through Climate Change R&D Project for New Climate Regime., funded by Korea Ministry of Environment (MOE)(RS-2022-KE002096).
[Declaration of using Generative AI and AI-assisted technologies in the writing process]
During the preparation of this work, the author used ChatGPT-4 by OpenAI in order to review, translate, and edit the English draft of the manuscript to ensure an academic writing style.
Notes
References
-
Bessec M, Fouquau J. 2008. The non-linear link between electricity consumption and temperature in Europe: A threshold panel approach. Energy Econ 30(5): 2705-2721.
[https://doi.org/10.1016/j.eneco.2008.02.003]
-
Cao J, Zhou W, Wang W, Pan X, Jing C, Qian Y. 2023. Spatially heterogeneous effect of temperature on electricity consumption in Shenzhen, China. Build Environ 241:, 110468.
[https://doi.org/10.1016/j.buildenv.2023.110468]
-
Deschênes O, Greenstone M. 2011. Climate change, mortality, and adaptation: Evidence from annual fluctuations in weather in the US. Am Econ J 3(4): 152-185.
[https://doi.org/10.1257/app.3.4.152]
- Electric Power Data Open Portal System. 2024. [accessed 2024 Dec 17] https://bigdata.kepco.co.kr/
-
Hansen B. 1999. Threshold effects in non-dynamic panels: Estimation, testing, and inference. J Econom 93(2): 345-368.
[https://doi.org/10.1016/S0304-4076(99)00025-1]
- Korean Meteorological Administration. 2024. [accessed 2024 Dec 26] https://data.kma.go.kr/resources/html/en/aowdp.html
-
Moral-Carcedo J, Vicéns-Otero J. 2005. Modelling the non-linear response of Spanish electricity demand to temperature variations. Energy Econ 27(3): 477-494.
[https://doi.org/10.1016/j.eneco.2005.01.003]
-
Pesaran M. 2007. A simple panel unit root test in the presence of cross‐section dependence. J Appl Econom 22(2): 265-312.
[https://doi.org/10.1002/jae.951]
-
Pesaran M. 2021. General diagnostic tests for cross-sectional dependence in panels. Empir Econ 60(1): 13-50.
[https://doi.org/10.1007/s00181-020-01875-7]
-
Santamouris M, Cartalis C, Synnefa A, Kolokotsa D. 2015. On the impact of urban heat island and global warming on the power demand and electricity consumption of buildings - A review. Energy Build 98: 119-124.
[https://doi.org/10.1016/j.enbuild.2014.09.052]
- Shin D, Jo H. 2014. A empirical study on the climate factor sensitivity and threshold temperature of daily maximum electricity consumption in Korea (in Korean with English abstract). J Econ Stud 32(4): 175-212.
-
Smeekes S, Wilms I. 2023. bootUR: An R package for bootstrap unit root tests. J Stat Softw 106(12): 1-39.
[https://doi.org/10.18637/jss.v106.i12]
-
Wang Q. 2015. Fixed-effect panel threshold model using Stata. Stata J 15(1): 121-134.
[https://doi.org/10.1177/1536867X1501500108]
-
Zhang C, Liao H, Mi Z. 2019. Climate impacts: Temperature and electricity consumption. Nat Hazards 99(3): 1259-1275.
[https://doi.org/10.1007/s11069-019-03653-w]
-
Zhang G, Shen L, Su B. 2023. Temperature change and daily urban-rural residential electricity consumption in Northwestern China: Responsiveness and inequality. Energy Econ 126: 106973.
[https://doi.org/10.1016/j.eneco.2023.106973]
-
Zhang M, Chen Y, Hu W, Deng N, He W. 2021. Exploring the impact of temperature change on residential electricity consumption in China: The ‘crowding-out’ effect of income growth. Energy Build 245: 111040.
[https://doi.org/10.1016/j.enbuild.2021.111040]